Wavelet integrated multiscale feature fusion network for imbalanced skin lesion classification
crossref(2024)
摘要
Abstract Accurate medical skin lesion classification is crucial for disease diagnosis and treatment planning. However, current methods are challenged by the presence of scattered feature, imbalanced class distributions and adverse effects of noise. To address these issues, a wavelet integrated multi-scale feature fusion network for imbalanced skin lesion classification is proposed. Firstly, a multi-scale feature fusion network (MSFuse) is designed, which contains parallel local and global feature modules to efficiently capture local spatial context features and global semantic information representation of features at different scales, while implementing adaptive hierarchical feature fusion blocks (HFF) to fuse semantic information between features at different scales in each branch. Subsequently, a Multi-Weighted New Loss (MWNL) function is proposed to overcome the challenge of uneven sample size and classification difficulty while reducing the impact of abnormal samples on training. Finally, the discrete wavelet transform (DWT) is incorporated instead of the average pooling of the fusion branch in MSFuse to remove the high-frequency components of the output features and extract the high-level features from the low-frequency components to suppress the effect of noise on the final classification results and improve the classification accuracy. Ablation experiments and comparison experiments are conducted on the ISIC2018 dataset, and the subjective and objective experimental results demonstrate that the proposed network outperforms the state-of-the-art methods and achieves the superior skin lesion classification results with 84.42%, 70.35%, 74.53%, 74.76% and 74.34% for Acc, MCC, F1, Prec, and Recall, respectively.
更多查看译文
AI 理解论文
溯源树
样例
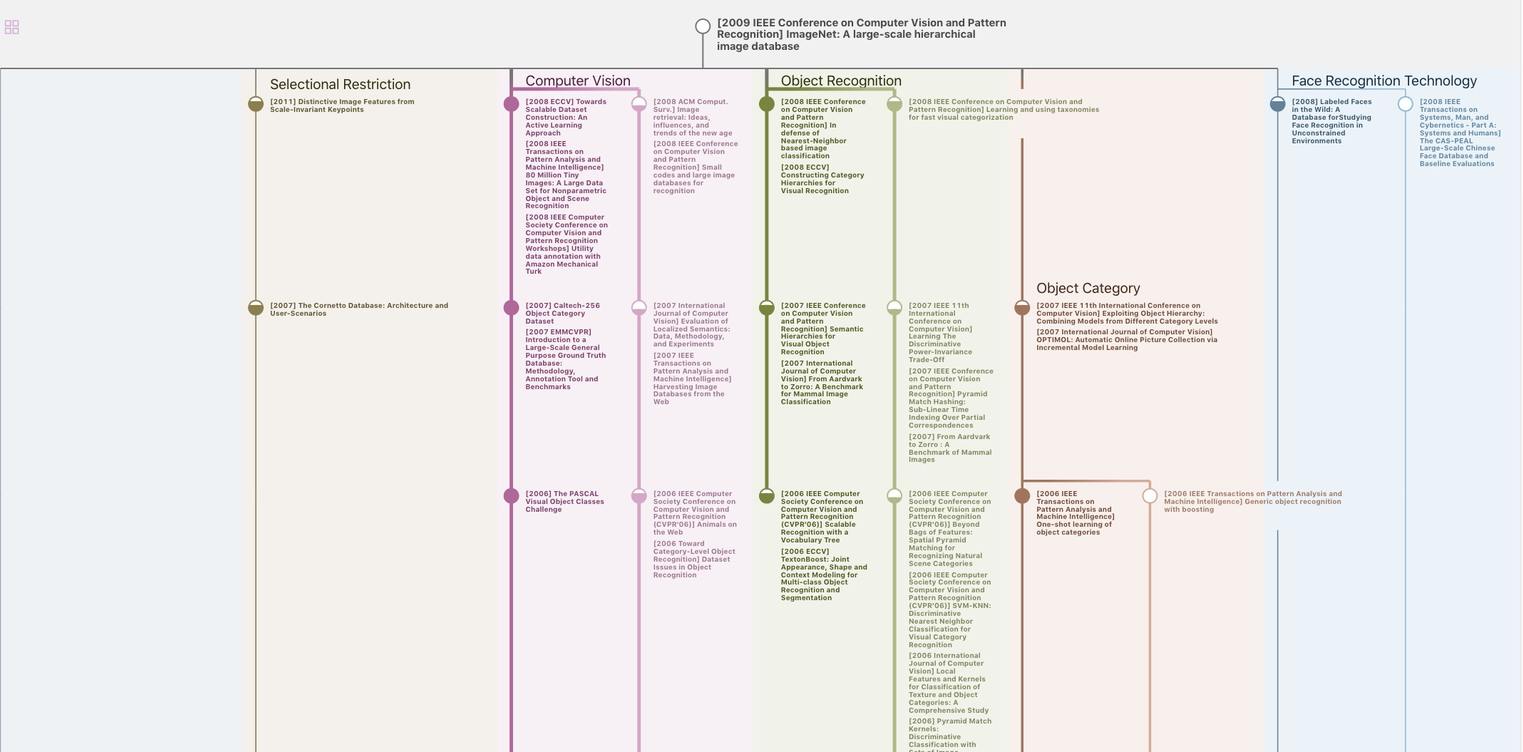
生成溯源树,研究论文发展脉络
Chat Paper
正在生成论文摘要