TLS-MWP: A Tensor-based Long- and Short-range Convolution for Multiple Weather Prediction
IEEE Transactions on Circuits and Systems for Video Technology(2024)
摘要
Weather prediction plays a crucial role in human development. Recently, deep learning has demonstrated promising prospects in weather forecasting by integrating convolutional neural networks (CNNs) and recurrent neural networks (RNNs). However, two main challenges still exist in multiple weather condition prediction. The first challenge considers multiple weather condition correlations in predictions. The second challenge is how to model long- and short-range spatial dependencies under multiple weather conditions. A novel operator named as tensor-based long- and short-range convolution (TLS-Conv) is proposed to address these challenges. Within this operator, the node & relation attention is utilized to identify the contributions of spatial grid points and weather conditions for prediction. Additionally, the adaptive tensor graph convolution (ATGCN) is tailored to dynamically capture long-range spatial dependencies within multiple weather conditions. Finally, the traditional convolution is integrated with the ATGCN to model both long- and short-range spatial dependencies and weather condition correlations. Building upon the TLS-Conv, the tensor-based long- and short-range convolution for multiple weather prediction (TLS-MWP) model is proposed to predict multiple weather conditions. Extensive experiments are conducted under real-world weather conditions to evaluate its performance. These results unequivocally demonstrate that TLS-MWP surpasses previous methods. The code is available on GitHub at: https://github.com/xuguangning1218/TLS_MWP.
更多查看译文
关键词
weather prediction,long-range spatial,spatial temporal,tensor graph
AI 理解论文
溯源树
样例
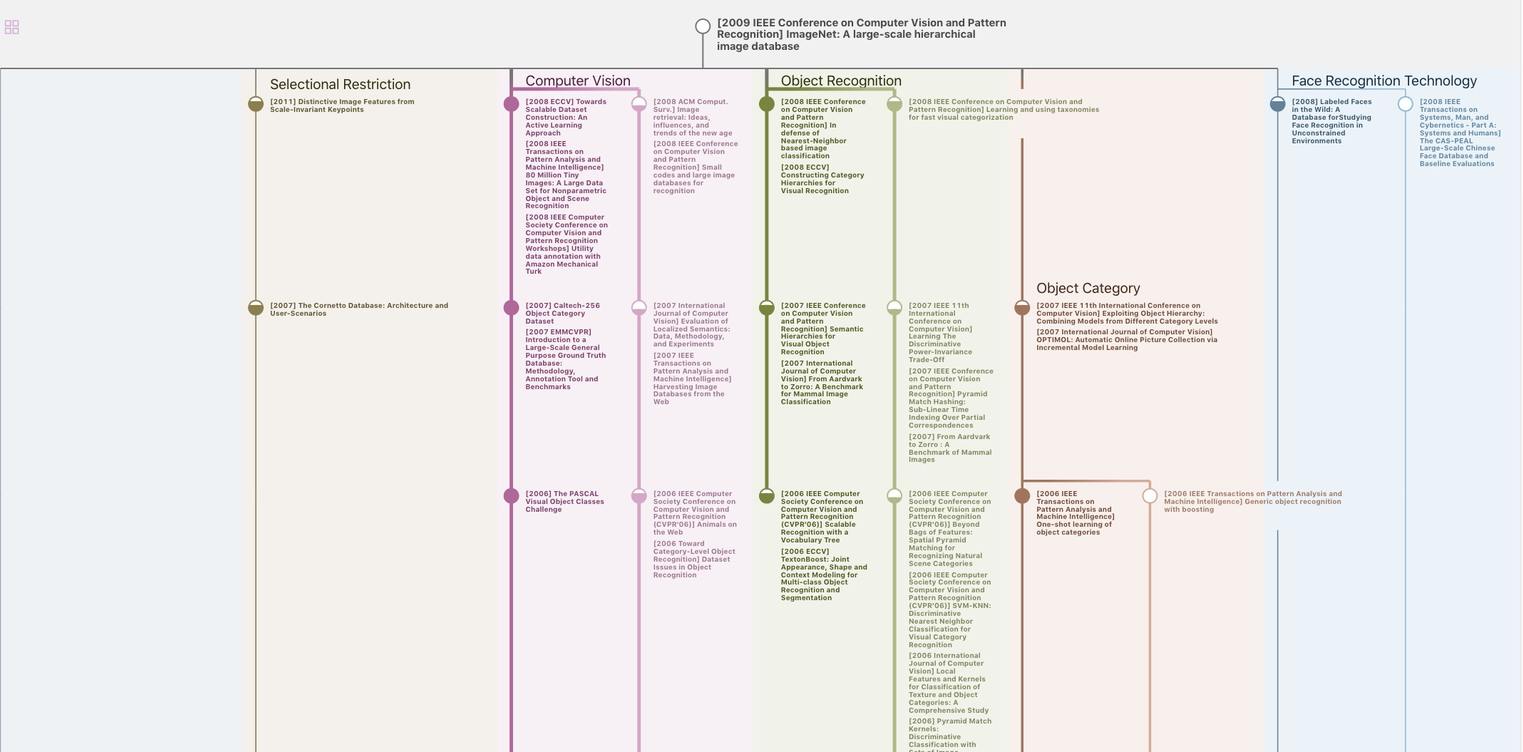
生成溯源树,研究论文发展脉络
Chat Paper
正在生成论文摘要