A classification and quantitative assessment method for internal and external surface defects in pipelines based on ASTC-Net
Advanced Engineering Informatics(2024)
摘要
Classification and size quantification of defects on both the internal and external surfaces of pipelines are critical to pipeline integrity assessment. However, defect classification is challenging because of the similarities of defect signals on the internal and external surfaces. In addition, most existing size quantification methods are not sufficiently accurate. To solve these problems, this paper proposes a classification and quantitative evaluation method based on an asymmetric student–teacher with a classifier network (ASTC-Net). First, a novel approach for expanding defect magnetic flux leakage (MFL) data is validated through experiments and simulations. Second, ASTC-Net is built to address the problem of defect classification and quantification. Finally, the superiority of the method is verified by experiments. The results show that this approach pioneers the accurate classification of defects on both internal and external surfaces by achieving an accuracy of 99.41%. Furthermore, a high-precision quantitative assessment of defect size is realized, with length, width, and depth errors of only 0.35 mm, 0.34 mm, and 0.41% of the wall thickness, respectively. These experimental results clearly demonstrate that this method has exceptionally high accuracy in defect classification and quantification, offering vast prospects for its application in pipeline MFL evaluation.
更多查看译文
关键词
Pipeline leak detection,Classification,Quantification,Magnetic shielding,Magnetic flux leakage,ASTC-Net
AI 理解论文
溯源树
样例
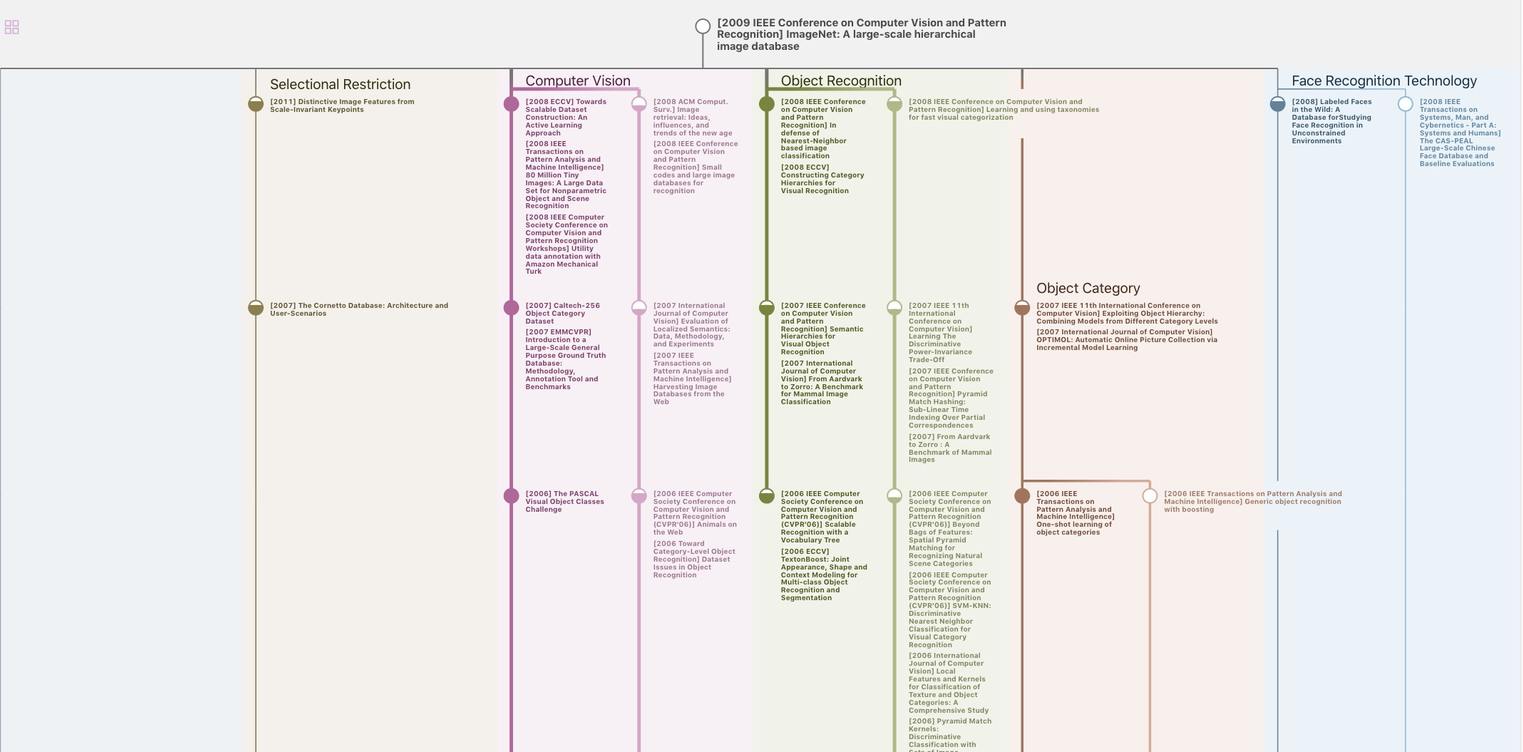
生成溯源树,研究论文发展脉络
Chat Paper
正在生成论文摘要