Style Adaptation module: Enhancing detector robustness to inter-manufacturer variability in surface defect detection
Computers in Industry(2024)
摘要
In recent years, deep learning-based approaches for industrial surface defect detection have shown great promise. To address the domain shift issue among data from different sources in the industrial domain, we present a novel plug-and-play Style Adaptation (SA) module, which endows the equipped defect detector with the capability to exhibit robustness to diverse styles present within the samples. This module effectively leverages datasets sourced from diverse origins while possessing congruent data types. In contrast to other domain adaptation approaches lacking well-defined domain delineations, the SA module generates representations characterized by distinct practical implications and precise mathematical formulations. Moreover, incorporating attention mechanisms reduces the need for manual intervention, allowing the module to focus autonomously on crucial branches in it. Experimental results demonstrate the superior efficacy of our approach compared to state-of-the-art techniques. Furthermore, an authentic dataset from various manufacturers is publicly available for deep learning research and industrial applications. Access the dataset at: https://github.com/THU-PMVAI/MTS3D
更多查看译文
关键词
Surface defect detection,Domain adaptation,Style transfer
AI 理解论文
溯源树
样例
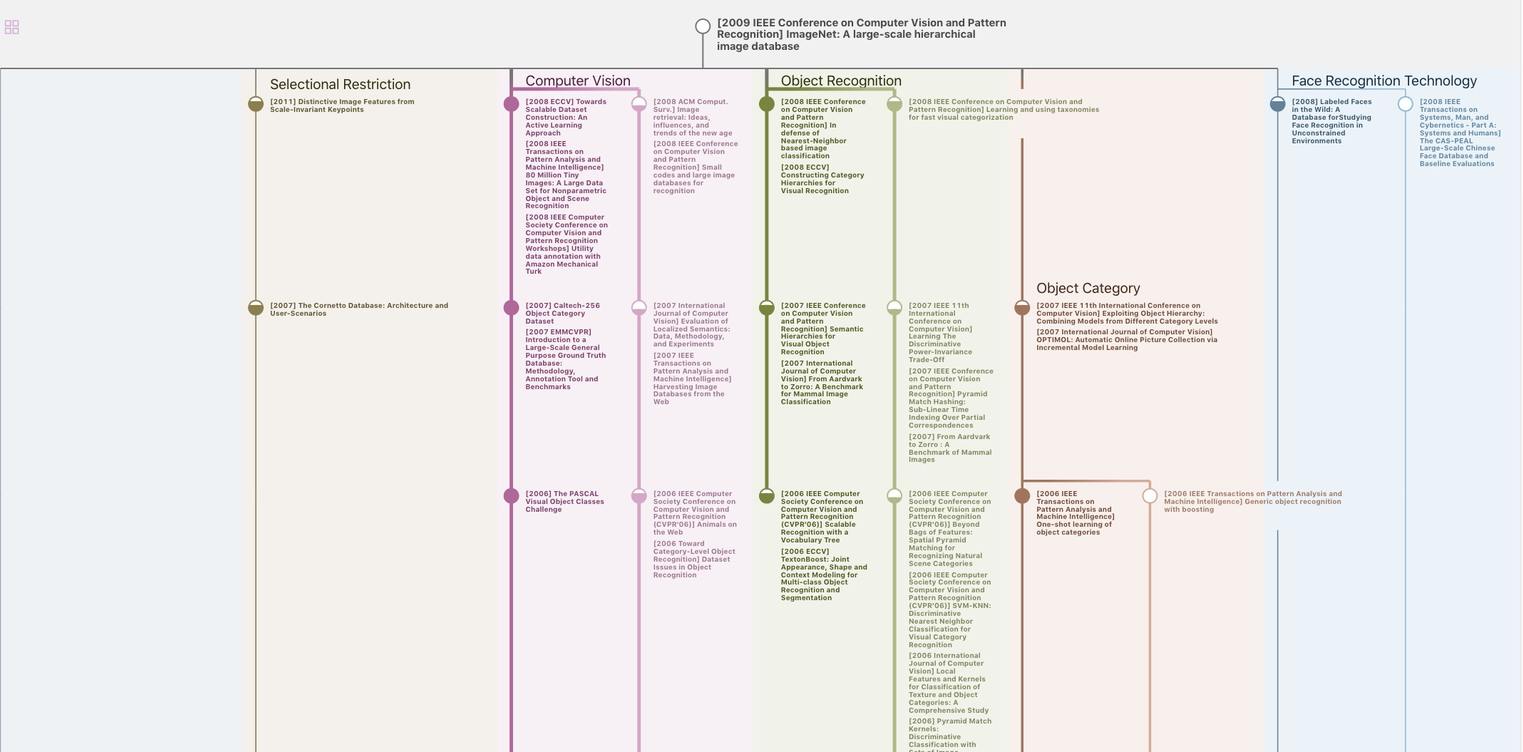
生成溯源树,研究论文发展脉络
Chat Paper
正在生成论文摘要