TTS: Hilbert Transform-based Generative Adversarial Network for Tattoo and Scene Text Spotting
IEEE Transactions on Multimedia(2024)
摘要
Text spotting in natural scenes is of increasing interest and significance due to its critical role in several applications, such as visual question answering, named entity recognition and event rumor detection on social media. One of the newly emerging challenging problems is Tattoo Text Spotting (TTS) in images for assisting forensic teams and for person identification. Unlike the generally simpler scene text addressed by current state-of-the-art methods, tattoo text is typically characterized by the presence of decorative backgrounds, calligraphic handwriting and several distortions due to the deformable nature of the skin. This paper describes the first approach to address TTS in a real-world application context by designing an end-to-end text spotting method employing a Hilbert transform-based Generative Adversarial Network (GAN). To reduce the complexity of the TTS task, the proposed approach first detects fine details in the image using the Hilbert transform and the Optimum Phase Congruency (OPC). To overcome the challenges of only having a relatively small number of training samples, a GAN is then used for generating suitable text samples and descriptors for text spotting (i.e. both detection and recognition). The superior performance of the proposed TTS approach, for both tattoo and general scene text, over the state-ofthe-art methods is demonstrated on a new TTS-specific dataset (publicly available1) as well as on the existing benchmark natural scene text datasets: Total-Text, CTW1500 and ICDAR 2015.
更多查看译文
关键词
Hilbert transform,Text detection,Text spotting,Generative adversarial networks,Calligraphic text,Tattoo text spotting
AI 理解论文
溯源树
样例
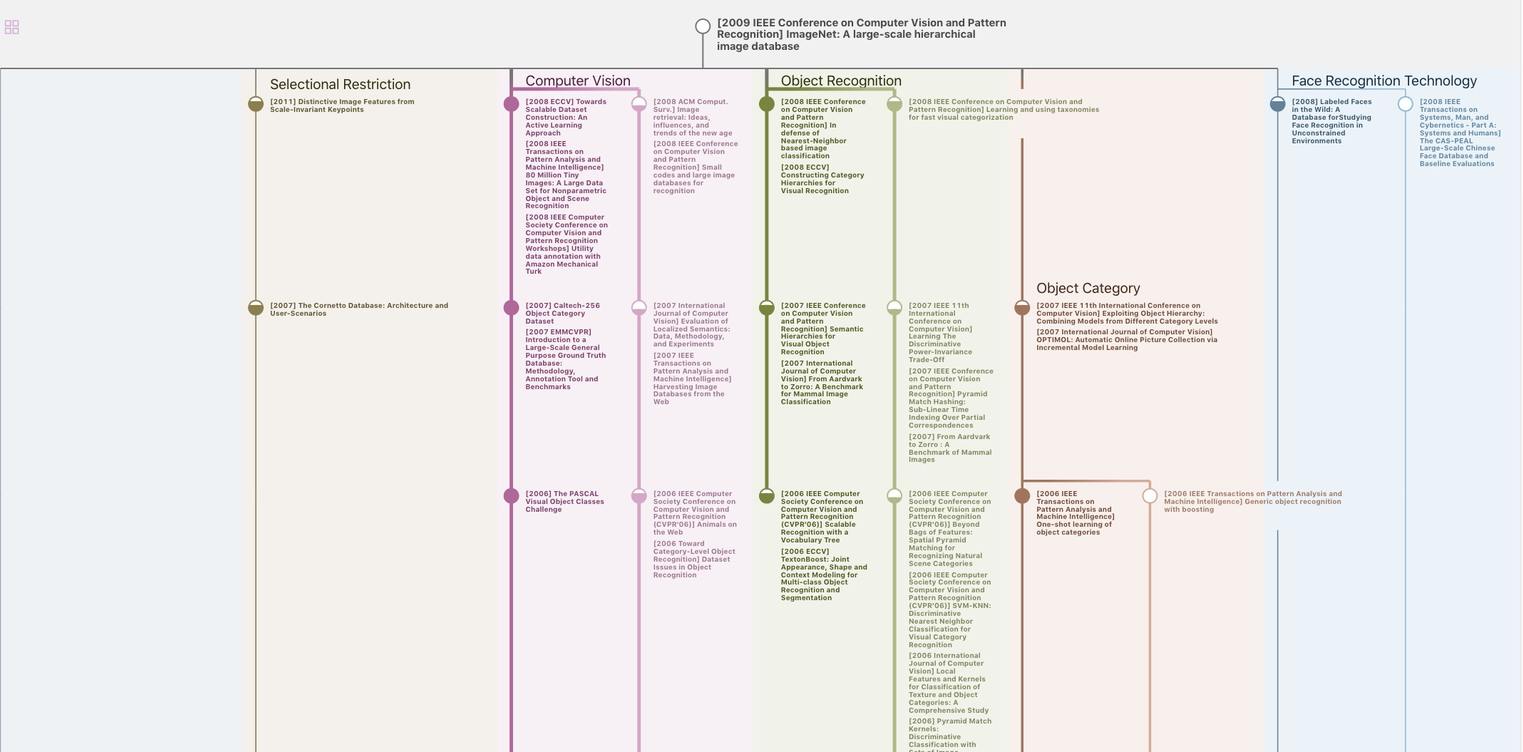
生成溯源树,研究论文发展脉络
Chat Paper
正在生成论文摘要