Towards Efficient Multi-view Representation Learning.
ISM(2023)
Abstract
The proliferation of deep neural networks (DNNs) has drawn unprecedented interest in the study of various contents such as image, audio, video, to name a few. However, due to the data-driven nature, the high computational requirement and slow running time are considered as Achilles’ heels of DNN-based algorithms, limiting the progress of DNNs in time-sensitive applications. Recently, distinct discriminant canonical correlation analysis network (DDCCANet), a multi-view neural network, has shown great generalizability across multiple application domains, both analytically and experimentally. However, Although the computational requirement and running time by DDCCANet are more manageable than DNN-based algorithms, they can be substantially further improved. This paper proposes two new algorithms for multi-view feature representation learning, namely incremental DDCCANet (IDDCCANet) with substantial save in computational memory and GPU-accelerated DDCCANet (GADDCCANet) with drastically accelerated running time, forming a practically significant platform for multi-view feature representation learning. To validate the power of the proposed algorithms, experiments are conducted on several data sets with different types of inputs (e.g., raw image pixels, classical and DNN-based features). Experimental results clearly show that the proposed algorithms provide promising solutions to address the two longstanding challenges.
MoreTranslated text
Key words
Efficient,Multi-view,DDCCA,Fusion,CNN
AI Read Science
Must-Reading Tree
Example
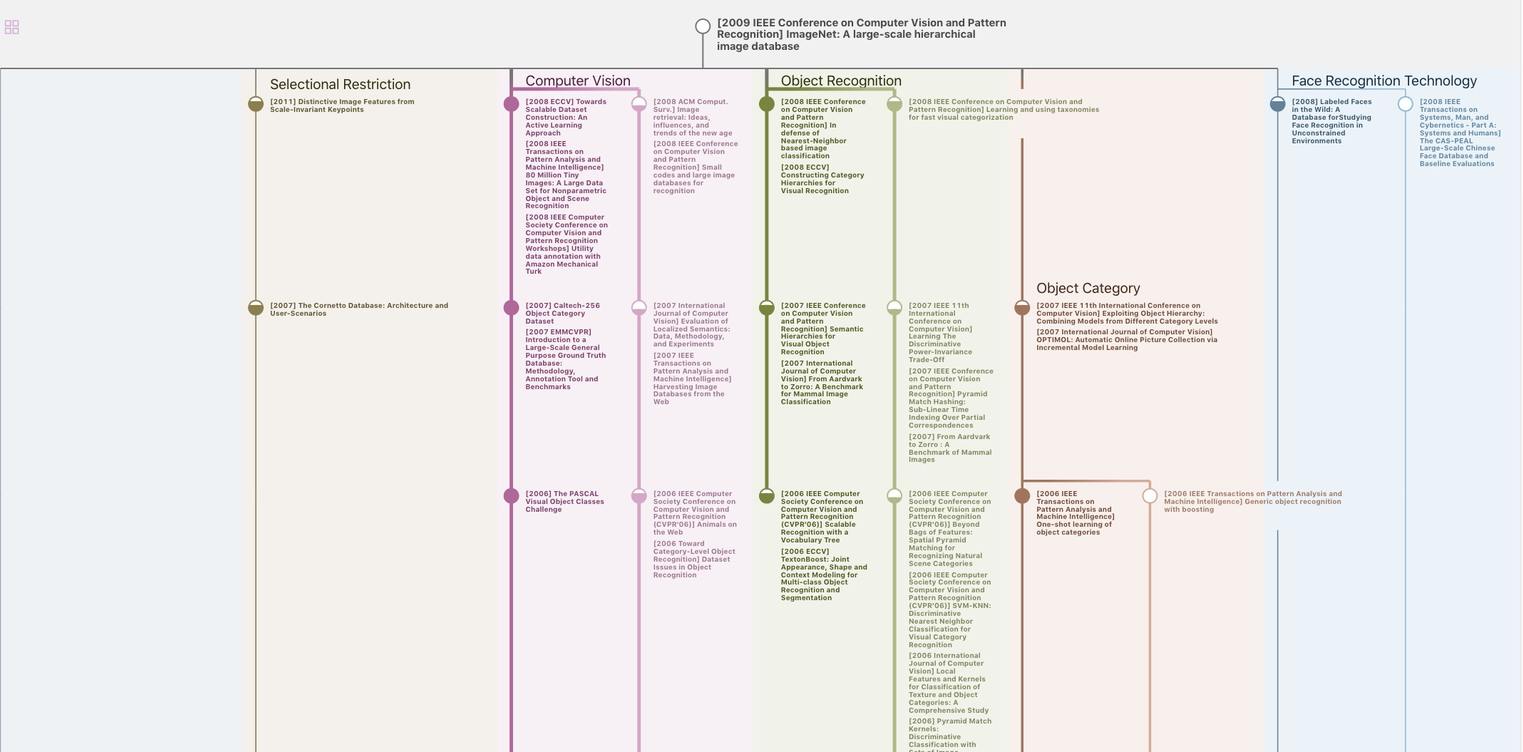
Generate MRT to find the research sequence of this paper
Chat Paper
Summary is being generated by the instructions you defined