Cross-Modal Consistency for Single-Modal MR Image Segmentation.
IEEE transactions on bio-medical engineering(2024)
摘要
OBJECTIVE:Multi-modal magnetic resonance (MR) image segmentation is an important task in disease diagnosis and treatment, but it is usually difficult to obtain multiple modalities for a single patient in clinical applications. To address these issues, a cross-modal consistency framework is proposed for a single-modal MR image segmentation.
METHODS:To enable single-modal MR image segmentation in the inference stage, a weighted cross-entropy loss and a pixel-level feature consistency loss are proposed to train the target network with the guidance of the teacher network and the auxiliary network. To fuse dual-modal MR images in the training stage, the cross-modal consistency is measured according to Dice similarity entropy loss and Dice similarity contrastive loss, so as to maximize the prediction similarity of the teacher network and the auxiliary network. To reduce the difference in image contrast between different MR images for the same organs, a contrast alignment network is proposed to align input images with different contrasts to reference images with a good contrast.
RESULTS:Comprehensive experiments have been performed on a publicly available prostate dataset and an in-house pancreas dataset to verify the effectiveness of the proposed method. Compared to state-of-the-art methods, the proposed method can achieve better segmentation.
CONCLUSION:The proposed image segmentation method can fuse dual-modal MR images in the training stage and only need one-modal MR images in the inference stage.
SIGNIFICANCE:The proposed method can be used in routine clinical occasions when only single-modal MR image with variable contrast is available for a patient.
更多查看译文
关键词
Consistency Learning,Contrast Alignment,Single-modal MR Image Segmentation
AI 理解论文
溯源树
样例
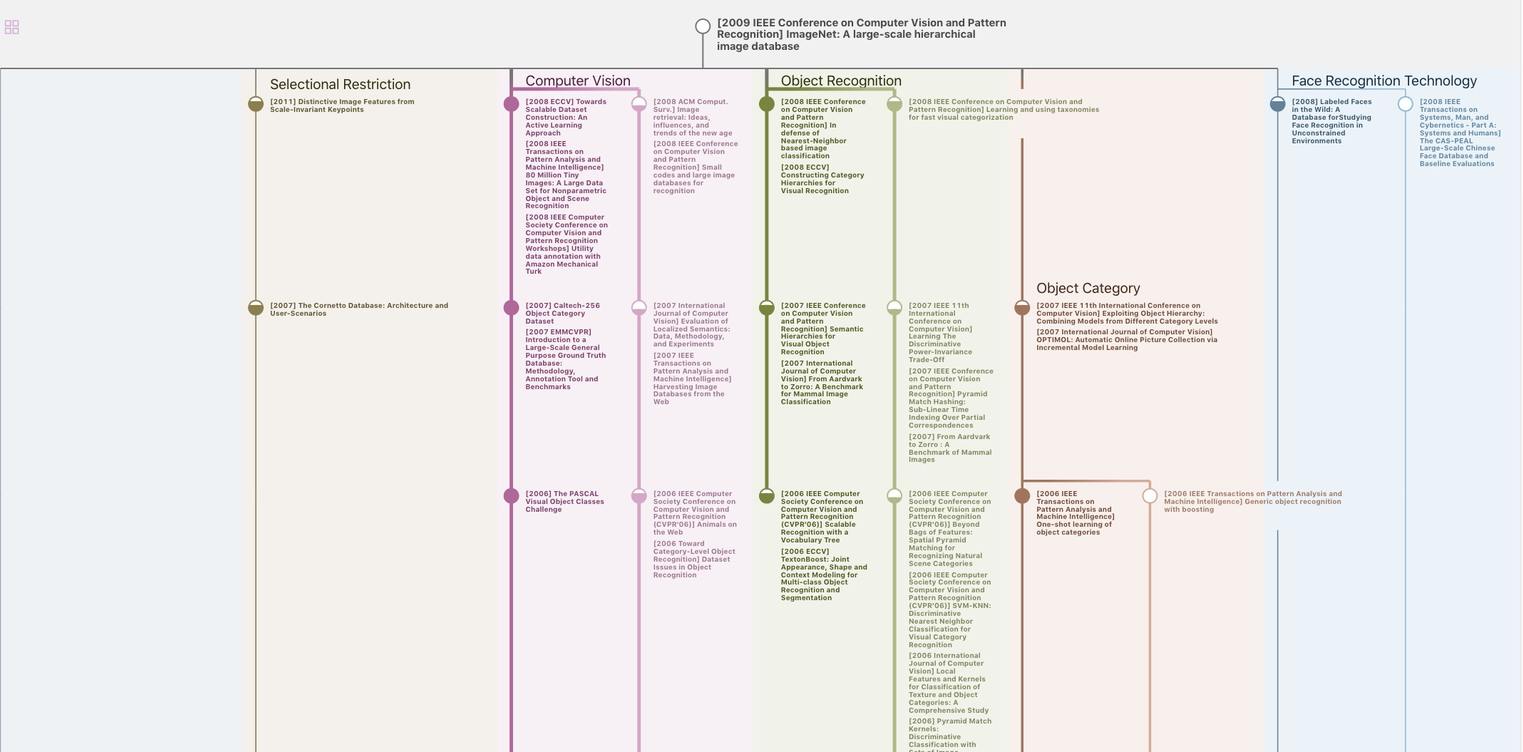
生成溯源树,研究论文发展脉络
Chat Paper
正在生成论文摘要