Content Selection in Abstractive Summarization with Biased Encoder Mixtures.
International Joint Conference on the Analysis of Images, Social Networks and Texts(2023)
摘要
Current abstractive summarization models consistently outperform extractive counterparts yet are unable to close the gap with Oracle extractive upper bound. Recent research suggests that the reason lies in the lack of planning and bad sentence-wise saliency intuition. Existing solutions to the problem either require new fine-tuning sessions to accommodate the architectural changes or disrupt the natural information flow limiting the utilization of accumulated global knowledge. Inspired by text-to-image result blending techniques we propose a plug-and-play alternative that preserves the integrity of the original model, Biased Encoder Mixture. Our approach utilizes attention masking and Siamese networks to reinforce the signal of salient tokens in encoder embeddings and guide the decoder to more relevant results. The evaluation on four datasets and their respective state-of-the-art abstractive summarization models demonstrate that Biased Encoder Mixture outperforms the attention-based plug-and-play alternatives even with static masking derived from sentence saliency positional distribution.
更多查看译文
AI 理解论文
溯源树
样例
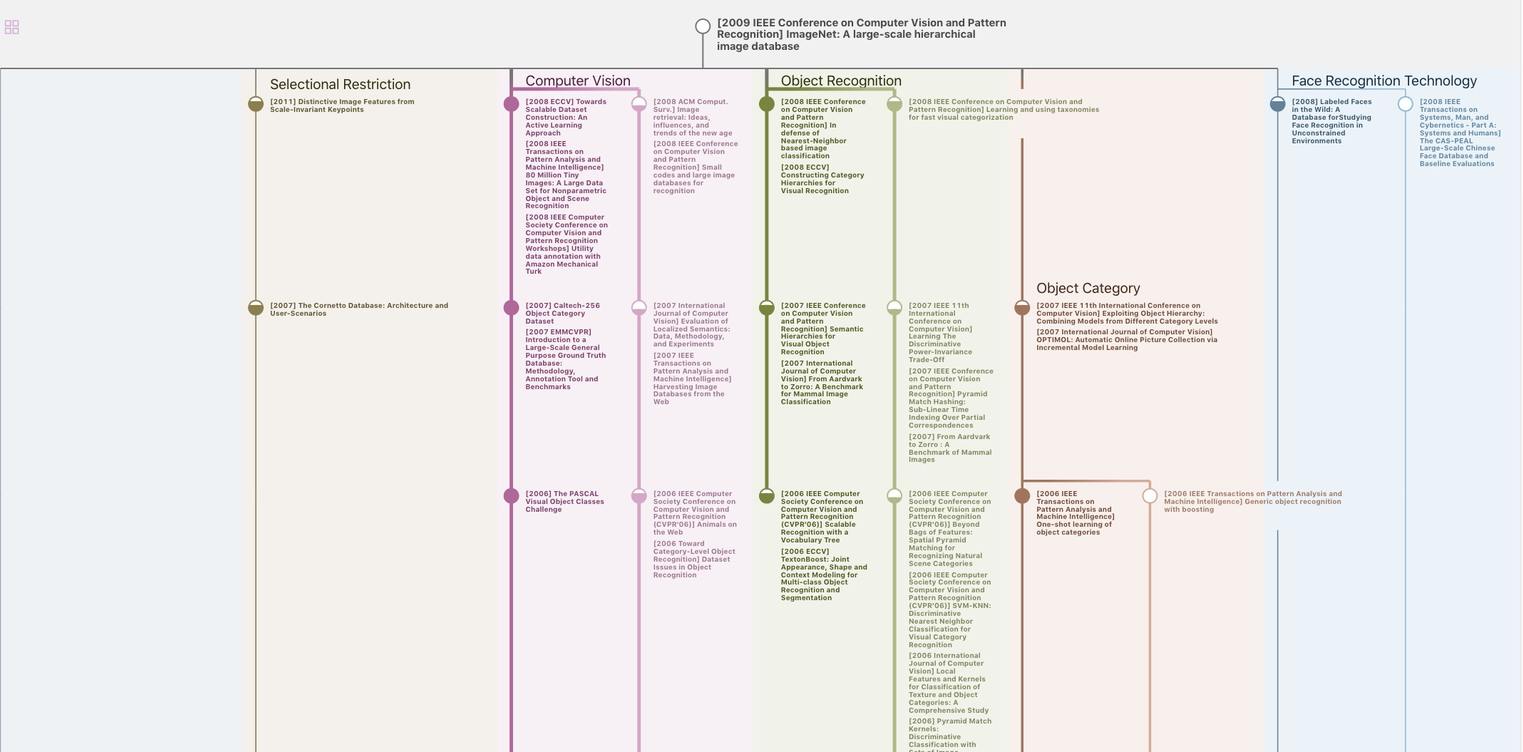
生成溯源树,研究论文发展脉络
Chat Paper
正在生成论文摘要