CGGLNet: Semantic Segmentation Network for Remote Sensing Images Based on Category-Guided Global-Local Feature Interaction
IEEE TRANSACTIONS ON GEOSCIENCE AND REMOTE SENSING(2024)
摘要
As spatial resolution increases, the information conveyed by remote sensing images becomes more and more complex. Large-scale variation and highly discrete distribution of objects greatly increase the challenge of the semantic segmentation task for remote sensing images. Mainstream approaches usually use implicit attention mechanisms or transformer modules to achieve global context for good results. However, these approaches fail to explicitly extract intraobject consistency and interobject saliency features leading to unclear boundaries and incomplete structures. In this article, we propose a category-guided global-local feature interaction network (CGGLNet), which utilizes category information to guide the modeling of global contextual information. To better acquire global information, we proposed a category-guided supervised transformer module (CGSTM). This module guides the modeling of global contextual information by estimating the potential class information of pixels so that features of the same class are more aggregated and those of different classes are more easily distinguished. To enhance the representation of local detailed features of multiscale objects, we designed the adaptive local feature extraction module (ALFEM). By parallel connection of the CGSTM and the ALFEM, our network can extract rich global and local context information contained in the image. Meanwhile, the designed feature refinement segmentation head (FRSH) helps to reduce the semantic difference between deep and shallow features and realizes the full integration of different levels of information. Extensive ablation and comparison experiments on two public remote sensing datasets (ISPRS Vaihingen dataset and ISPRS Potsdam dataset) indicate that our proposed CGGLNet achieves superior performance compared to the state-of-the-art methods.
更多查看译文
关键词
Category-guided,global contextual information,remote sensing,semantic segmentation,transformer
AI 理解论文
溯源树
样例
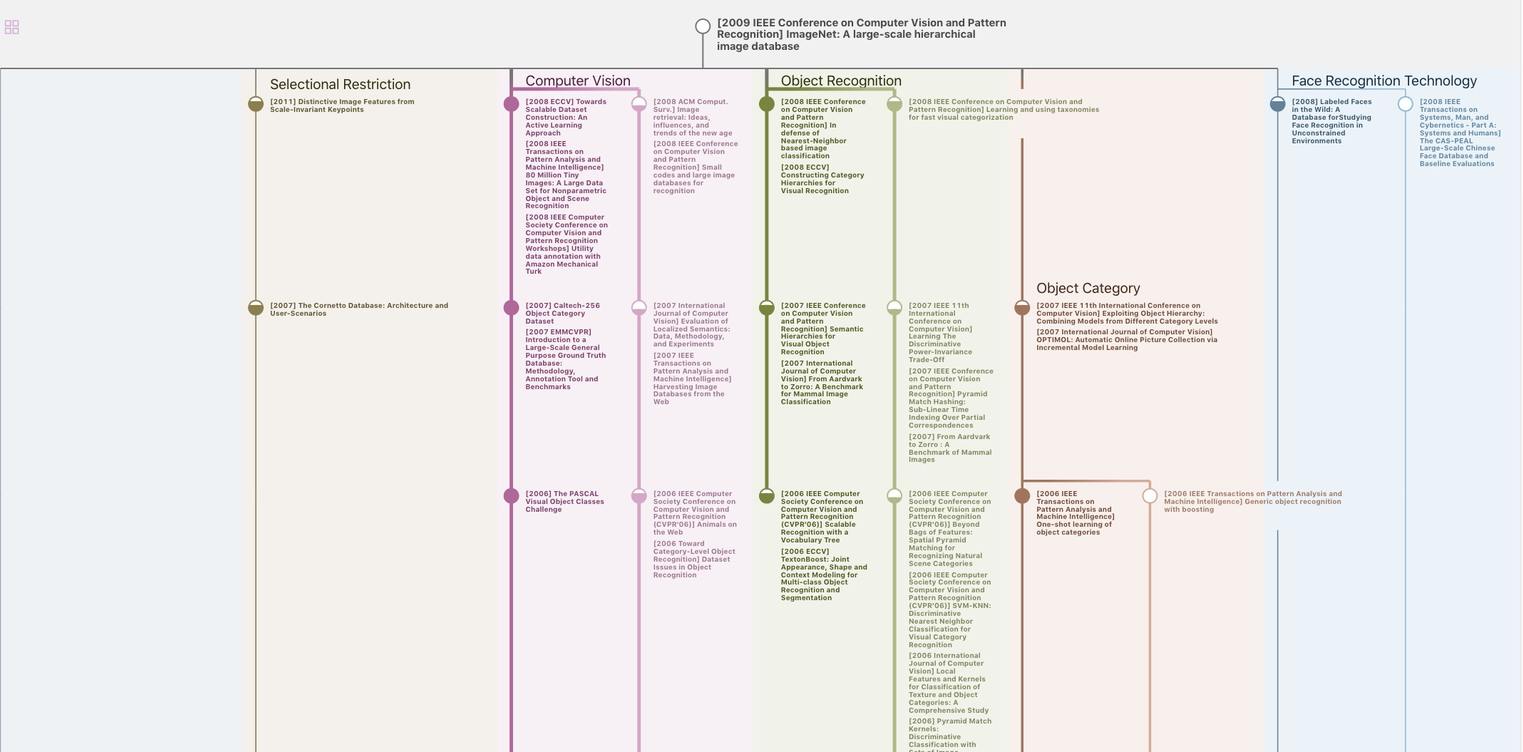
生成溯源树,研究论文发展脉络
Chat Paper
正在生成论文摘要