Momentum-based distributed gradient tracking algorithms for distributed aggregative optimization over unbalanced directed graphs
Automatica(2024)
摘要
This paper studies a distributed aggregative optimization problem over a directed graph with the row-stochastic weighted matrix. Different from the existing work on distributed optimization, the local cost function of each agent depends both on its local decision variable and on the sum of all functions formed by the decision variables of all agents. Inspired by the distributed dynamic average consensus protocol, heavy-ball strategy, and Nesterov gradient descent method, a momentum-based distributed gradient tracking algorithm with a fixed step size is proposed to solve such a problem. Further, it is shown that the proposed algorithm has a linear convergence rate if the global cost function is strongly convex with the Lipschitz-continuous gradient. The upper bounds of the fixed step size and the momentum parameter are restricted by a sufficiently small positive constant, respectively. Finally, a numerical example is provided to verify the effectiveness of the findings.
更多查看译文
关键词
Distributed aggregative optimization,Row-stochastic weighted matrix,Gradient tracking,Acceleration,Linear convergence
AI 理解论文
溯源树
样例
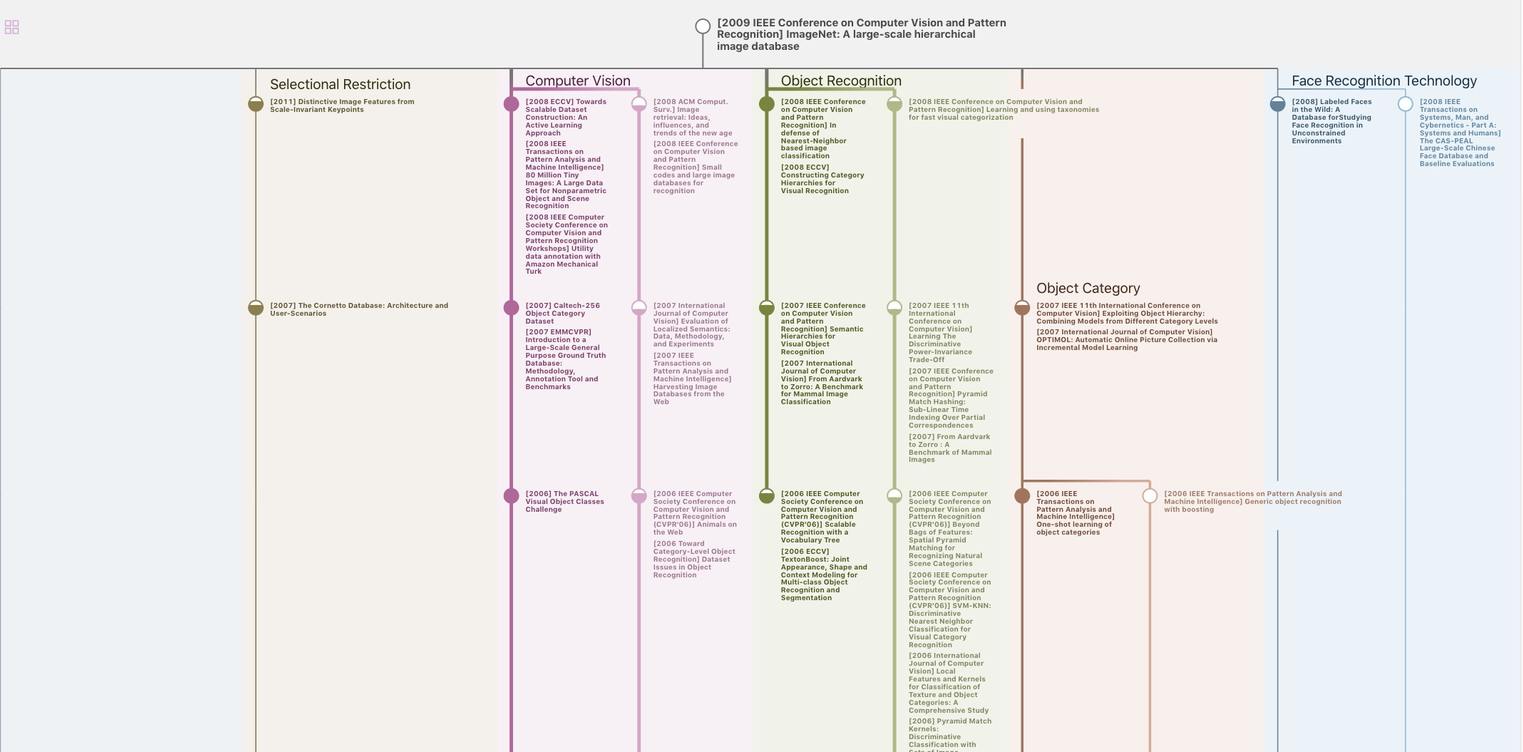
生成溯源树,研究论文发展脉络
Chat Paper
正在生成论文摘要