Novel Predictive-Reconstructive Feature Filtering LSTM-Based Autoencoder for Fault Diagnosis
2023 China Automation Congress (CAC)(2023)
摘要
Feature extraction plays a crucial role in data-driven fault diagnosis. To further extract features that better fit the needs of industrial process fault diagnosis, this paper proposes a predictive-reconstructive feature filtering LSTM-based Autoencoder (PRFFLAE) network. In PRFFLAE, LAE is utilized to predict the key performance indicators and reconstruct industrial process data for improving the accuracy of fault diagnosis. Additionally, permutation importance is introduced into PRLAE to select the feature related to fault diagnosis. Guided by the feature filtering ranking results based on key indicators for predicting performance, highly contributing features are selected to solve the challenge of evaluating the best fault diagnosis feature. The Tennessee-Eastman Process (TEP) is used to verify the performance of PRFFLAE. The simulation results indicate that PRFFLAE can effectively extract fault-related features and achieves better accuracy than other feature extraction methods.
更多查看译文
AI 理解论文
溯源树
样例
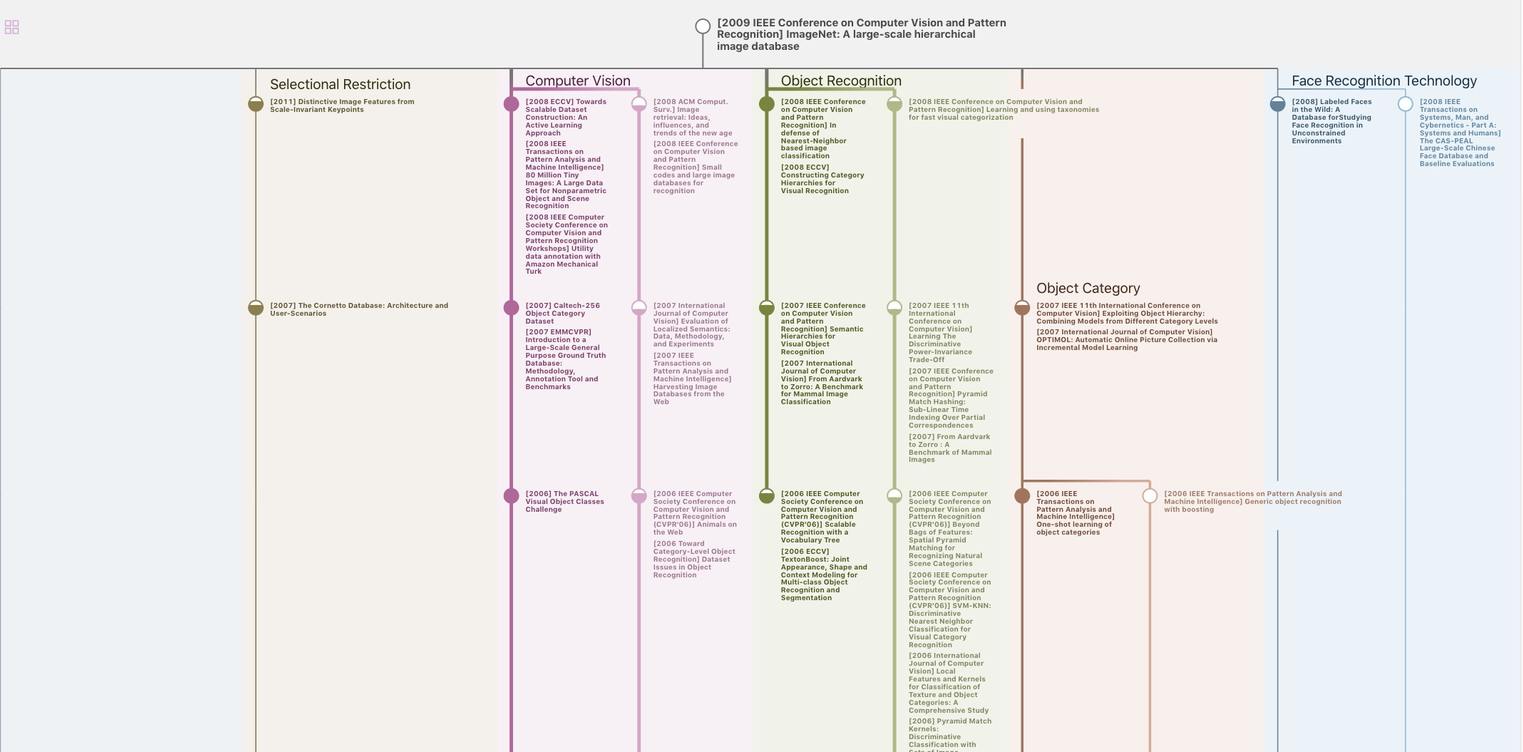
生成溯源树,研究论文发展脉络
Chat Paper
正在生成论文摘要