R2-GVIO: A Robust, Real-Time GNSS-Visual-Inertial State Estimator in Urban Challenging Environments
IEEE Internet of Things Journal(2024)
摘要
Visual-Inertial Odometry (VIO) often suffers from drifting, particularly in large-scale environments. Concurrently, the Global Navigation Satellite System (GNSS) signals will be intermittent or even inaccessible in obstructed environments. In this work, we present a robust, real-time GNSS-visual-inertial state estimator, abbreviated as R-GVIO, that achieves drift-free six-degree-of-freedom accurate global positioning in urban challenging environments. Specifically, we integrate GNSS into the optimization-based monocular and stereo VIO, enabling the fusion of GNSS Real-time Kinematic (RTK), reprojection error, and IMU pre-integration within a factor graph framework. In addition, to enhance the R-GVIO’s robustness in GNSS-unfriendly environments, we propose an online GNSS measurement outlier detection and culling algorithm based on velocity constraints and a time synchronization mechanism. Furthermore, we propose a GNSS-aided initialization method that provides accurate gravity direction, IMU zero bias, and local-global external parameters. Moreover, we adopt the marginalization strategy of covisibility graph keyframes and add the GNSS factor constraint on the covisibility keyframe pose, ensuring the real-time performance of the system. Experiments on public datasets and real-world experiments verify that in challenging environments, including urban canyons, complex indoor-outdoor, and large-scale environments, the positioning accuracy and robustness of the proposed R-GVIO algorithm are superior to the state-of-the-art algorithms VINS-Fusion, ORB-SLAM3, IC-GVINS, and GICI-LIB. To make contribute to the community, we open sourced the complex environment datasets “SYSU-Campus-GVI” on GitHub.
更多查看译文
关键词
Visual-Inertial Odometry,GNSS,Sensor Fusion,Localization
AI 理解论文
溯源树
样例
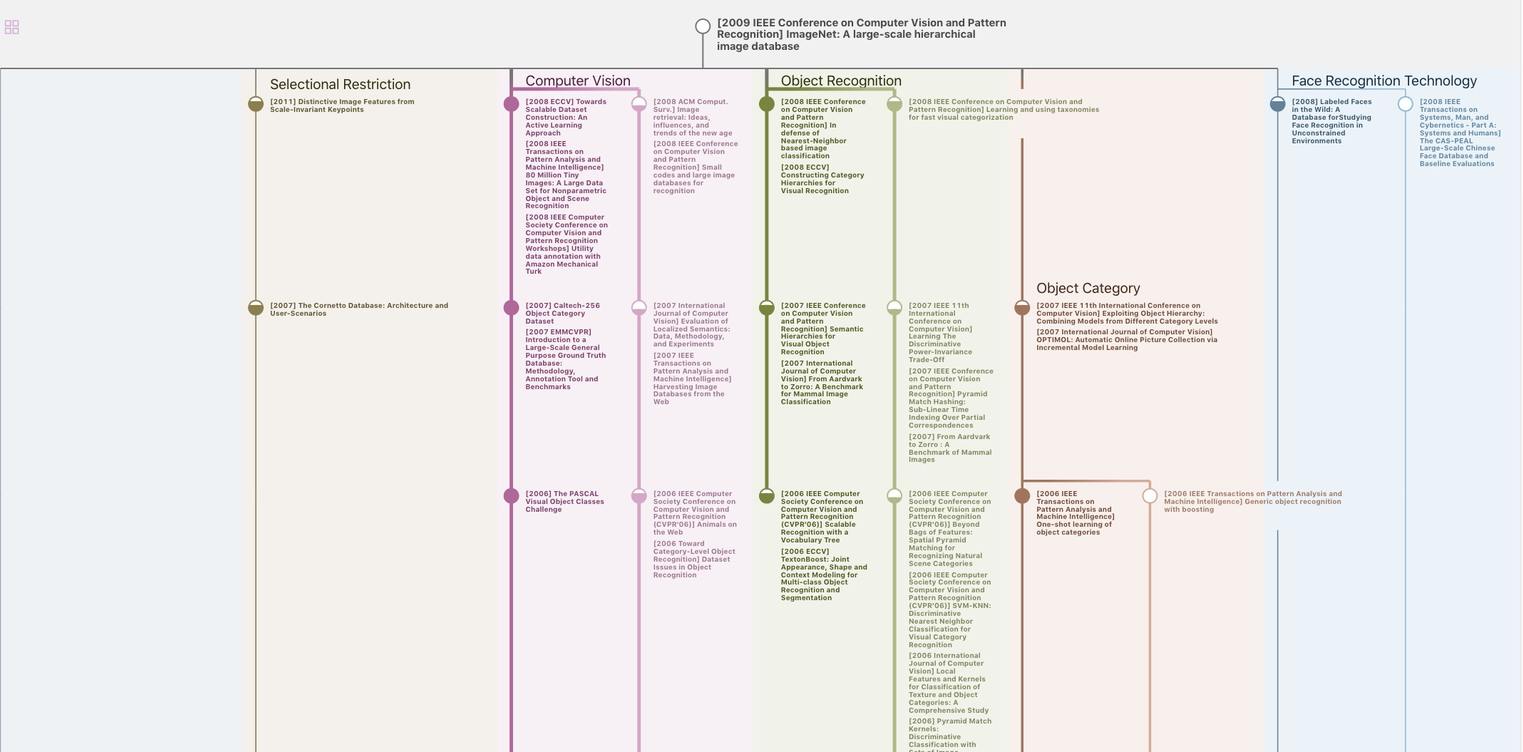
生成溯源树,研究论文发展脉络
Chat Paper
正在生成论文摘要