Multi-Space Alignments Towards Universal LiDAR Segmentation
CVPR 2024(2024)
Abstract
A unified and versatile LiDAR segmentation model with strong robustness and
generalizability is desirable for safe autonomous driving perception. This work
presents M3Net, a one-of-a-kind framework for fulfilling multi-task,
multi-dataset, multi-modality LiDAR segmentation in a universal manner using
just a single set of parameters. To better exploit data volume and diversity,
we first combine large-scale driving datasets acquired by different types of
sensors from diverse scenes and then conduct alignments in three spaces, namely
data, feature, and label spaces, during the training. As a result, M3Net is
capable of taming heterogeneous data for training state-of-the-art LiDAR
segmentation models. Extensive experiments on twelve LiDAR segmentation
datasets verify our effectiveness. Notably, using a shared set of parameters,
M3Net achieves 75.1
official benchmarks of SemanticKITTI, nuScenes, and Waymo Open.
MoreTranslated text
AI Read Science
Must-Reading Tree
Example
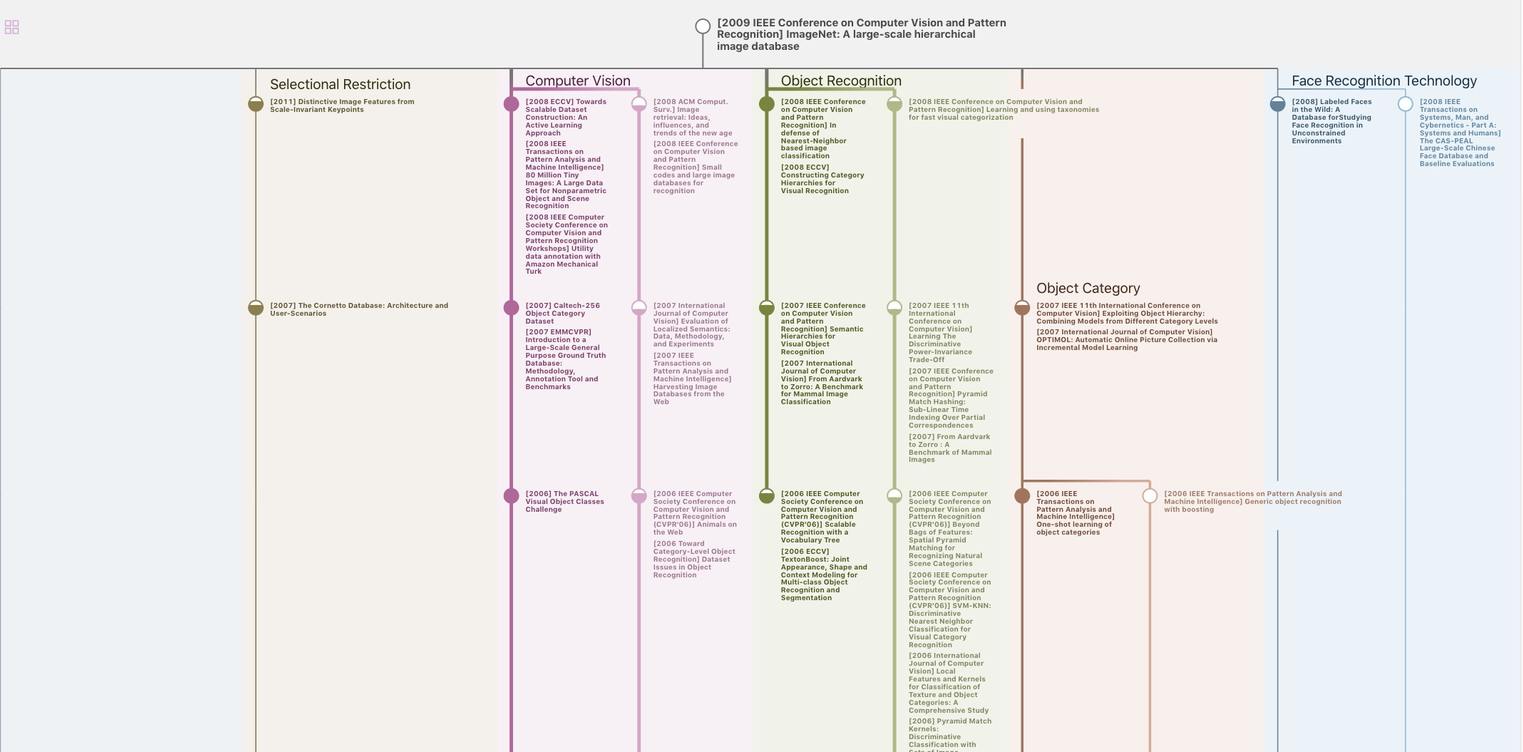
Generate MRT to find the research sequence of this paper
Chat Paper
Summary is being generated by the instructions you defined