Multicenter Development and Prospective Validation of eCARTv5: A Gradient Boosted Machine Learning Early Warning Score.
medRxiv : the preprint server for health sciences(2024)
摘要
Rationale:Early detection of clinical deterioration using early warning scores may improve outcomes. However, most implemented scores were developed using logistic regression, only underwent retrospective internal validation, and were not tested in important patient subgroups.
Objectives:To develop a gradient boosted machine model (eCARTv5) for identifying clinical deterioration and then validate externally, test prospectively, and evaluate across patient subgroups.
Methods:All adult patients hospitalized on the wards in seven hospitals from 2008- 2022 were used to develop eCARTv5, with demographics, vital signs, clinician documentation, and laboratory values utilized to predict intensive care unit transfer or death in the next 24 hours. The model was externally validated retrospectively in 21 hospitals from 2009-2023 and prospectively in 10 hospitals from February to May 2023. eCARTv5 was compared to the Modified Early Warning Score (MEWS) and the National Early Warning Score (NEWS) using the area under the receiver operating characteristic curve (AUROC).
Measurements and Main Results:The development cohort included 901,491 admissions, the retrospective validation cohort included 1,769,461 admissions, and the prospective validation cohort included 46,330 admissions. In retrospective validation, eCART had the highest AUROC (0.835; 95%CI 0.834, 0.835), followed by NEWS (0.766 (95%CI 0.766, 0.767)), and MEWS (0.704 (95%CI 0.703, 0.704)). eCART's performance remained high (AUROC ≥0.80) across a range of patient demographics, clinical conditions, and during prospective validation.
Conclusions:We developed eCARTv5, which accurately identifies early clinical deterioration in hospitalized ward patients. Our model performed better than the NEWS and MEWS retrospectively, prospectively, and across a range of subgroups.
更多查看译文
AI 理解论文
溯源树
样例
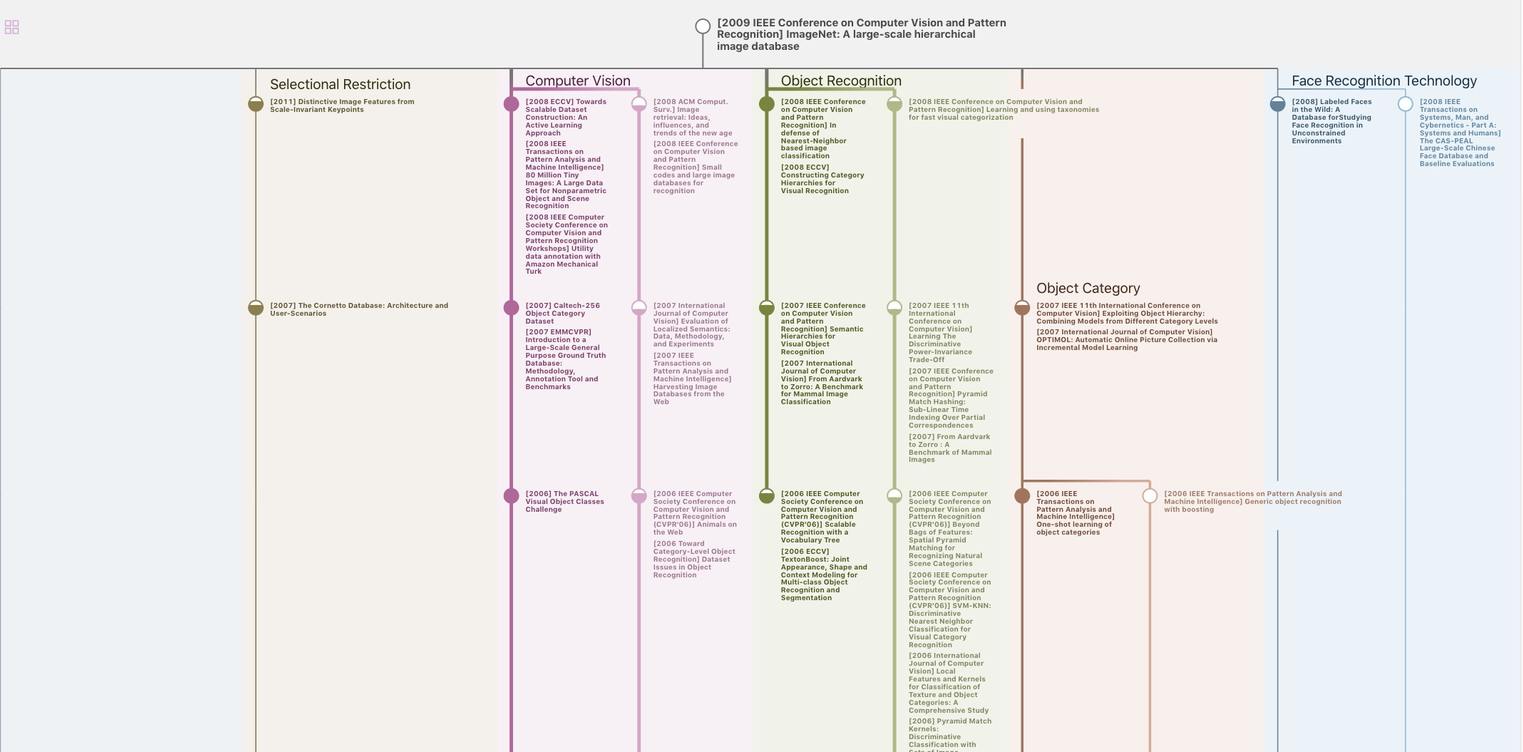
生成溯源树,研究论文发展脉络
Chat Paper
正在生成论文摘要