Prediction of Traffic Flow Density and Velocity Based on Kalman Filter Fusion of The Non-Local Gas-Kinetic Model and Convolution Neural Networks-Long-Short Term Memory Model
2023 China Automation Congress (CAC)(2023)
摘要
The integrated perception, planning, and control of intelligent transportation system has become a research hotspot in academia. In this context, the safe running of an intelligent vehicle highly depends on the accurate prediction of its driving environment and working conditions. Traffic flow parameters represent the macro characteristics of an intelligent vehicle driving environment. Accurate prediction of traffic flow parameters is beneficial to improve the accuracy of trajectory prediction of vehicles in the surrounding environment. As two kinds of traffic flow parameter prediction models: the macroscopic traffic flow model and the data-based learning model have their own characteristics. To fully take advantage of these two models, our work proposes a study of the macroscopic traffic flow model based on a nonlocal gas-kinetic (GKT) and a deep learning model based on convolutional neural networks-long short term memory. Real data sets US-101 and PeMS are used respectively to predict traffic flow density and velocity. Finally, the Kalman filter is employed to fuse the results of the two models. The experiment shows that the prediction accuracy of traffic flow density and velocity can be improved as compared with the Macroscopic traffic flow model and the data-based learning model.
更多查看译文
AI 理解论文
溯源树
样例
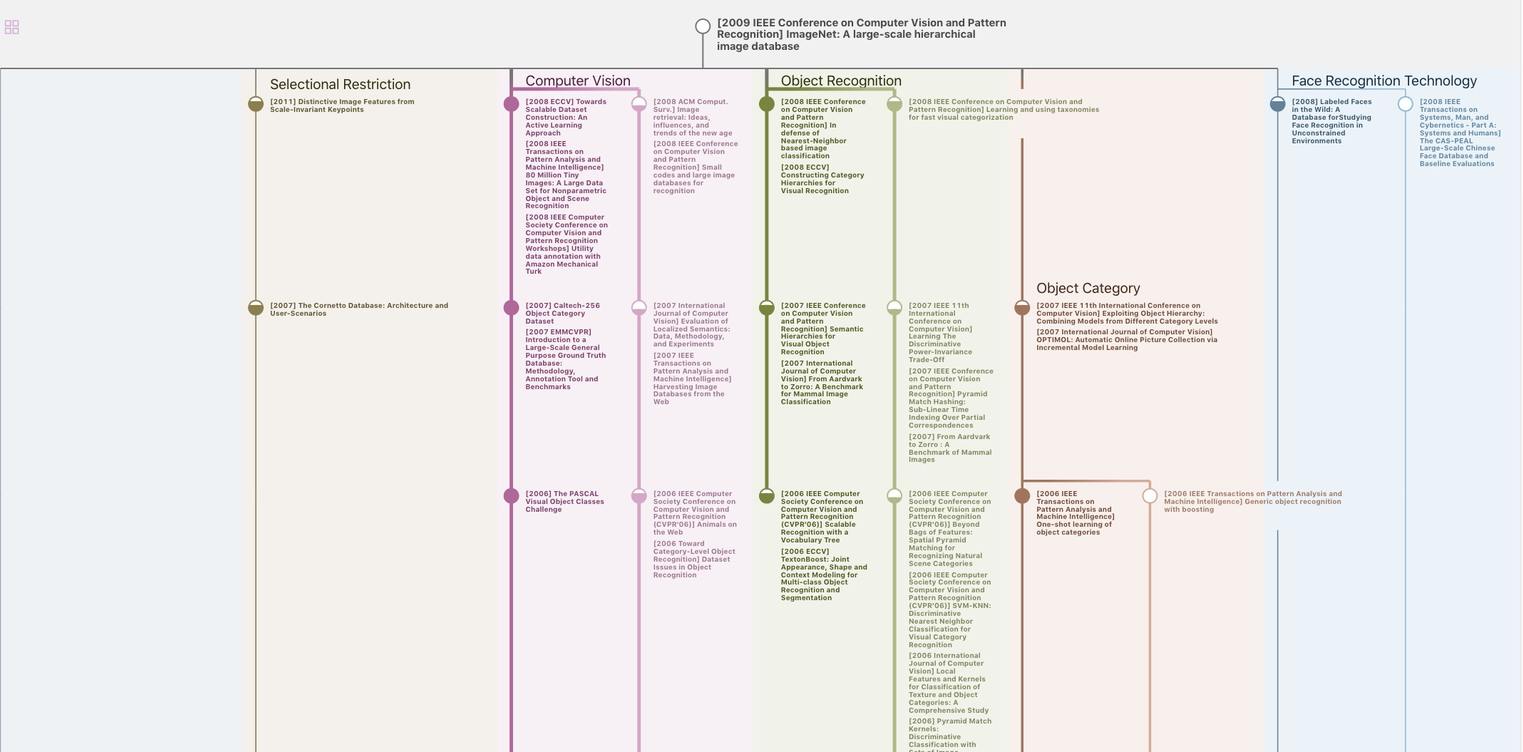
生成溯源树,研究论文发展脉络
Chat Paper
正在生成论文摘要