De-confounded Data-free Knowledge Distillation for Handling Distribution Shifts
CVPR 2024(2024)
Abstract
Data-Free Knowledge Distillation (DFKD) is a promising task to train
high-performance small models to enhance actual deployment without relying on
the original training data. Existing methods commonly avoid relying on private
data by utilizing synthetic or sampled data. However, a long-overlooked issue
is that the severe distribution shifts between their substitution and original
data, which manifests as huge differences in the quality of images and class
proportions. The harmful shifts are essentially the confounder that
significantly causes performance bottlenecks. To tackle the issue, this paper
proposes a novel perspective with causal inference to disentangle the student
models from the impact of such shifts. By designing a customized causal graph,
we first reveal the causalities among the variables in the DFKD task.
Subsequently, we propose a Knowledge Distillation Causal Intervention (KDCI)
framework based on the backdoor adjustment to de-confound the confounder. KDCI
can be flexibly combined with most existing state-of-the-art baselines.
Experiments in combination with six representative DFKD methods demonstrate the
effectiveness of our KDCI, which can obviously help existing methods under
almost all settings, e.g., improving the baseline by up to 15.54%
accuracy on the CIFAR-100 dataset.
MoreTranslated text
AI Read Science
Must-Reading Tree
Example
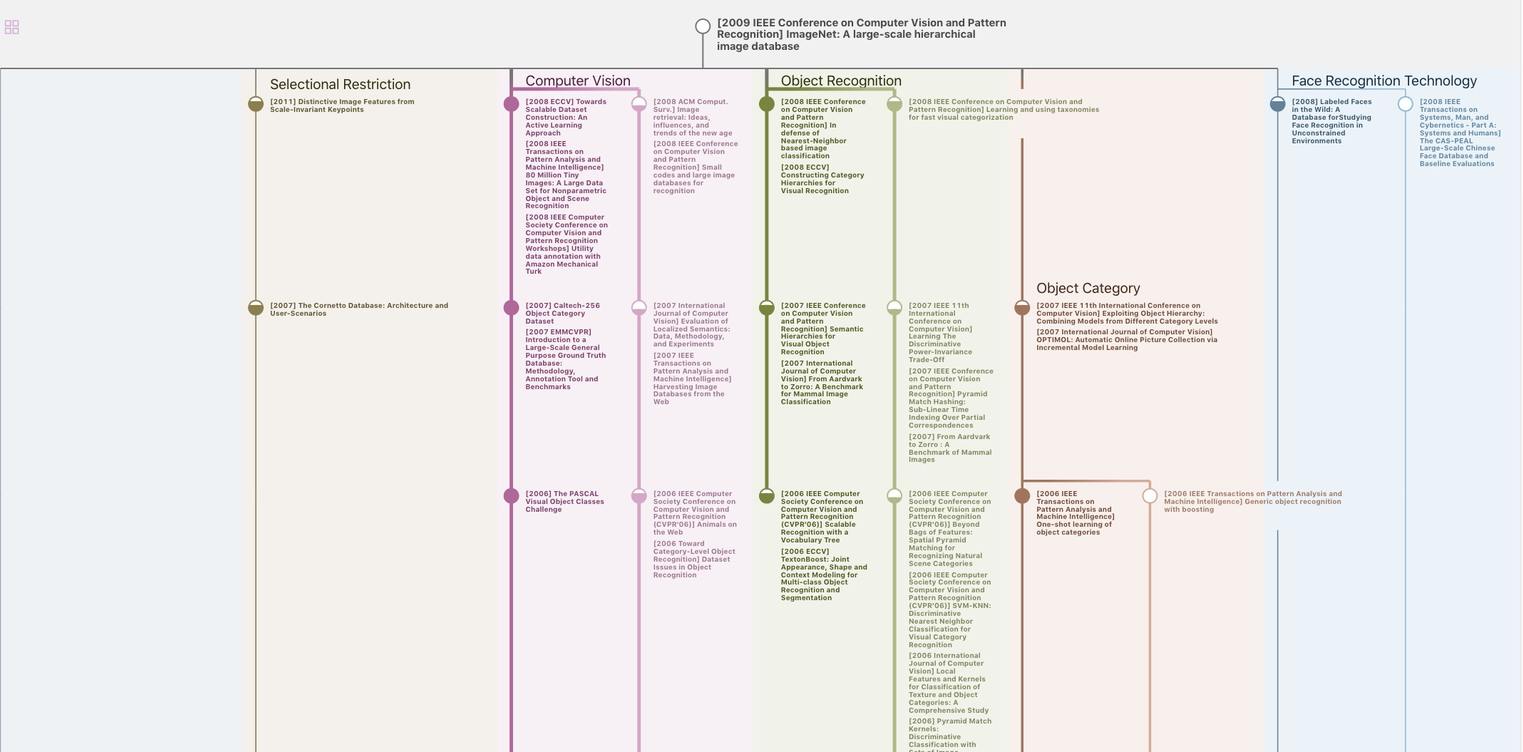
Generate MRT to find the research sequence of this paper
Chat Paper
Summary is being generated by the instructions you defined