Exploring the Reliability of IoT Packet Classifiers: An Experimental Study.
GLOBECOM (Workshops)(2023)
摘要
Making robust inferences from loT network traffic is needed for the reliable management of loT devices in health, industrial, or agricultural domains, particularly at scale. Ensemble methods aim to enhance accuracy and consistency by aggregating predictions from multiple classification models. However, the process of combining several models can be difficult due to disagreements in their predictions. This research examines the causes of packet-based classifiers disagreement, specifically when and why packets receive multiple prediction labels. We develop a method that identifies packets that cause disagreement and show how disagreements can provide information on the reliability of the prediction from the classifier. For this study, we adapted three classifiers from previous research on loT traffic inference. The classifiers were applied to public traces of loT packets. Our results indicate that disagreement varies across loT classes and is lower in certain protocols, such as SSDP and NTP at the application layer and LLC at the datalink layer. We also found that when the three classifiers agree on a prediction, there is a 97% chance that it is correct. By removing disagreeing predictions, an ensemble classifier's accuracy can be increased.
更多查看译文
关键词
Machine Learning,Packet Classification,Reli-able Inference,Internet of Things
AI 理解论文
溯源树
样例
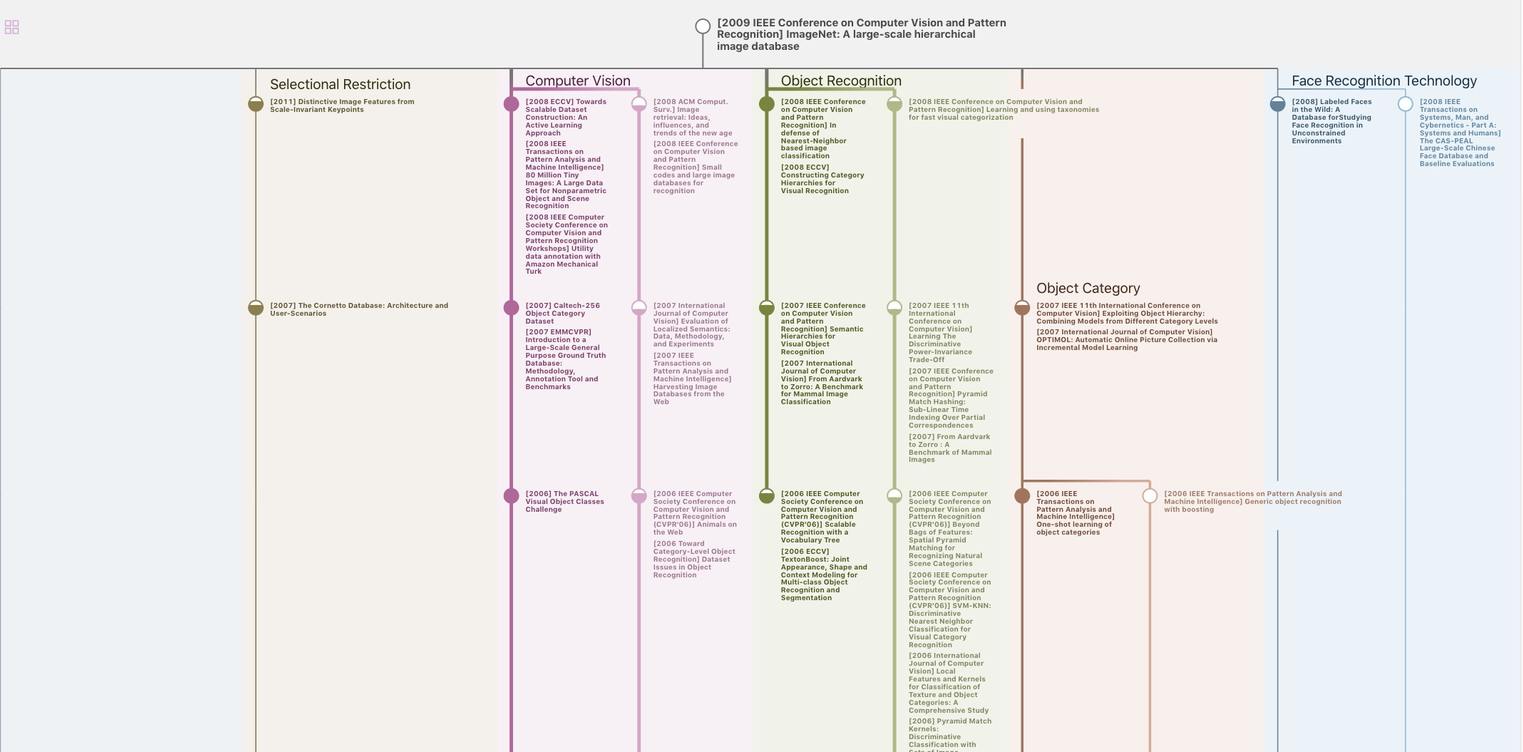
生成溯源树,研究论文发展脉络
Chat Paper
正在生成论文摘要