Fusion and Discrimination: A Multimodal Graph Contrastive Learning Framework for Multimodal Sarcasm Detection
IEEE Transactions on Affective Computing(2024)
摘要
Identifying sarcastic clues from both textual and visual information has become an important research issue, called Multimodal Sarcasm Detection. In this paper, we investigate multimodal sarcasm detection from a novel perspective, where a multimodal graph contrastive learning strategy is proposed to fuse and distinguish the sarcastic clues for textual modality and visual modality. Specifically, we first utilize object detection to derive the crucial visual regions accompanied by their captions of the images, which allows better learning of the key visual regions of visual modality. In addition, to make full use of the semantic information of the visual modality, we employ optical character recognition to extract the textual content in the images. Then, based on image regions, the textual content of visual modality, and the context of the textual modality, we build a multimodal graph for each sample to model the intricate sarcastic relations between modalities. Furthermore, we devise a graph-oriented contrastive learning strategy to leverage the correlations in the same label and differences between different labels, so as to capture better multimodal representations for multimodal sarcasm detection. Extensive experiments show that our method outperforms the previous best baseline models (with a 2.47% improvement in Accuracy, a 1.99% improvement in F-score, and a 2.20% improvement in Macro F-score). The ablation study shows that both multimodal graph structure and graph-oriented contrastive learning are important to our framework. Further, the experiments of using different pre-trained methods show that the proposed multimodal graph contrastive learning framework can directly work with various pre-trained models and achieve outstanding performance in multimodal sarcasm detection.
更多查看译文
关键词
Multimodal sarcasm detection,sarcasm detection,graph model,contrastive learning
AI 理解论文
溯源树
样例
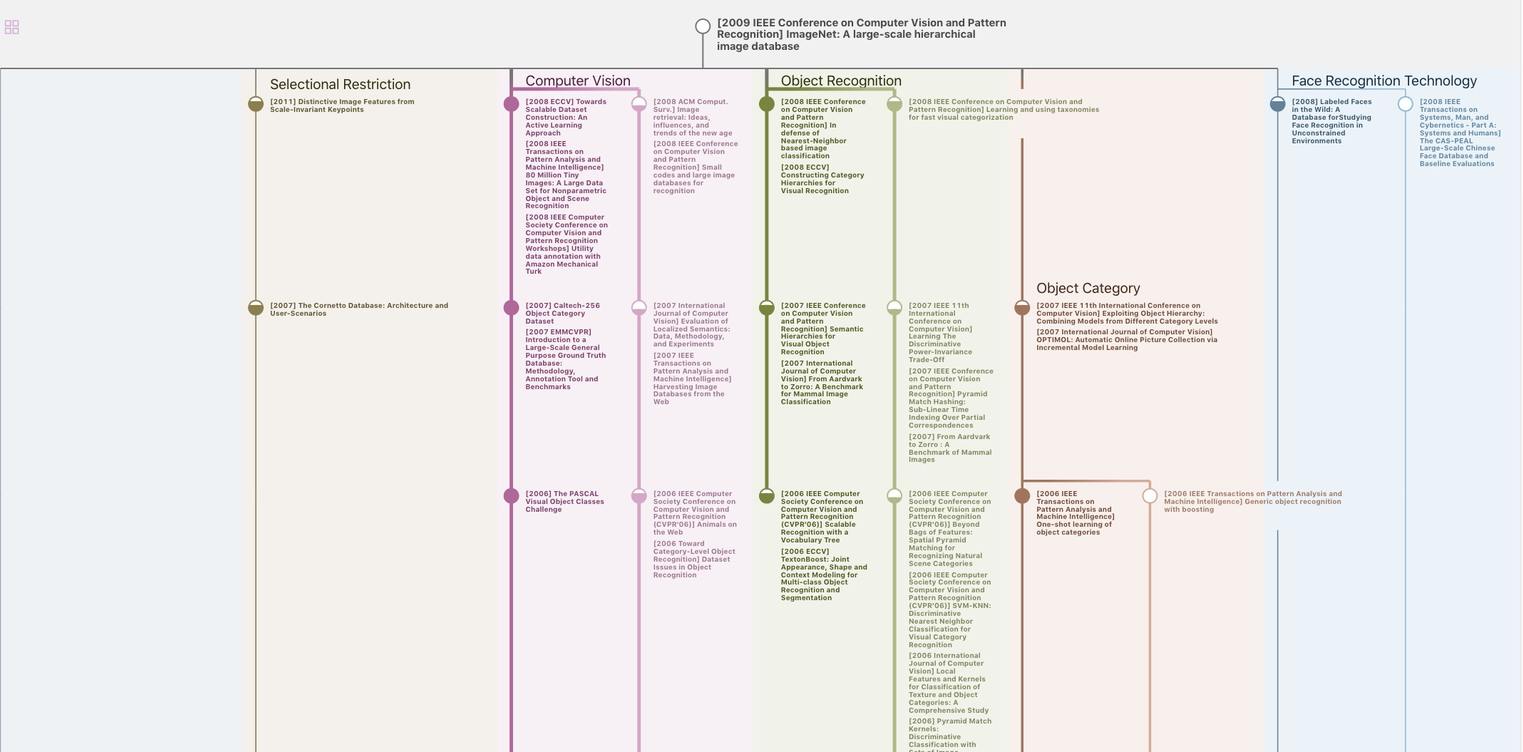
生成溯源树,研究论文发展脉络
Chat Paper
正在生成论文摘要