MDCN: A Dual-Branch Model Based on Difference Convolution for Non-Intrusive Load Disaggregation
2023 China Automation Congress (CAC)(2023)
摘要
Non-intrusive load disaggregation is one of the tasks of Non-intrusive Load Monitoring (NILM), aims to disaggregate appliances power from total power. Convolutional neural networks (CNNs) have shown excellent performance in NILM due to their powerful feature representation capabilities. However, when multiple appliances are working simultaneously, CNN models exhibit performance degradation and weak generalization ability. This is because the Power Superposition (PS) of multiple appliances is similar to shadows in images, which can cause information loss and interference. We propose a new model called Mix Difference Convolutional Network (MDCN) to improve the performance when PS occurs. MDCN is based on a dual-branch structure, and it performs supervised learning on both the power sequence and the on/off sequence of the target appliance. Experimental results demonstrate that compared to existing CNN models, MDCN achieves the best performance on the REDD and UK-DALE datasets, especially when PS occurs. In addition, ablation experiments were conducted to verify the effectiveness of the proposed difference convolution operators.
更多查看译文
AI 理解论文
溯源树
样例
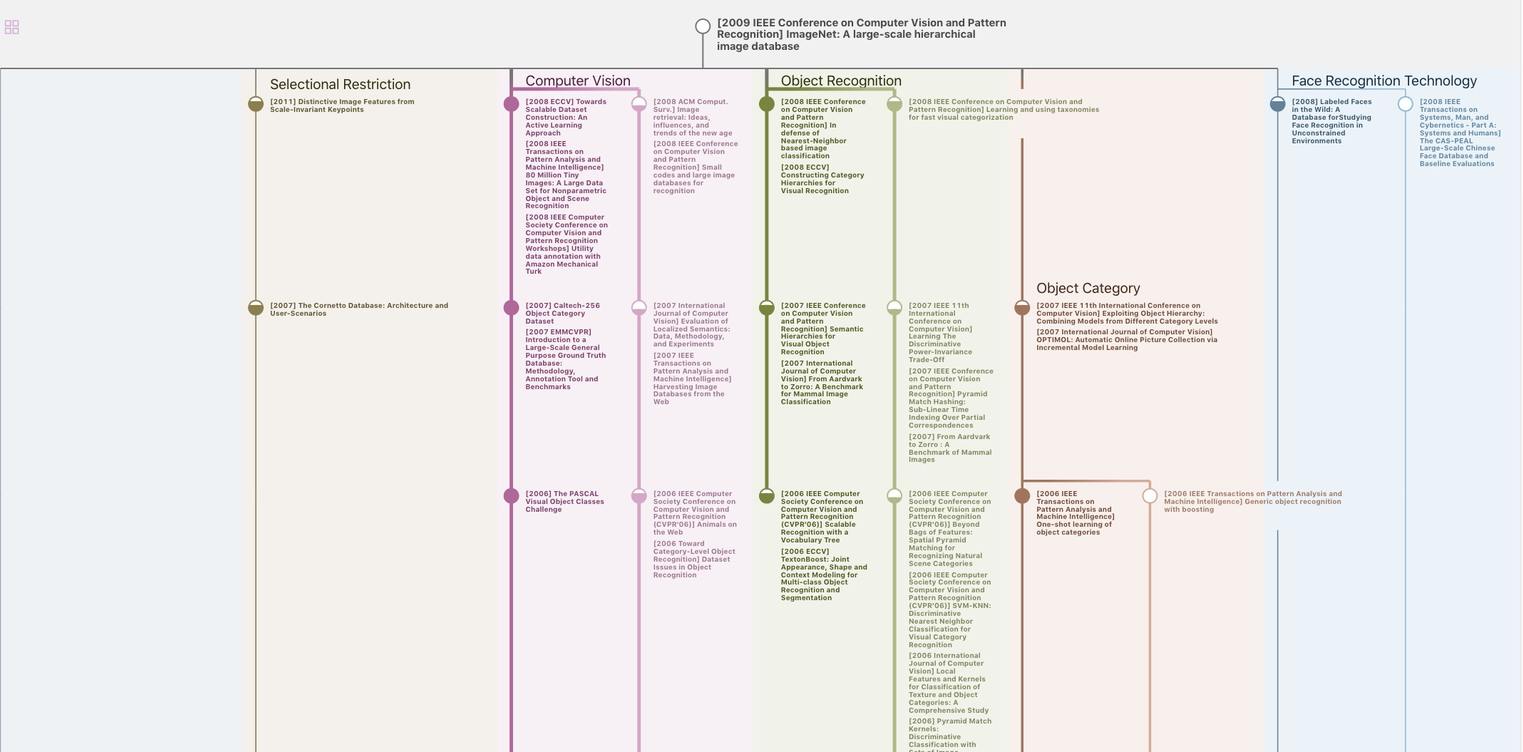
生成溯源树,研究论文发展脉络
Chat Paper
正在生成论文摘要