Threats, Attacks, and Defenses in Machine Unlearning: A Survey
CoRR(2024)
摘要
Recently, Machine Unlearning (MU) has gained considerable attention for its
potential to improve AI safety by removing the influence of specific data from
trained Machine Learning (ML) models. This process, known as knowledge removal,
addresses concerns about data such as sensitivity, copyright restrictions,
obsolescence, or low quality. This capability is also crucial for ensuring
compliance with privacy regulations such as the Right To Be Forgotten (RTBF).
Therefore, strategic knowledge removal mitigates the risk of harmful outcomes,
safeguarding against biases, misinformation, and unauthorized data
exploitation, thereby enhancing the ethical use and reliability of AI systems.
Efforts have been made to design efficient unlearning approaches, with MU
services being examined for integration with existing machine learning as a
service (MLaaS), allowing users to submit requests to erase data. However,
recent research highlights vulnerabilities in machine unlearning systems, such
as information leakage and malicious unlearning requests, that can lead to
significant security and privacy concerns. Moreover, extensive research
indicates that unlearning methods and prevalent attacks fulfill diverse roles
within MU systems. For instance, unlearning can act as a mechanism to recover
models from backdoor attacks, while backdoor attacks themselves can serve as an
evaluation metric for unlearning effectiveness. This underscores the intricate
relationship and complex interplay between these elements in maintaining system
functionality and safety. Therefore, this survey seeks to bridge the gap
between the extensive number of studies on threats, attacks, and defenses in
machine unlearning and the absence of a comprehensive review that categorizes
their taxonomy, methods, and solutions, thus offering valuable insights for
future research directions and practical implementations.
更多查看译文
AI 理解论文
溯源树
样例
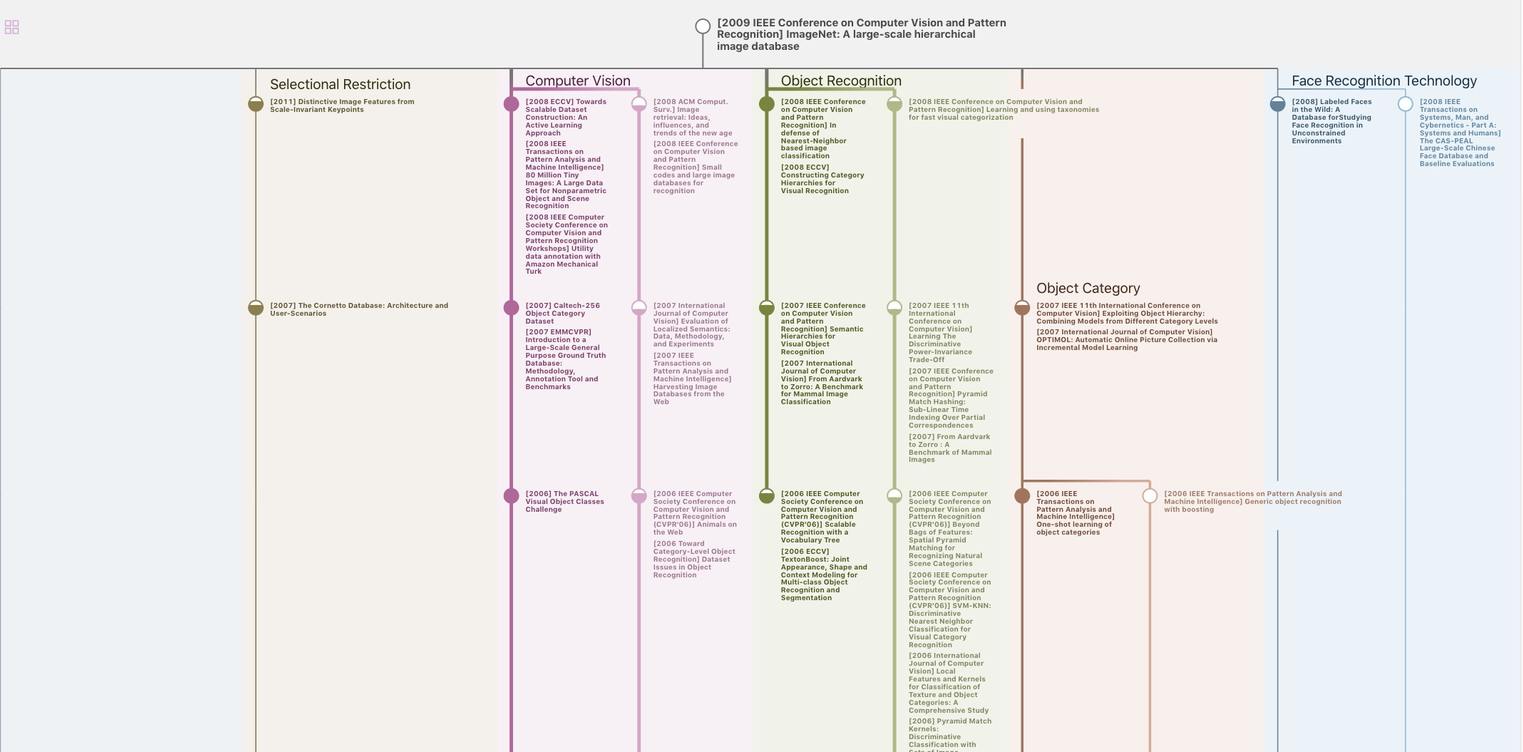
生成溯源树,研究论文发展脉络
Chat Paper
正在生成论文摘要