Fast, Quantization Aware DNN Training for Efficient HW Implementation.
Euromicro Symposium on Digital Systems Design(2023)
摘要
Quantization of Deep Neural Networks is a central technique to reduce the computation load in embedded devices. Even in quantized Deep Neural Networks (DNNs), the scaler/rescaler following a convolution or dense layer often requires a high bit width multiplication and a shift. Previous work has proposed to remove the multiplier by restricting the quantization method. We propose a Quantisation Aware Training (QAT) approach, which explicitly models the rescaler during training, eliminating the limitations of quantization functions and achieving a 30–35% improvement in training time and a significant reduction in memory requirements compared to the state-of-the-art. GitHub: https://github.com/embedded-machine-learning/FastQATforPOTRescaler
更多查看译文
AI 理解论文
溯源树
样例
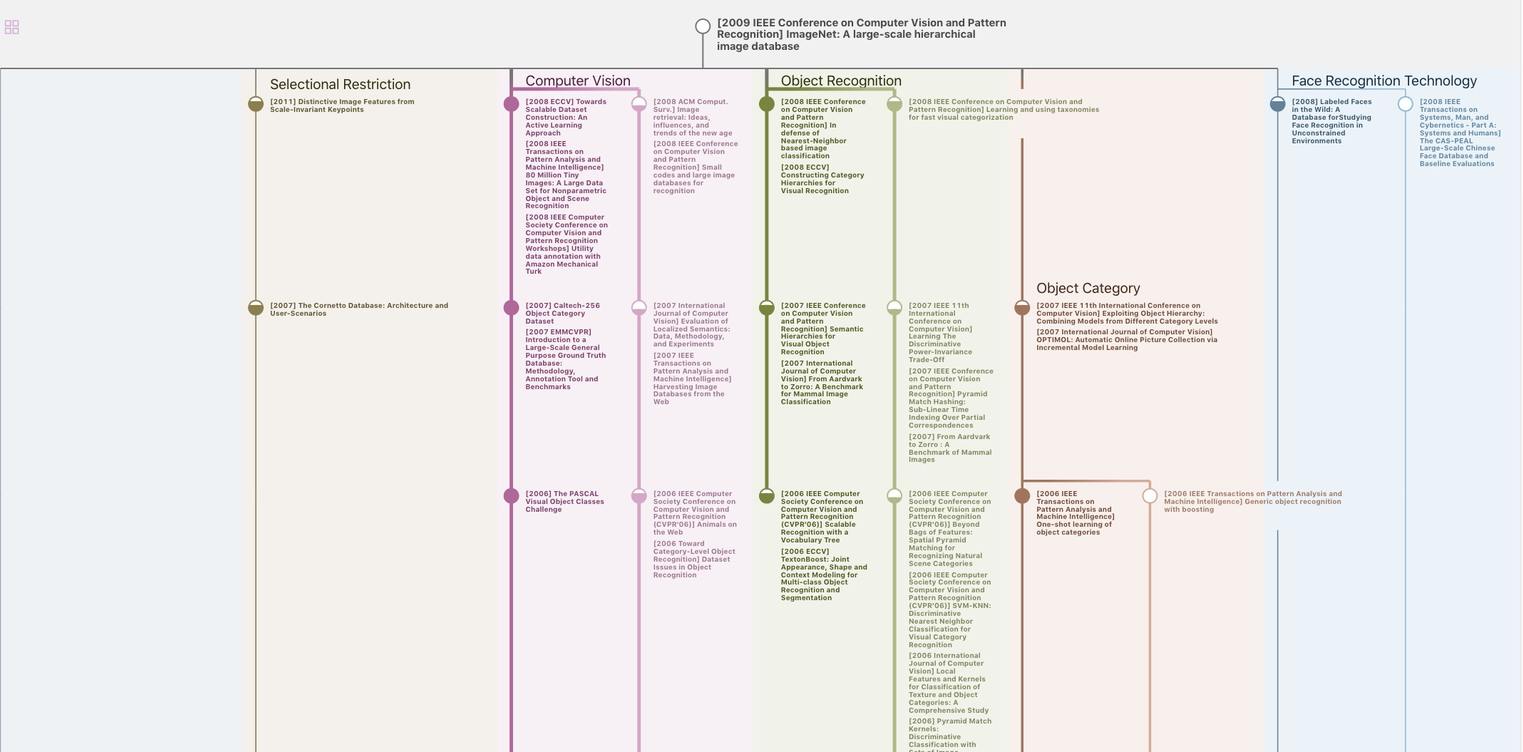
生成溯源树,研究论文发展脉络
Chat Paper
正在生成论文摘要