Continuous Learning for Anomaly Detection: A Case Study in Volcanic Unrest Monitoring
2024 International Conference on Artificial Intelligence, Computer, Data Sciences and Applications (ACDSA)(2024)
摘要
In the field of volcanology, timely detection of anomalies is essential for disaster mitigation. Traditional methods often fall short in adapting to evolving volcanic behavior. We propose a model that combines continual learning and autoencoders to adaptively detect anomalies. The autoencoder extracts relevant features from sensor data, while continual learning enables the model to adapt to changing volcanic patterns. A case study demonstrates its effectiveness in real-time monitoring, offering a data-driven and efficient solution for volcanic anomaly detection.
更多查看译文
关键词
Anomaly Detection,Time series Analysis,Continual Learning,Volcanic Unrest
AI 理解论文
溯源树
样例
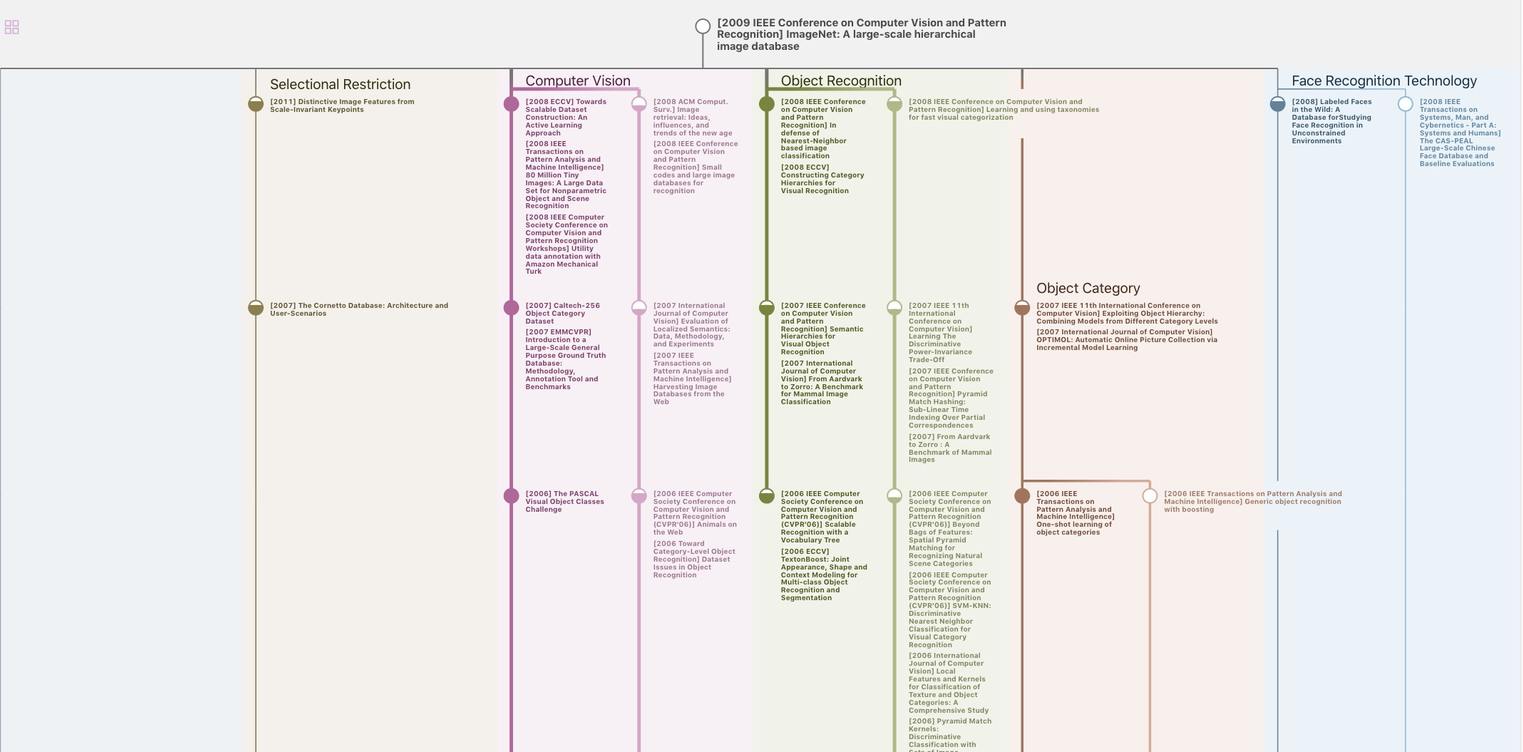
生成溯源树,研究论文发展脉络
Chat Paper
正在生成论文摘要