Adaptive Tie-Line Power Smoothing With Renewable Generation Based on Risk-Aware Reinforcement Learning
IEEE Transactions on Power Systems(2024)
摘要
The district cooling system (DCS) is a promising resource to smooth tie-line power fluctuations in a grid-connected microgrid with high-penetration renewable generation owing to its controllable large-scale loads and thermal inertia in buildings. However, due to complex system thermal dynamics, it is challenging to achieve precise model-based control of a DCS to cope with uncertain renewable generation. In this paper, a risk-aware reinforcement learning (RL) control framework is proposed for a DCS to achieve adaptive tie-line power smoothing. We first formulate the DCS control problem as a Constrained Markov Decision Process (CMDP). If the traditional RL is used to solve the CMDP, there is a high risk of frequent and extreme constraint violations during training due to random explorations. To effectively measure the risk of critical constraint violations, we introduce the conditional value-at-risk (CVaR), and reformulate the CMDP into a CVaR-based CMDP. We propose a risk-aware RL approach to solve the CVaR-based CMDP, which can improve the robustness of the obtained control strategy. Numerical case studies validate the effectiveness of the proposed method under the variation of renewable generation and power demands.
更多查看译文
关键词
Tie-line power smoothing,demand response,renewable generation,risk-aware reinforcement learning
AI 理解论文
溯源树
样例
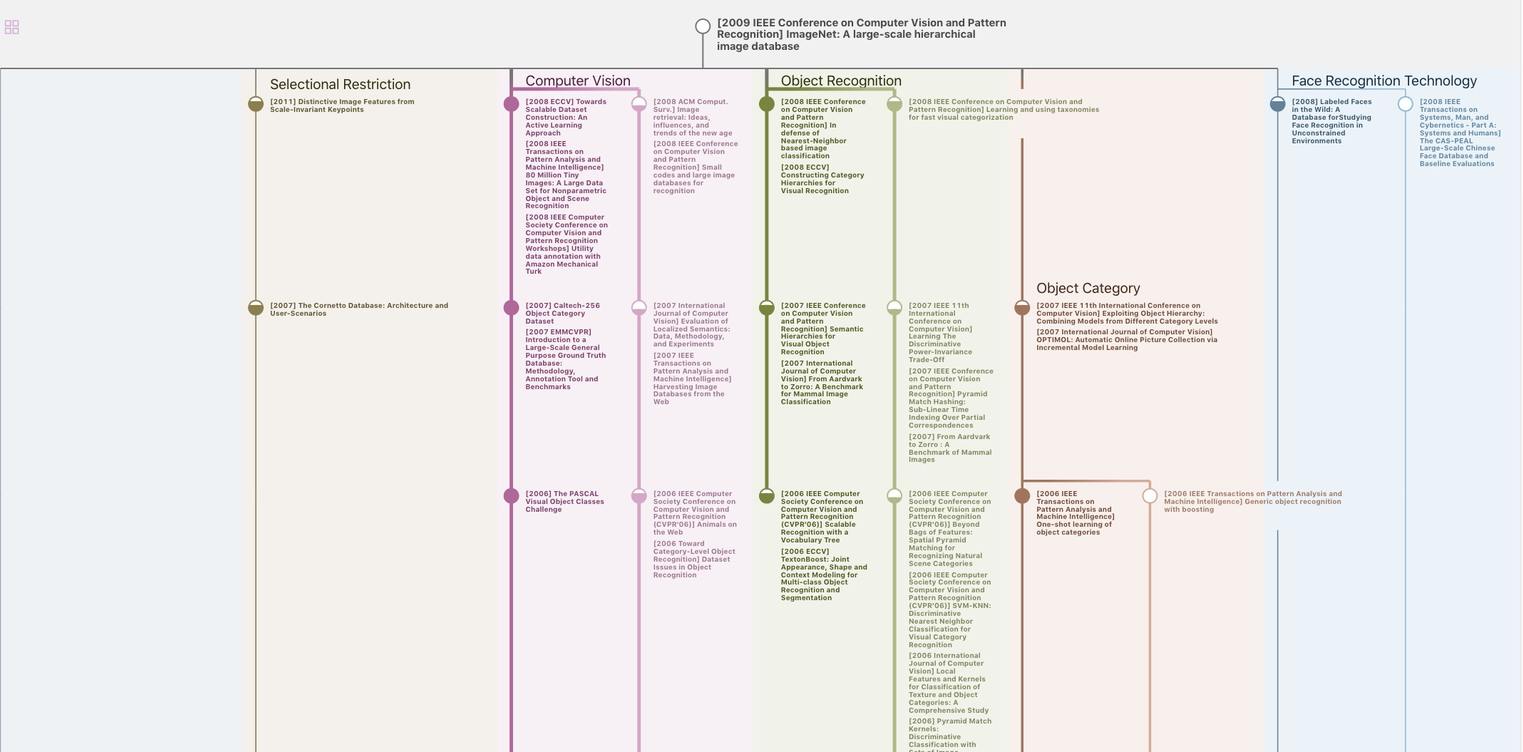
生成溯源树,研究论文发展脉络
Chat Paper
正在生成论文摘要