Chance-Constrained Abnormal Data Cleaning for Robust Classification With Noisy Labels
IEEE TRANSACTIONS ON EMERGING TOPICS IN COMPUTATIONAL INTELLIGENCE(2024)
Abstract
Supervised classification is a common field of machine learning. However, the existing classification methods based on deep models are vulnerable to overfitting the noisy labels in the training set. This paper proposes a data-cleaning method to achieve robust classification against noisy labels. A chance-constrained abnormal data cleaning approach is proposed based on chance-constrained optimization, in which a polynomial sublevel set for the data of each class is generated. The data outside the polynomial sublevel set is abnormal and has a low probability of belonging to the labeled class. The classification method only uses normal data to establish the estimated classifiers. We show the convergence of the proposed abnormal data-cleaning approach. Furthermore, we give the algorithm for classification with abnormal data cleaning. Experimental data-based validations have been implemented to validate the proposed classification algorithm. The results show that the proposed approach can correctly clean the abnormal noisy labels and improve the performance of Supervised classification methods.
MoreTranslated text
Key words
Classification with noisy labels,chance constrained optimization
AI Read Science
Must-Reading Tree
Example
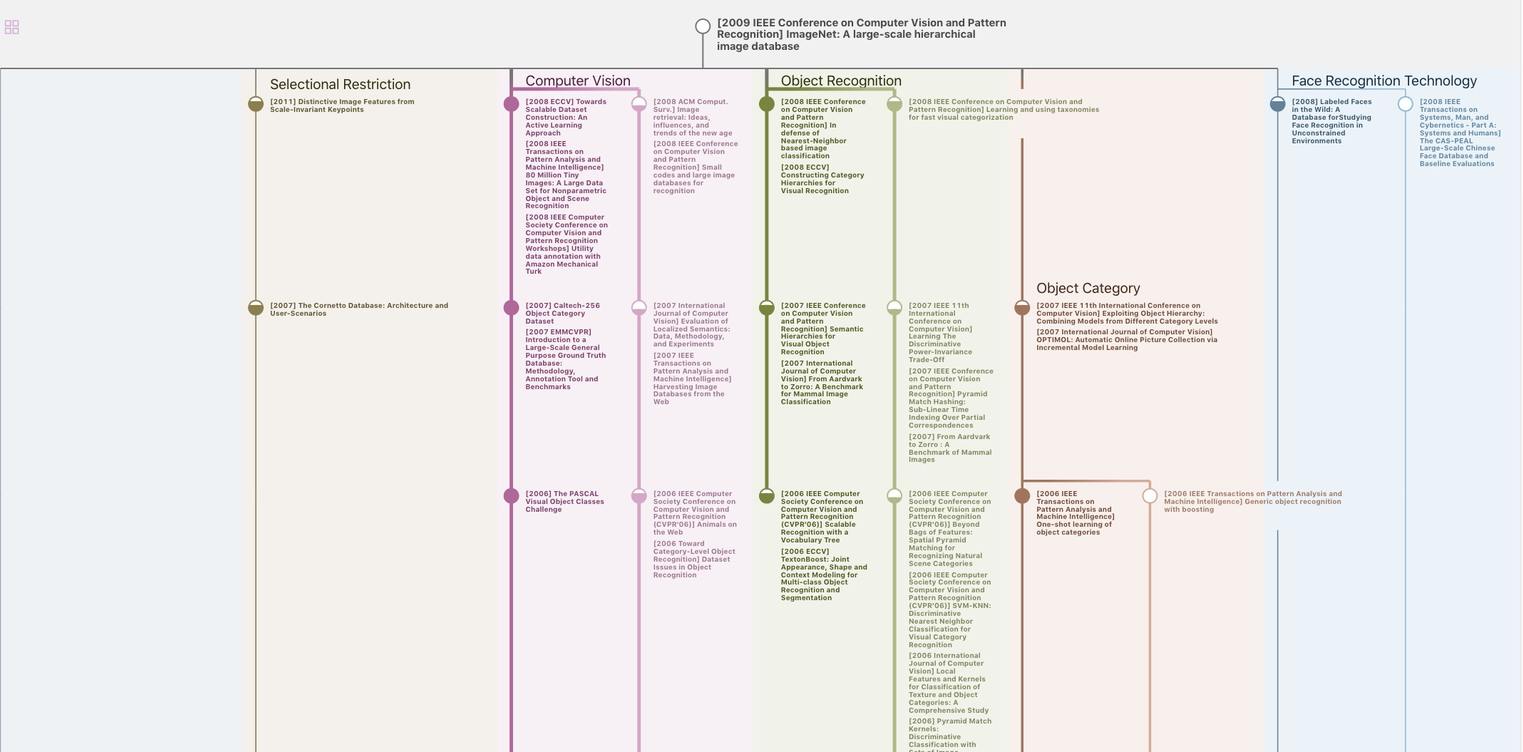
Generate MRT to find the research sequence of this paper
Chat Paper
Summary is being generated by the instructions you defined