Contrast-enhanced CT-based deep radiomics strategy reveals the lymph node status in bladder cancer and its biological significance
crossref(2024)
Abstract
Abstract Purpose: To precisely evaluate bladder cancer (BCa) lymph node (LN) status, we proposed a deep radiomics model that combines local and global information on the contrast-enhanced (CECT) images, and investigated the biological significance of image features for LN metastasis prediction. Methods: Patients’ pretherapy CECT images with pathologically confirmed LN status of BCa were retrospectively collected from four clinical centers and publicly shared on TCIA. Radiomics models, deep learning models, and hybrid models integrating both radiomics and deep learning features with different classifiers were developed. Receiver operating characteristic analysis, Mann–Whitney U tests, and decision curve analysis (DCA) were used for diagnostic performance evaluation. Finally, weighted correlation network analysis and functional enrichment analysis were adopted to determine the biological pathways significantly associated with these image features. Results: Eighty patients were finally enrolled in this study. The hybrid model with support vector machine classifier obtained the best performance, with the sensitivity of 0.923 and area under the curve of 0.960 (95% CI: 0.884, 1.000) after 20-round 5-fold cross-validation, which significantly (p < 0.05) outperformed the other models for the LN metastasis prediction. DCA further showed great clinical benefit of the hybrid model for the prediction task. Additionally, Six biological pathways associated with immune inflammatory response, tumor transformation and lymphatic metastasis were significantly correlated with these image features for the LN status identification. Conclusion: The hybrid model could achieve favorable sensitivity and precision for BCa LN metastasis prediction. Key biological pathways were closely associated with image features in the hybrid model for LN metastasis prediction.
MoreTranslated text
AI Read Science
Must-Reading Tree
Example
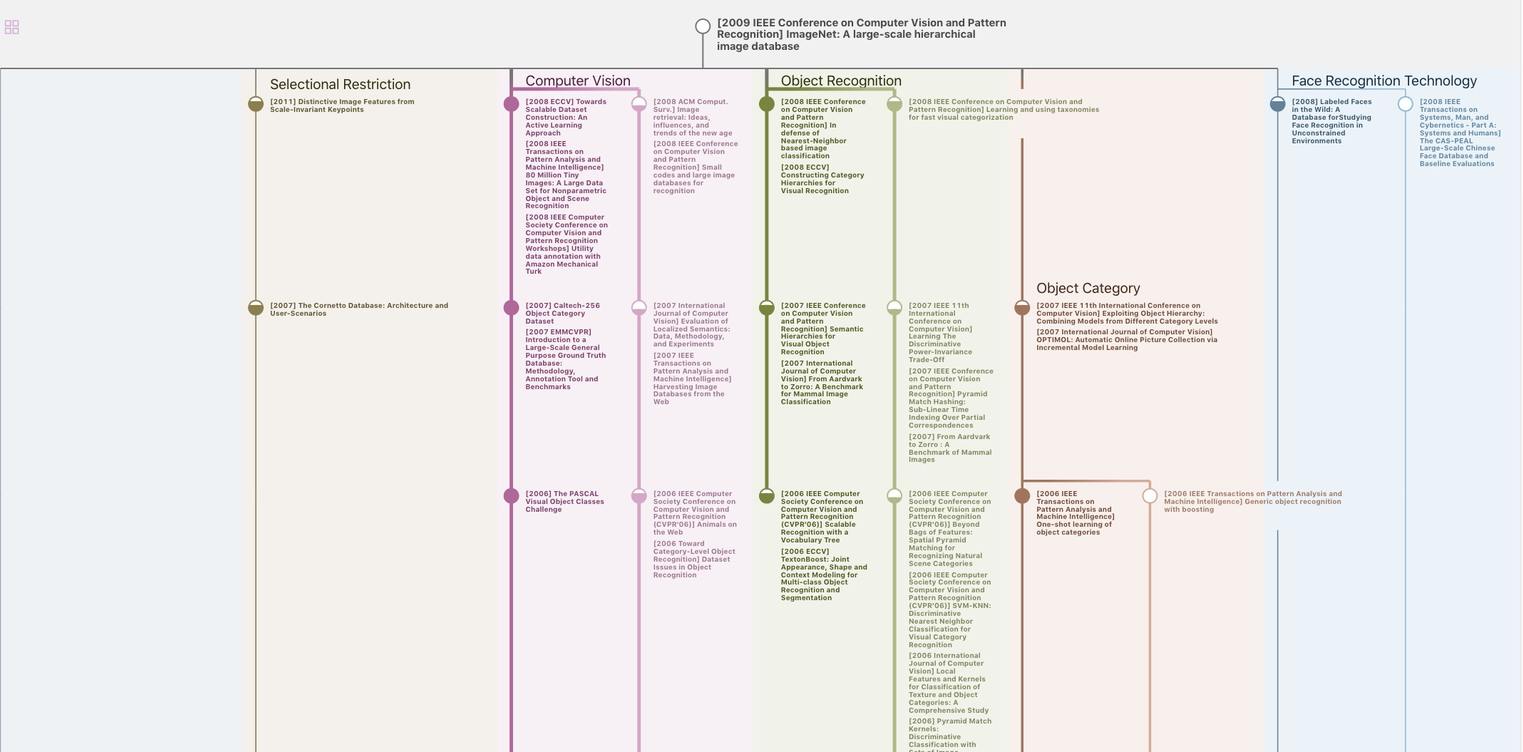
Generate MRT to find the research sequence of this paper
Chat Paper
Summary is being generated by the instructions you defined