Self-tuning iterative learning control for an USV: Application to an autonomous berthing operation with an avoidance obstacle mechanism
Ocean Engineering(2024)
摘要
This note focuses on the path planning and path-following control in the task of berthing for the underactuated surface vessel (USV), aiming to realize these functions that the accuracy of path-following can be preset and the smoother path planning based on the task of berthing. The proposed scheme is formulated as two components, i.e., the potential field virtual ship (PFVS) guidance part and the self-tuning iterative learning control (STILC) part. By utilizing one novel repulsive field applied around the obstacle and one corrected potential field, the PFVS guidance law is developed in the scheme to plan the reference path and program the real-time heading angle for the USV. The main superiorities of this design are that the guidance signal buffeting and target unreachable problem have been evaded in obstacle avoidance. In addition, the reference speed has been ingenious combined with the artificial potential field (APF). Furthermore, to improve the suitability of the closed-loop control system with ocean engineering, the iterative learning control (ILC) has been improved by introducing the input event-triggered mechanism. An STILC algorithm is designed for preset accuracy can achieve and reduce computing burden. In this algorithm, the number of iterations would self-turning based on the preset accurate. The computation burden of the proposed controller is reduce with the decreasing number of iterations. Through the Lyapunov analysis, we have exerted sufficient effort to ensure that all signals of the closed-loop control system are semi-globally uniformly ultimately bounded (SGUUB). The simulation experiments have been presented to verify the obstacle avoidance effectiveness and the burden-some superiority of the algorithm.
更多查看译文
关键词
Potential field virtual ship guidance,Path planning,Path-following control,Underactuated surface vessel,Event-triggered mechanism
AI 理解论文
溯源树
样例
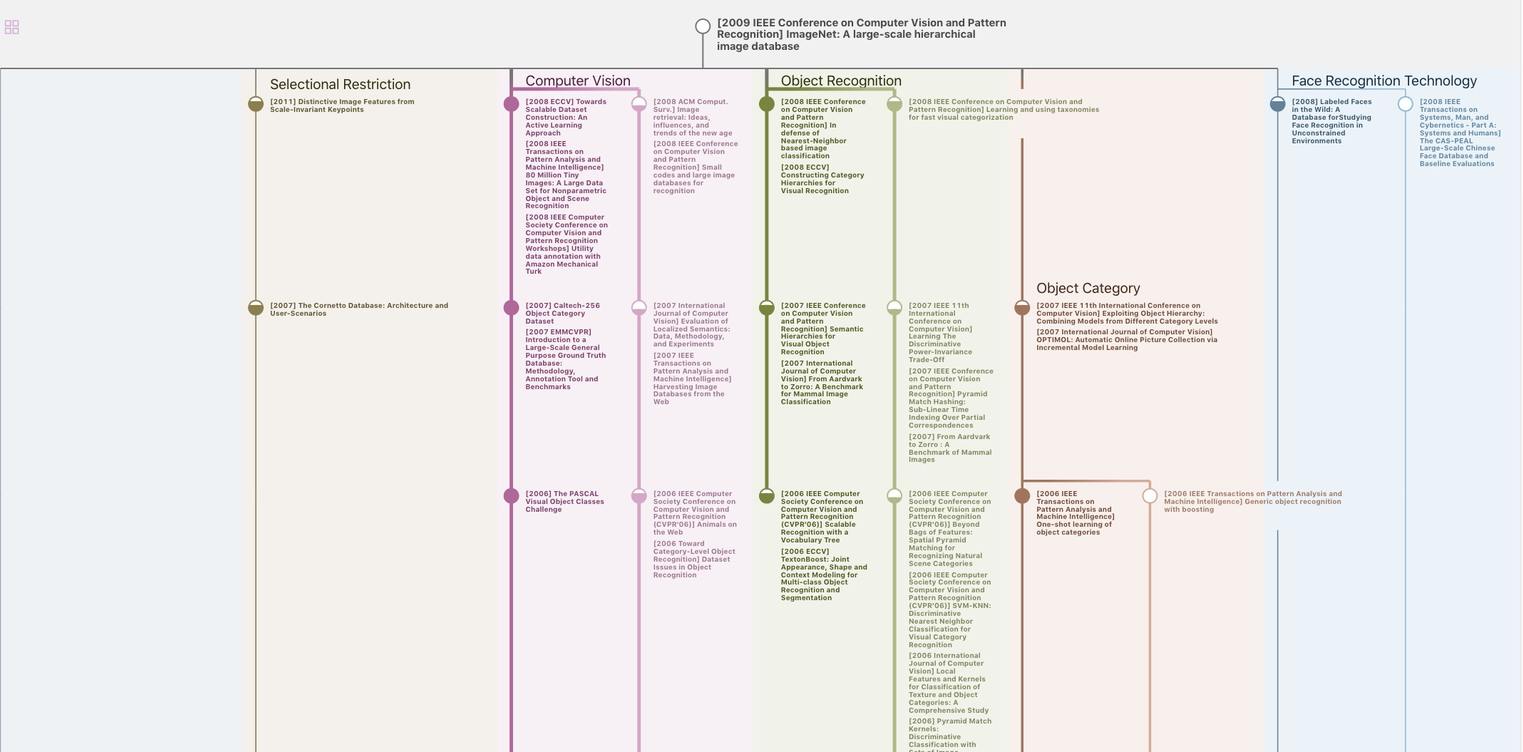
生成溯源树,研究论文发展脉络
Chat Paper
正在生成论文摘要