PNAS-MOT: Multi-Modal Object Tracking With Pareto Neural Architecture Search
IEEE ROBOTICS AND AUTOMATION LETTERS(2024)
摘要
Multiple object tracking is a critical task in autonomous driving. Existing works primarily focus on the heuristic design of neural networks to obtain high accuracy. As tracking accuracy improves, however, neural networks become increasingly complex, posing challenges for their practical application in real driving scenarios due to the high level of latency. In this letter, we explore the use of the neural architecture search (NAS) methods to search for efficient architectures for tracking, aiming for low real-time latency while maintaining relatively high accuracy. Another challenge for object tracking is the unreliability of a single sensor, therefore, we propose a multi-modal framework to improve the robustness. Experiments demonstrate that our algorithm can run on edge devices within lower latency constraints, thus greatly reducing the computational requirements for multi-modal object tracking while keeping lower latency.
更多查看译文
关键词
Visual tracking,deep learning methods
AI 理解论文
溯源树
样例
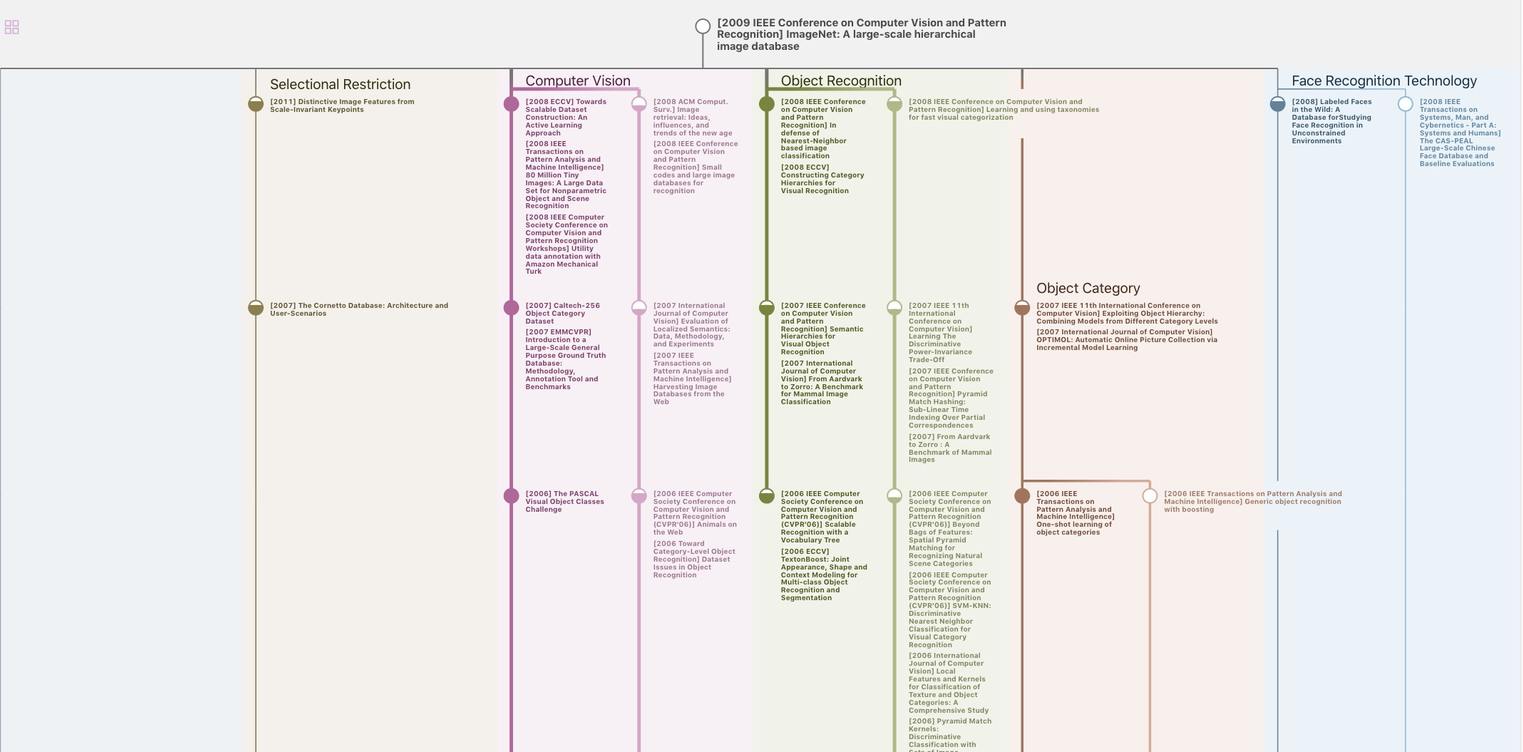
生成溯源树,研究论文发展脉络
Chat Paper
正在生成论文摘要