TT-GCN: Temporal-Tightly Graph Convolutional Network for Emotion Recognition From Gaits
IEEE TRANSACTIONS ON COMPUTATIONAL SOCIAL SYSTEMS(2024)
摘要
The human gait reflects substantial information about individual emotions. Current gait emotion recognition methods focus on capturing gait topology information and ignore the importance of fine-grained temporal features. This article proposes the temporal-tightly graph convolutional network (TT-GCN) to extract temporal features. TT-GCN comprises three significant mechanisms: the causal temporal convolution network (casual-TCN), the walking direction recognition auxiliary task, and the feature mapping layer. To obtain tight temporal dependencies and enhance the relevance among gait periods, the causal-TCN is introduced. Based on the assumption of emotional consistency in the walking directions, the auxiliary task is proposed to enhance the ability of fine-grained feature extraction. Through the feature mapping layer, affective features can be mapped into the appropriate representation and fused with deep learning features. TT-GCN shows the best performance across five comprehensive metrics. All experimental results verify the necessity and feasibility of exploring fine-grained temporal feature extraction.
更多查看译文
关键词
Feature extraction,Legged locomotion,Emotion recognition,Task analysis,Skeleton,Frequency modulation,Arms,gait,graph convolutional network (GCN)
AI 理解论文
溯源树
样例
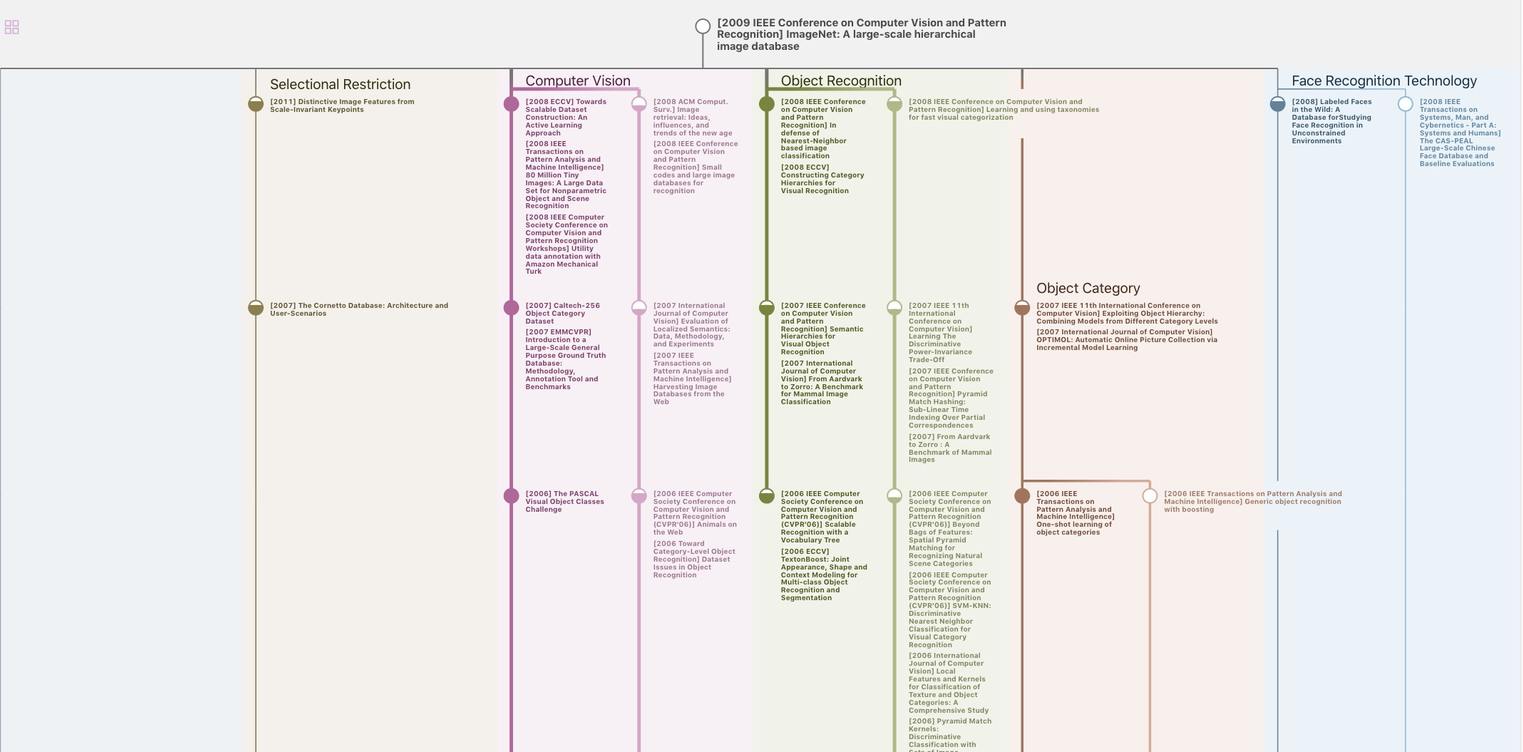
生成溯源树,研究论文发展脉络
Chat Paper
正在生成论文摘要