Federated Learning with Non Independent and Identically Distributed Data
2024 Conference of Young Researchers in Electrical and Electronic Engineering (ElCon)(2024)
摘要
The aim of this article is to study the effect of different Federated Learning strategies with several non-IID (Independent and Identically Distributed) data distributions on the test accuracy of the studied neural network model and compare it with the centralized training. In this work, we focus on implementation of three Flower framework algorithms with four non-IID data skews to conduct classification of dumpers cases. The experiments show that the test accuracy in centralized training was up to 85 % while in decentralized case was up to 81%.
更多查看译文
关键词
federated learning strategies,non-IID data distribution,flower framework,neural network model,classification,dumpers dataset
AI 理解论文
溯源树
样例
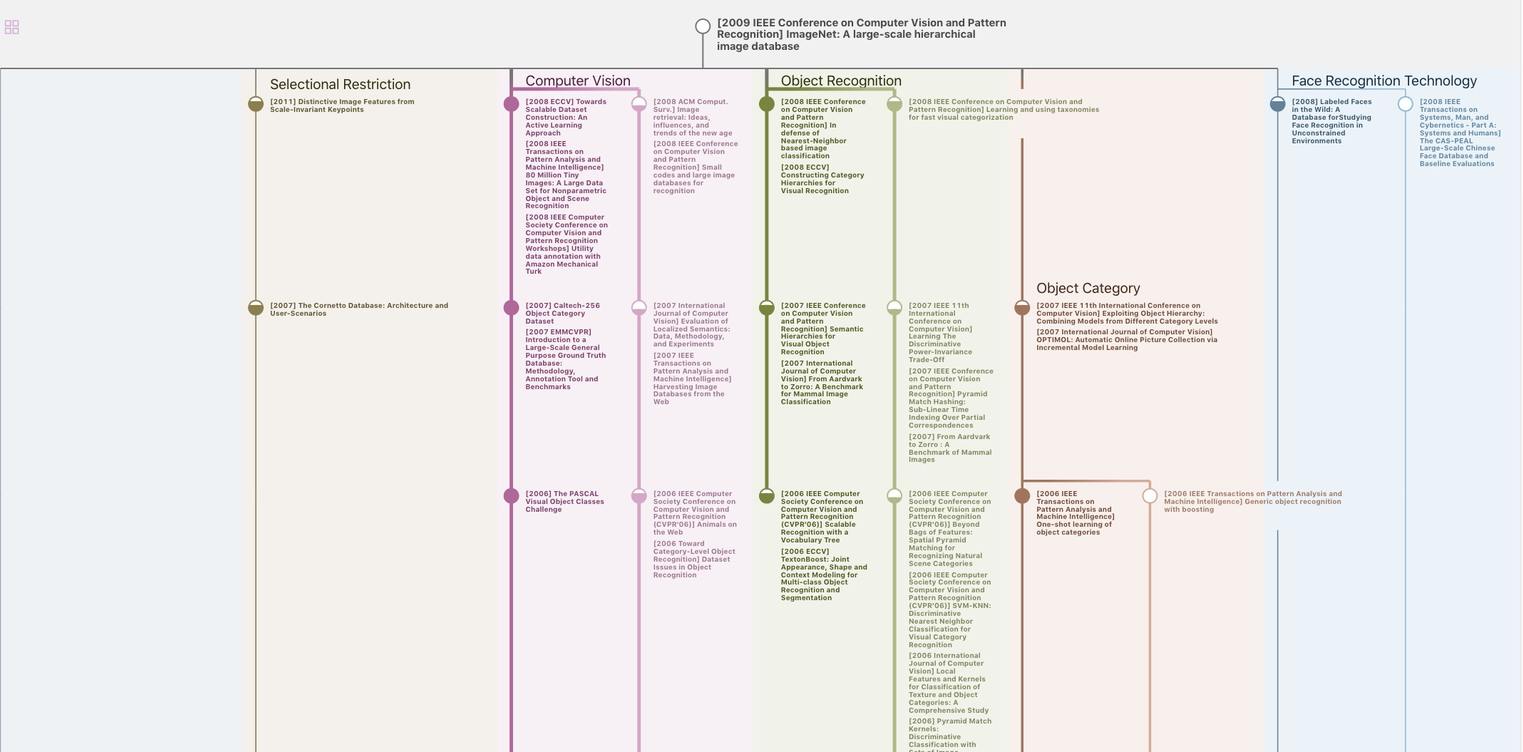
生成溯源树,研究论文发展脉络
Chat Paper
正在生成论文摘要