Causality-Invariant Interactive Mining for Cross-Modal Similarity Learning.
IEEE transactions on pattern analysis and machine intelligence(2024)
摘要
In the real world, how to effectively learn consistent similarity measurement across different modalities is essential. Most of the existing similarity learning methods cannot deal well with cross-modal data due to the modality gap and have obvious performance degeneration when applied to cross-modal data. To tackle this problem, we propose a novel cross-modal similarity learning method, called Causality-Invariant Interactive Mining (CIIM), that can effectively capture informative relationships among different samples and modalities to derive the modality-consistent feature embeddings in the unified metric space. Our CIIM tackles the modality gap from two aspects, i.e., sample-wise and feature-wise. Specifically, we start from the sample-wise view and learn the single-modality and hybrid-modality proxies for exploring the cross-modal similarity with the elaborate metric losses. In this way, sample-to-sample and sample-to-proxy correlations are both taken into consideration. Furthermore, we conduct the causal intervention to eliminate the modality bias and reconstruct the invariant causal embedding in the feature-wise aspect. To this end, we force the learned embeddings to satisfy the specific properties of our causal mechanism and derive the causality-invariant feature embeddings in the unified metric space. Extensive experiments on two cross-modality tasks demonstrate the superiority of our proposed method over the state-of-the-art methods.
更多查看译文
关键词
Similarity Learning,Cross-Modal Learning,Causal Intervention,Hard Mining
AI 理解论文
溯源树
样例
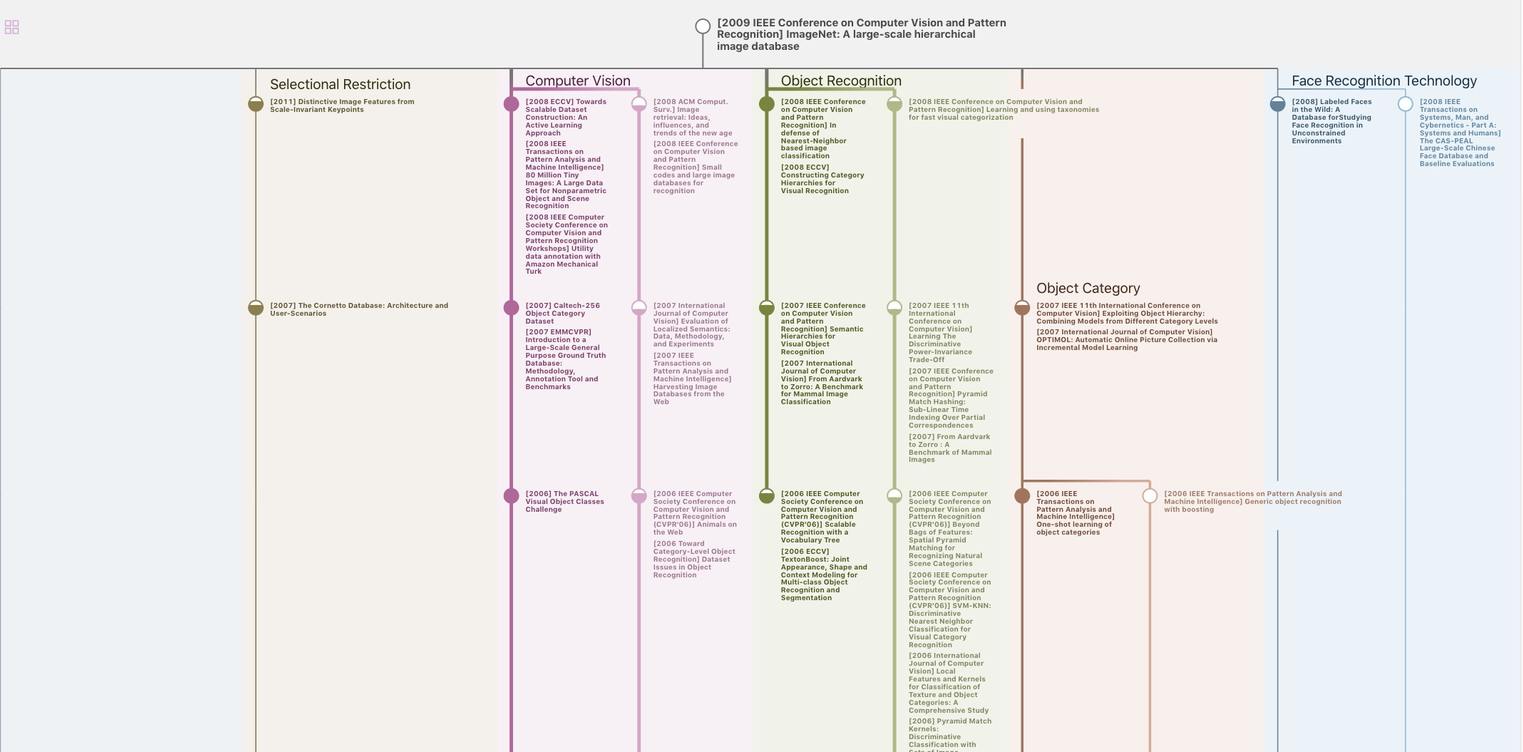
生成溯源树,研究论文发展脉络
Chat Paper
正在生成论文摘要