Radiomics model based on intratumoral and peritumoral features for predicting major pathological response in non-small cell lung cancer receiving neoadjuvant immunochemotherapy
FRONTIERS IN ONCOLOGY(2024)
摘要
Objective: To establish a radiomics model based on intratumoral and peritumoral features extracted from pre-treatment CT to predict the major pathological response (MPR) in patients with non-small cell lung cancer (NSCLC) receiving neoadjuvant immunochemotherapy. Methods: A total of 148 NSCLC patients who underwent neoadjuvant immunochemotherapy from two centers (SRRSH and ZCH) were retrospectively included. The SRRSH dataset (n=105) was used as the training and internal validation cohort. Radiomics features of intratumoral (T) and peritumoral regions (P1 = 0-5mm, P2 = 5-10mm, and P3 = 10-15mm) were extracted from pre-treatment CT. Intra- and inter- class correlation coefficients and least absolute shrinkage and selection operator were used to feature selection. Four single ROI models mentioned above and a combined radiomics (CR: T+P1+P2+P3) model were established by using machine learning algorithms. Clinical factors were selected to construct the combined radiomics-clinical (CRC) model, which was validated in the external center ZCH (n=43). The performance of the models was assessed by DeLong test, calibration curve and decision curve analysis. Results: Histopathological type was the only independent clinical risk factor. The model CR with eight selected radiomics features demonstrated a good predictive performance in the internal validation (AUC=0.810) and significantly improved than the model T (AUC=0.810 vs 0.619, p<0.05). The model CRC yielded the best predictive capability (AUC=0.814) and obtained satisfactory performance in the independent external test set (AUC=0.768, 95% CI: 0.62-0.91). Conclusion: We established a CRC model that incorporates intratumoral and peritumoral features and histopathological type, providing an effective approach for selecting NSCLC patients suitable for neoadjuvant immunochemotherapy.
更多查看译文
关键词
lung neoplasms,machine learning,immunotherapy,neoadjuvant therapy,peritumor
AI 理解论文
溯源树
样例
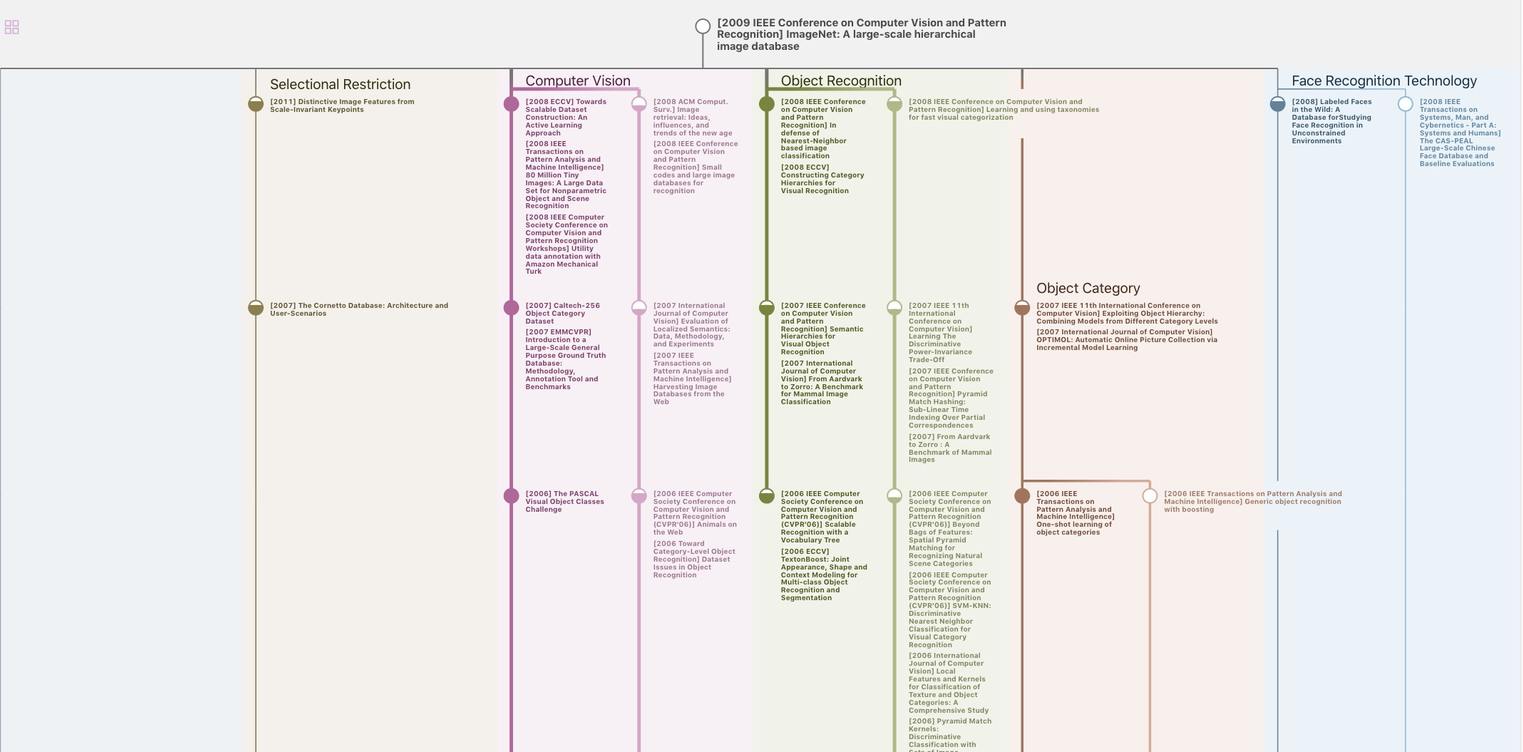
生成溯源树,研究论文发展脉络
Chat Paper
正在生成论文摘要