Data-model-interactive enhancement-based Francis turbine unit health condition assessment using graph driven health benchmark model
Expert Systems with Applications(2024)
摘要
As the data-driven Francis turbine units (FTUs) deterioration assessment method is widely investigated, the data quality in actual industrial scene has become an important prerequisite to restrict the method performance. However, existing data augmentation methods often lie in simply mixing real data and simulated data, ignoring the inherent cross-domain state relationships. In this paper, a data-model-interactive enhancement-based FTU health condition assessment using graph driven health benchmark model (HBM) is proposed. First, the pseudo-signals outputs of the mechanism digital twin (DT) model by calculative fluid dynamics (CFD) calculation are modified by critical working condition parameters, extending the theoretical assessment domain. To achieve data-model-interaction through cross-domain state coordination of digital and real data, a digital-reality hybrid graph is constructed from nodes mixing both actual and pseudo through node similarity. The hybrid spatial condition structure created by capture node status association explicitly enhances the sample representation ability. Avoiding excess noise effects, a knowledge-based unsupervised graph pruning regulation is adopted to refine the original graph considering noise and data reality. Finally, the spatial–temporal dependencies hidden in the refined graphs are mined by the designed hybrid graph neural network-based HBM, and output the assessed degradation label values. Verification experiments show that the proposed assessment method can effectively fit FTU deterioration under low-quality onsite data, and is more robust than the comparison method.
更多查看译文
关键词
Francis turbine units,Deterioration assessment,Data-model-interactive,Graph representation learning,Data enhancement
AI 理解论文
溯源树
样例
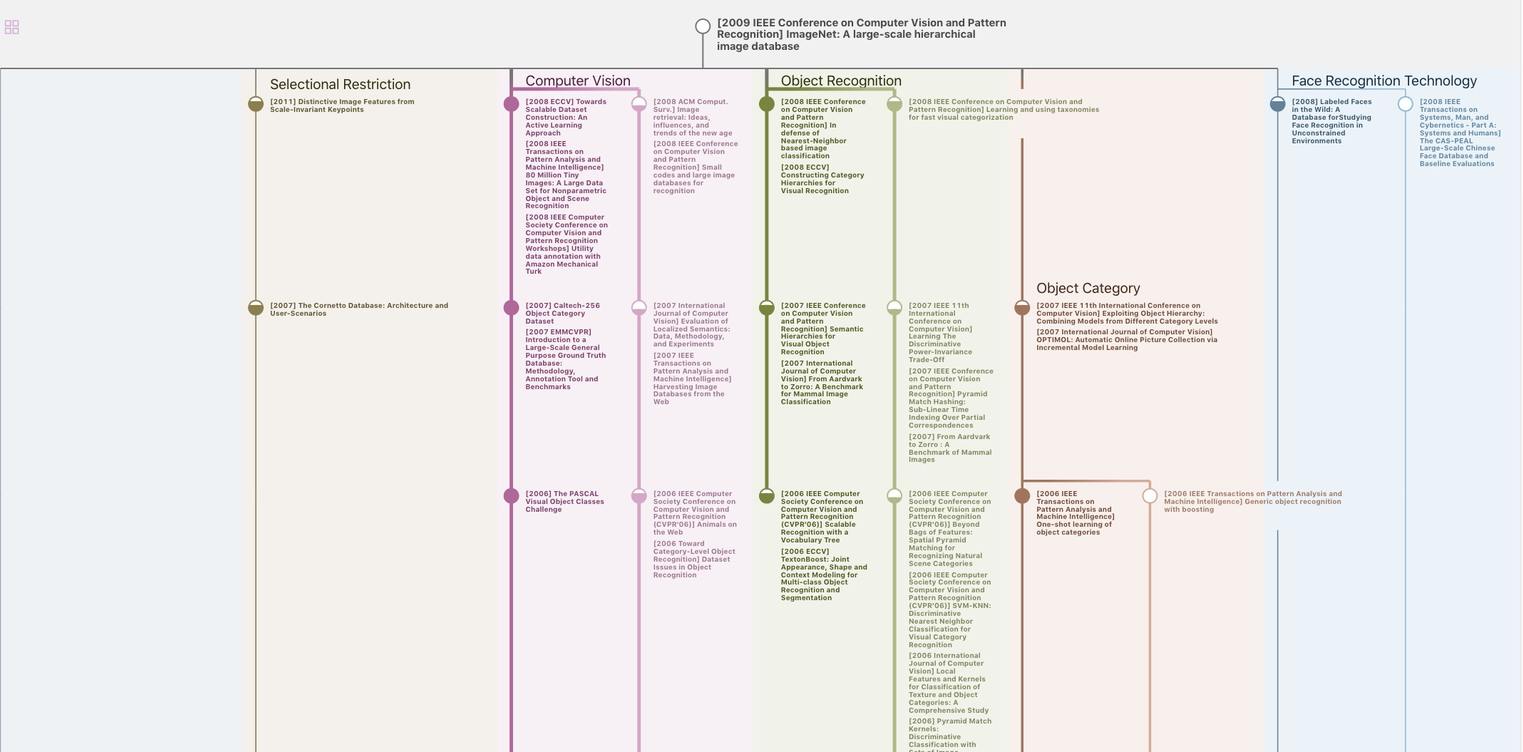
生成溯源树,研究论文发展脉络
Chat Paper
正在生成论文摘要