Crack _PSTU: Crack detection based on the U-Net framework combined with Swin Transformer
STRUCTURES(2024)
摘要
Cracks serve as a significant indicator of aging infrastructure. While the surfaces of traditional infrastructures are visually inspected manually, this approach is labor-intensive, highly subjective, and often surpasses the capabilities of available inspection personnel. Although some researchers have employed traditional image processing and machine learning techniques to address these challenges, the presence of irregular crack shapes, complex lighting conditions, and limitations in detecting only a single type of surface crack complicate automatic crack detection. Recent research indicates that deep learning methods are increasingly leading in image-based feature extraction, object detection, and pixel attribute analysis. This paper introduces a method, Crack_PSTU (Pretrained Swin Transformer U-Net), which leverages the U-Net framework and Swin Transformer model for infrastructure crack classification and detection tasks. Specifically, we utilize a pre-trained Swin Transformer network as the encoder and implement the decoder using convolution, pooling, and other operations, creating a "U"-shaped model architecture. Our experiments encompassed 11,298 crack images from varied scenes, with 9603 designated for training and 1695 for validation. The findings reveal that our approach surpasses other algorithms for this dataset.
更多查看译文
关键词
U-Net,Swin Transformer,Deep learning,Transfer learning,Crack detection
AI 理解论文
溯源树
样例
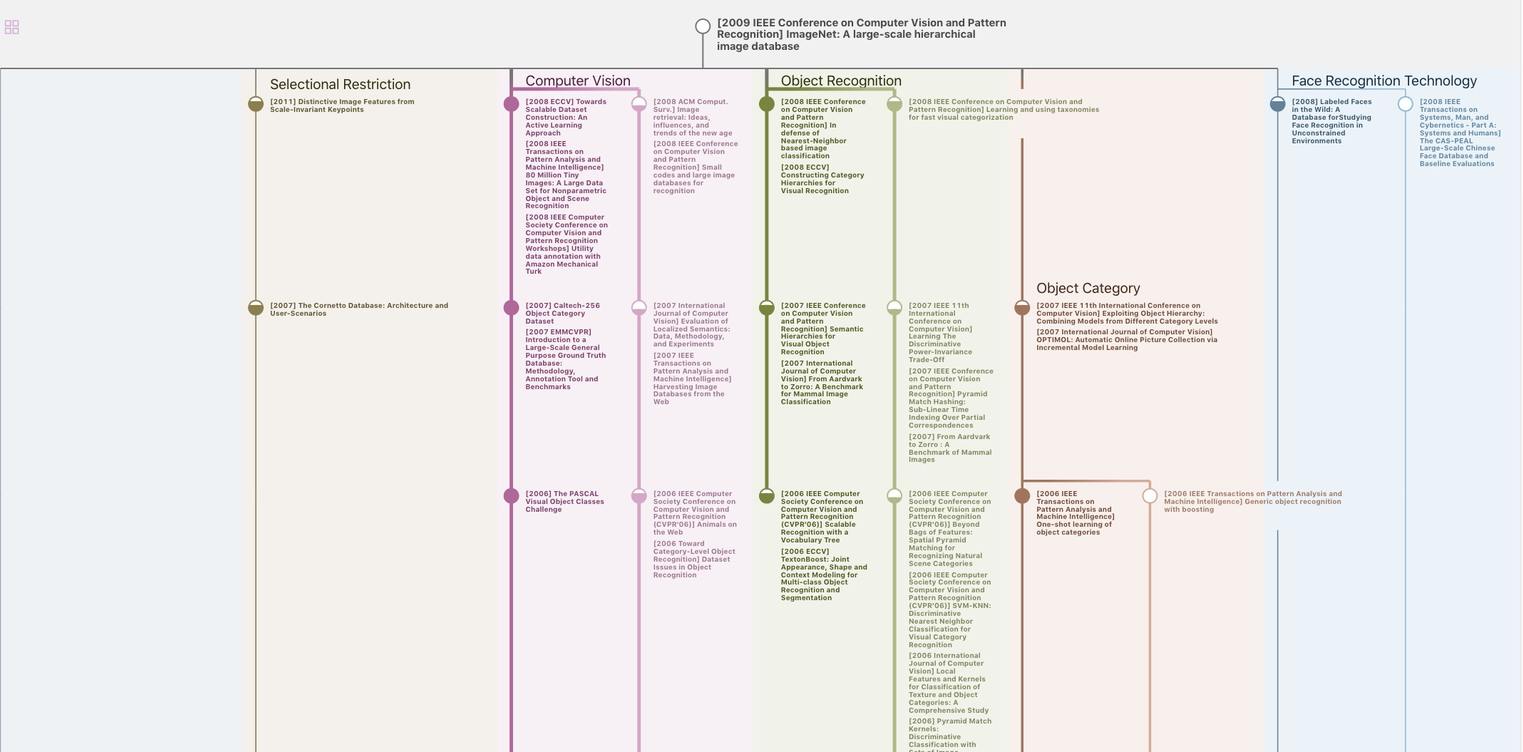
生成溯源树,研究论文发展脉络
Chat Paper
正在生成论文摘要