IndiTag: An Online Media Bias Analysis and Annotation System Using Fine-Grained Bias Indicators
arxiv(2024)
摘要
In the age of information overload and polarized discourse, understanding
media bias has become imperative for informed decision-making and fostering a
balanced public discourse. This paper presents IndiTag, an innovative online
media bias analysis and annotation system that leverages fine-grained bias
indicators to dissect and annotate bias in digital content. IndiTag offers a
novel approach by incorporating large language models, bias indicator, vector
database to automatically detect and interpret bias. Complemented by a
user-friendly interface facilitating both automated bias analysis and manual
annotation, IndiTag offers a comprehensive platform for in-depth bias
examination. We demonstrate the efficacy and versatility of IndiTag through
experiments on four datasets encompassing news articles from diverse platforms.
Furthermore, we discuss potential applications of IndiTag in fostering media
literacy, facilitating fact-checking initiatives, and enhancing the
transparency and accountability of digital media platforms. IndiTag stands as a
valuable tool in the pursuit of fostering a more informed, discerning, and
inclusive public discourse in the digital age. The demonstration video can be
accessed from https://youtu.be/Gt2T4T7DYqs. We release an online system for end
users and the source code is available at https://github.com/lylin0/IndiTag.
更多查看译文
AI 理解论文
溯源树
样例
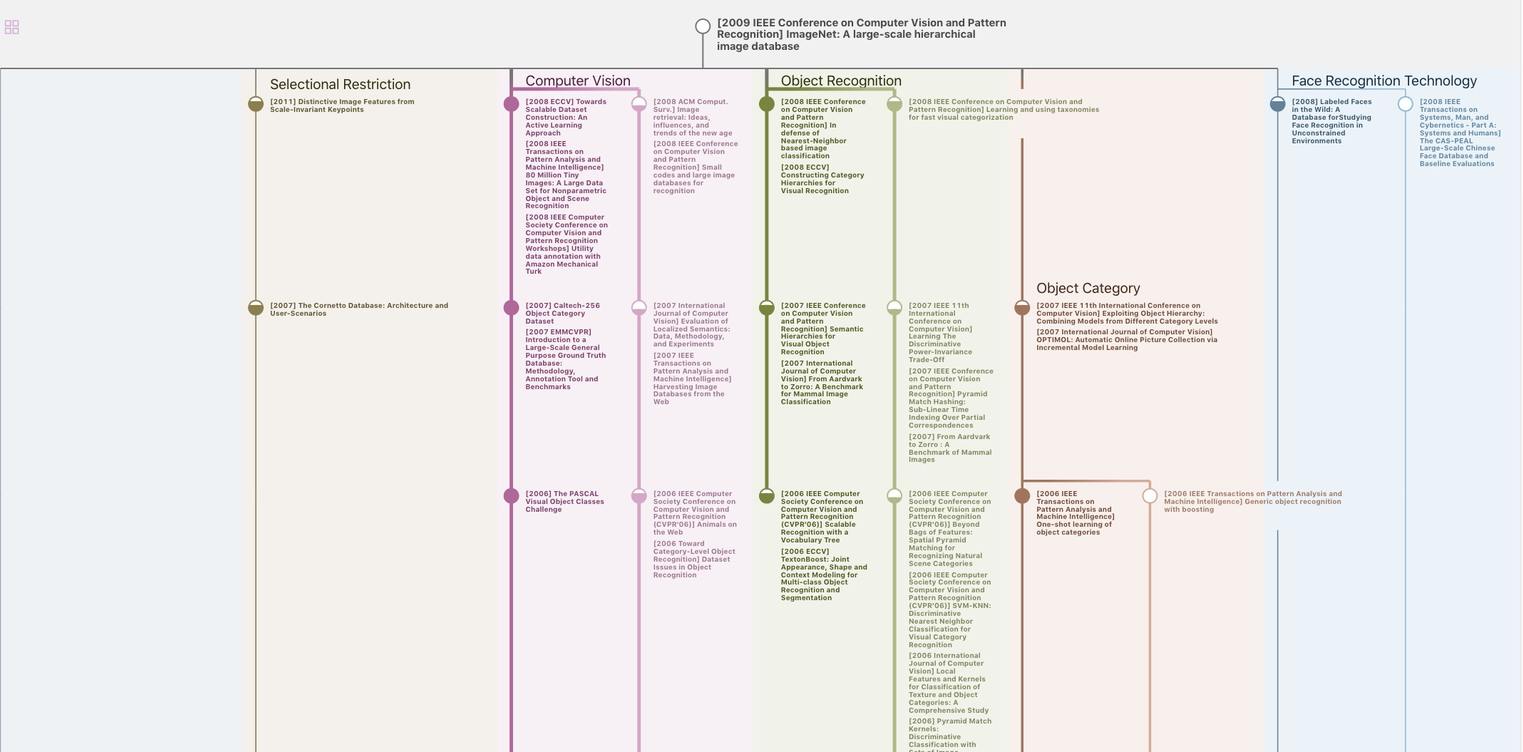
生成溯源树,研究论文发展脉络
Chat Paper
正在生成论文摘要