A Convolutional Neural Network to Reconstruct Ocean Surface PAR from Physical Predictors
2024 International Conference on Artificial Intelligence, Computer, Data Sciences and Applications (ACDSA)(2024)
摘要
A deep learning Convolutional Neural Network (CNN) architecture is proposed to reconstruct time series of photosynthetically available radiation (PAR) at the ocean surface. The CNN is informed by the main physical drivers of PAR variability, i.e., cloud fractional coverage and optical thickness, which are obtained from satellite and/or reanalysis data. This is crucial to properly account for cloud effects in gap regions because they cannot be predicted confidently from the spatiotemporal structure of the data in gap-free regions. Setting randomly blocks of missing pixels in each training epoch and using the remaining observations as input proved to be effective. Missing data in satellite-derived daily mean PAR products (MODIS-Aqua) are filled accurately using the CNN, with a R
2
of 0.97, a RMSE of 3.22 E/m
2
/day (9.9%), and a small bias of 0.17 E/m
2
/day (0.5%). Comparisons between reconstructed PAR from MODIS and estimates with no gaps from EPIC show agreement in gap regions. The CNN also allows reconstruction of global PAR fields for the period prior to Earth observing satellites, enabling low-frequency variability studies. The study will yield long-term gapless PAR products that are critical for some data assimilating physical/biogeochemical models which need homogenized forcing fields and will help to assess the impact of the light field on aquatic ecosystems.
更多查看译文
关键词
Convolutional Neural Networks,photosynthetically available radiation,physical predictors,MODIS,EPIC,MERRA-2
AI 理解论文
溯源树
样例
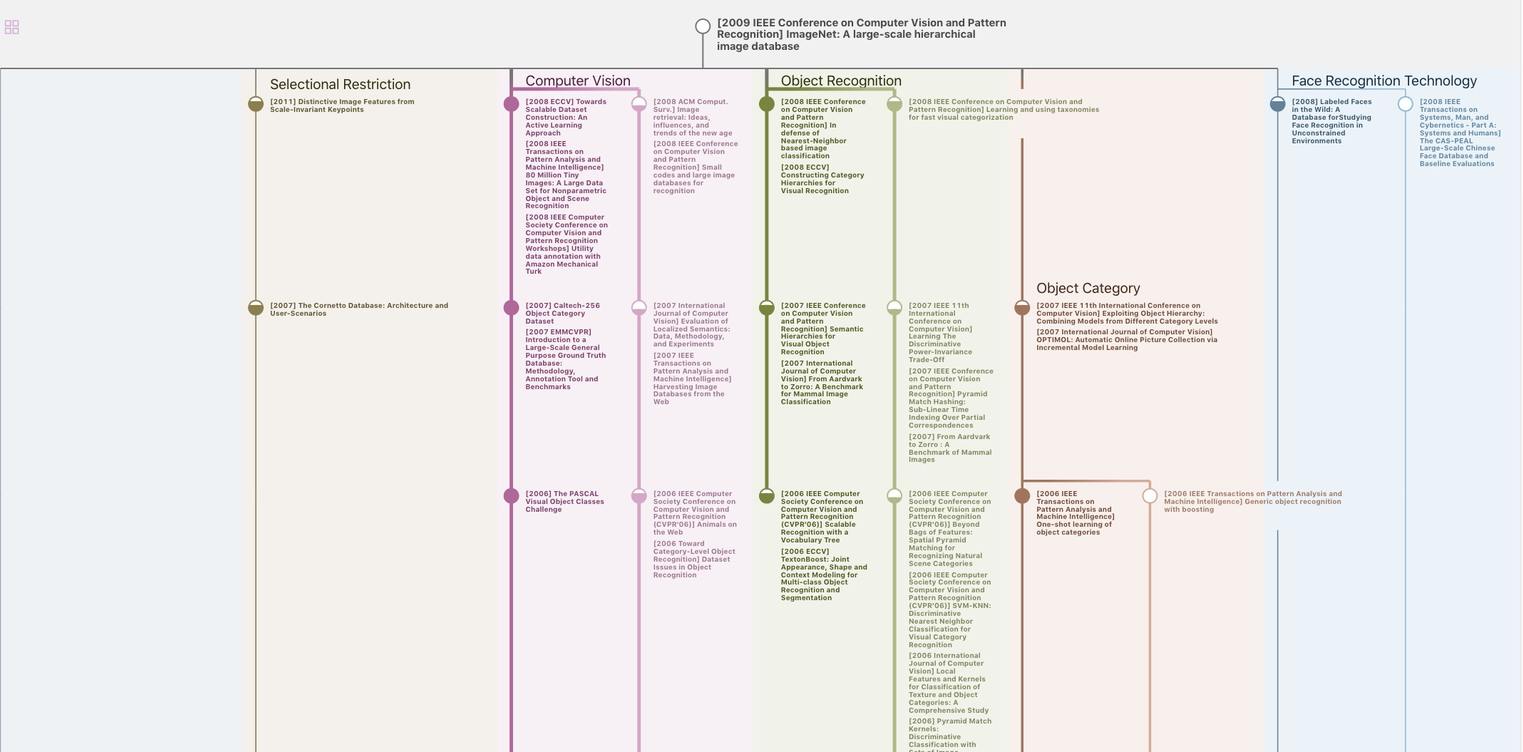
生成溯源树,研究论文发展脉络
Chat Paper
正在生成论文摘要