DC-MPAM: A Novel Customized Driving Cycle Construction Method for Predicting Cruising Distance and Vehicle Speed
IEEE Transactions on Transportation Electrification(2024)
Abstract
Standard driving cycles fail to represent specific road conditions accurately, leading to significant discrepancies when calculating cruising distances in comparison to actual driving miles. To address this, this paper proposes a novel multi-parameter and mutation driving cycle generation method (DC-MPAM). This method rapidly and accurately generates customized driving cycles by extracting six velocity feature parameters, adaptive classification parameters, and mutation parameters from historical vehicle speed data. A hierarchical verification approach is employed to ensure the accuracy of the results. Comparative analysis with existing methods has shown that the DC-MPAM method can significantly improve the precision of generated driving cycles by more than 5.3% across different datasets. This study predicts the range based on actual State of Charge (SOC) consumption, the State of Health (SOH) of the battery pack, and the impact of temperature changes. Compared to standard driving cycles, the predictive accuracy has increased by 2.24% to 8.93%. Additionally, the research incorporates the concept of transfer learning, utilizing the Transfer-ARBF vehicle speed prediction model to validate the significance of using custom driving cycles for pre-training of vehicle speed prediction models.
MoreTranslated text
Key words
electric bus,driving cycle,multi-parameters,mutation speed,predicted cruising distance,predicted speed
AI Read Science
Must-Reading Tree
Example
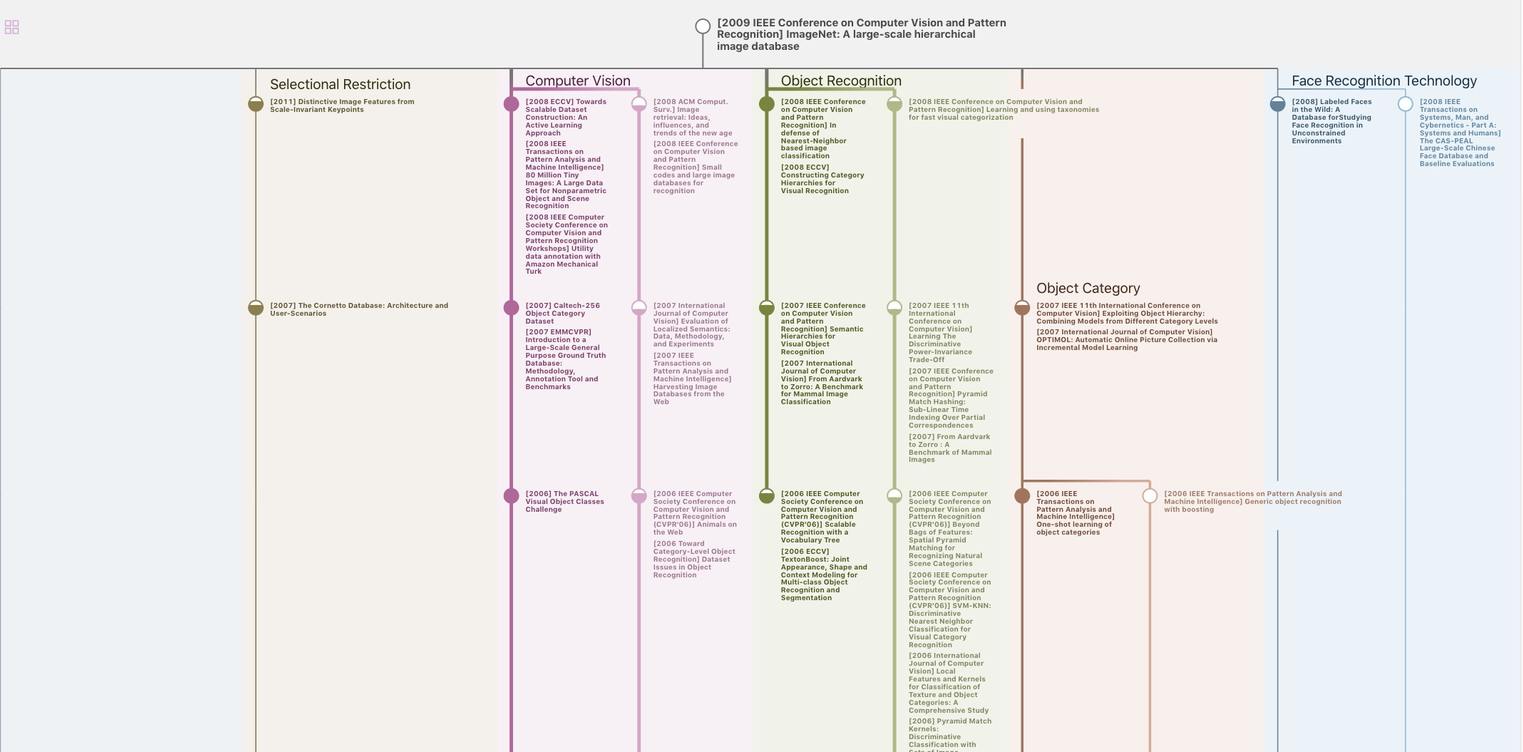
Generate MRT to find the research sequence of this paper
Chat Paper
Summary is being generated by the instructions you defined