Uncertainty Quantification and Interpretability for Clinical Trial Approval Prediction.
Health data science(2024)
摘要
Background: Clinical trial is a crucial step in the development of a new therapy (e.g., medication) and is remarkably expensive and time-consuming. Forecasting the approval of clinical trials accurately would enable us to circumvent trials destined to fail, thereby allowing us to allocate more resources to therapies with better chances. However, existing approval prediction algorithms did not quantify the uncertainty and provide interpretability, limiting their usage in real-world clinical trial management. Methods: This paper quantifies uncertainty and improves interpretability in clinical trial approval predictions. We devised a selective classification approach and integrated it with the Hierarchical Interaction Network, the state-of-the-art clinical trial prediction model. Selective classification, encompassing a spectrum of methods for uncertainty quantification, empowers the model to withhold decision-making in the face of samples marked by ambiguity or low confidence. This approach not only amplifies the accuracy of predictions for the instances it chooses to classify but also notably enhances the model's interpretability. Results: Comprehensive experiments demonstrate that incorporating uncertainty markedly enhances the model's performance. Specifically, the proposed method achieved 32.37%, 21.43%, and 13.27% relative improvement on area under the precision-recall curve over the base model (Hierarchical Interaction Network) in phase I, II, and III trial approval predictions, respectively. For phase III trials, our method reaches 0.9022 area under the precision-recall curve scores. In addition, we show a case study of interpretability that helps domain experts to understand model's outcome. The code is publicly available at https://github.com/Vincent-1125/Uncertainty-Quantification-on-Clinical-Trial-Outcome-Prediction. Conclusion: Our approach not only measures model uncertainty but also greatly improves interpretability and performance for clinical trial approval prediction.
更多查看译文
AI 理解论文
溯源树
样例
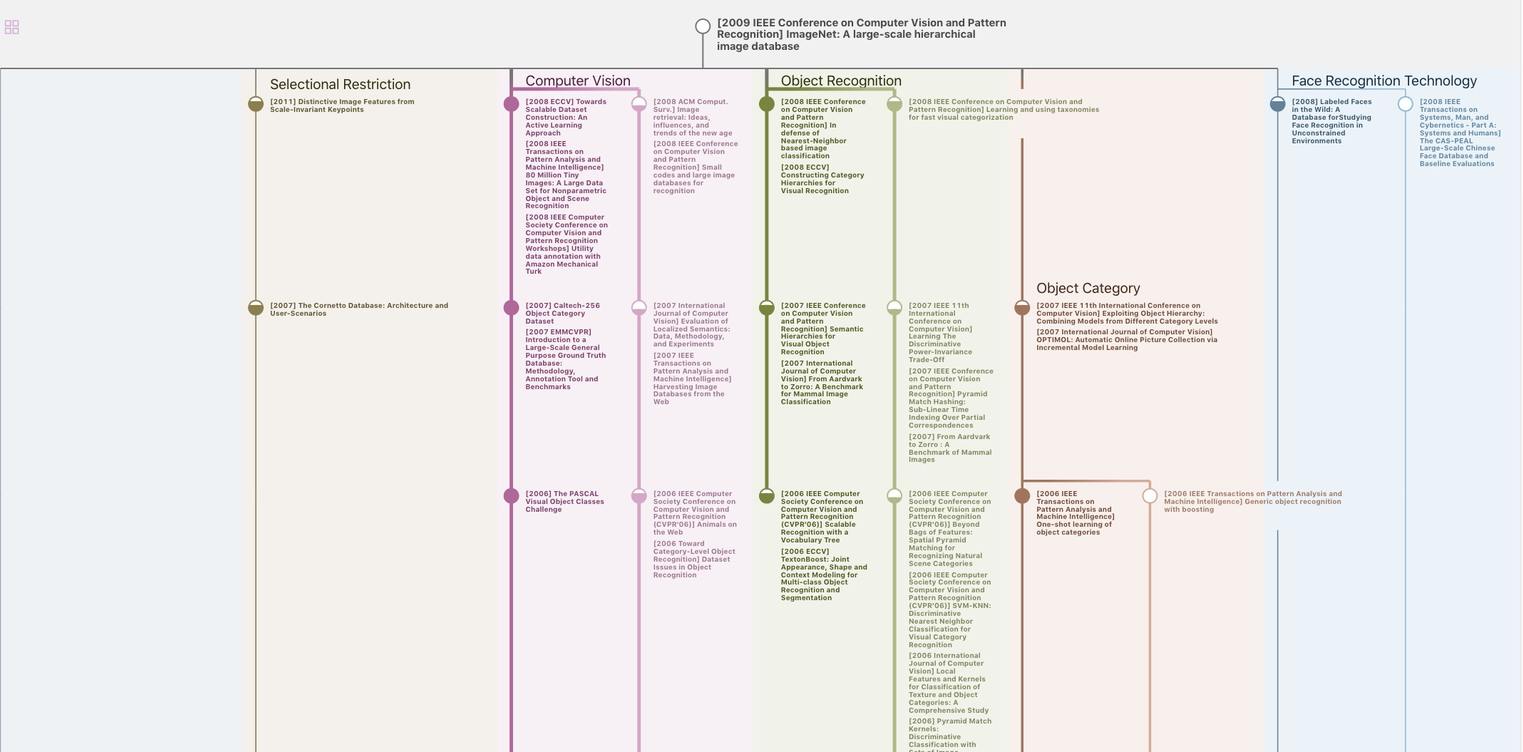
生成溯源树,研究论文发展脉络
Chat Paper
正在生成论文摘要