Optimizing Multi Commodity Flow Problem Under Uncertainty: A Deep Reinforcement Learning Approach.
International Conference on Machine Learning and Applications(2023)
摘要
We propose a deep reinforcement learning (DRL) approach to optimize the multi commodity flow problem under uncertainty. We frame the problem as a Markov decision problem (MDP) and develop a DRL agent that implements a Deep Q-Network (DQN) algorithm. The agent is trained via Q-Iearning iterations, and the Q-value function is modeled by a graph neural network (GNN) for value function approximation. The DRL agent is trained within a simulated environment to optimize the policy by minimizing costs, satisfying capacity constraints, and considering uncertain demands. We present the details of the environment and the DRL agent, the methodology, and a comparison of our approach with the traditional branch & bound algorithm. Our experimental results highlight the effectiveness and efficiency of the DRL approach in solving instances of the problem.
更多查看译文
AI 理解论文
溯源树
样例
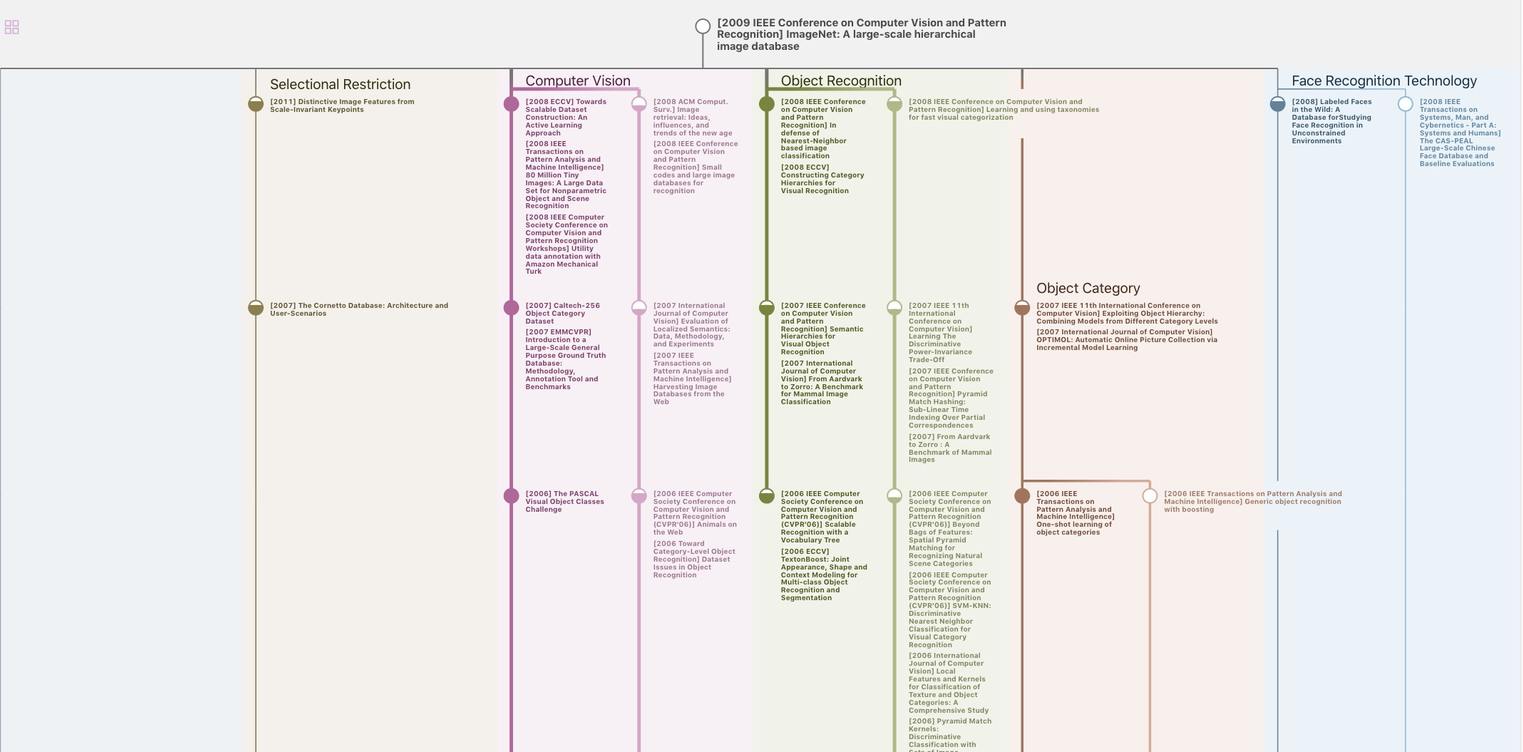
生成溯源树,研究论文发展脉络
Chat Paper
正在生成论文摘要