Cost-Effective Approximate Aggregation Queries on Geospatial Big Data.
GLOBECOM (Workshops)(2023)
摘要
Aggregation queries are essential in spatial data analytics, including Top-N, and geo-statistics such as ‘mean’ and ‘count’. Those queries require grouping geospatial objects into pre-defined clusters that are typically administrative polygons representing study areas such as cities. Given a big georeferenced dataset on the order of millions, and a group of polygons representing a city, the aggregation query requires grouping objects by polygons and determining to which polygon each object belongs. This is a computationally expensive geospatial operation because polygons are typically represented by huge amounts of vertices. In this paper, we show the design and realization of a system that we term ApproxGeoAgg for the efficient approximation of costly geospatial aggregate queries that require group-by operations. We have performed extensive testing, and our results show that our system outperforms plain baselines by order-of-magnitude in terms of balancing running times with accuracy. Specifically, for Top-N aggregation queries we obtain tiny loss in accuracy that reaches 0.00038% depending on parameter configurations, with a corresponding gain in running time on par with 2.6%, which escalates to circa 12% as we decrease the number of polygon boundary vertices.
更多查看译文
关键词
spatial approximate query processing,geospatial group-by,spatial aggregation,Douglas Peucker,line simplification
AI 理解论文
溯源树
样例
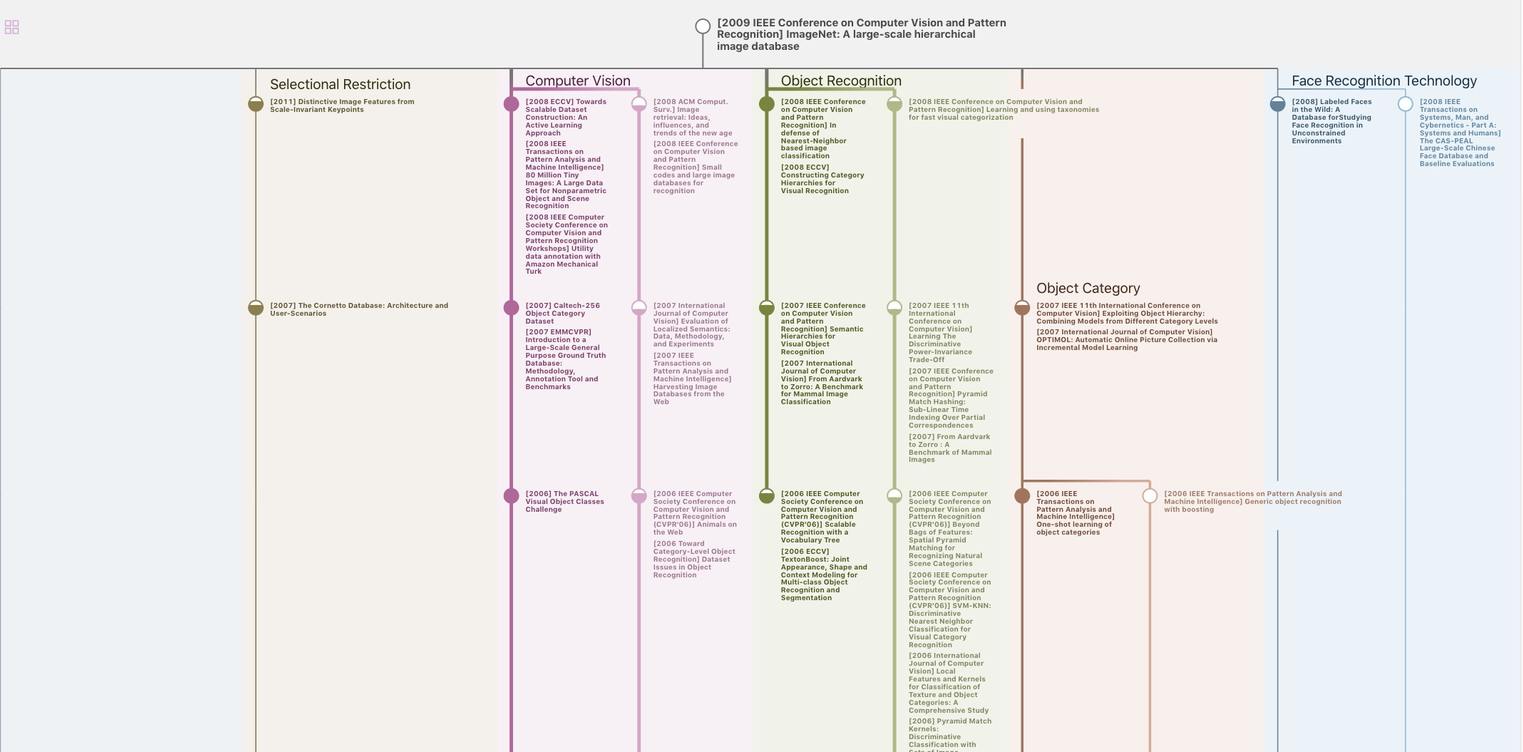
生成溯源树,研究论文发展脉络
Chat Paper
正在生成论文摘要