Embedding Secret Message in Chinese Characters via Glyph Perturbation and Style Transfer
IEEE TRANSACTIONS ON INFORMATION FORENSICS AND SECURITY(2024)
Abstract
Glyph perturbation adjusts the characters' structures and strokes to make the original characters change subtly, which cannot be detected by the naked eye. These generated variants with different glyph perturbation can represent different status of secret messages, which can be used to embed information in Chinese text documents. However, Chinese characters have characteristics in large numbers, complex structures, and diverse fonts, which limit the generation of glyph perturbation and make the design of Chinese characters time-consuming and laborious. Many font style transfer methods for Chinese characters have been proposed to improve the efficiency of Chinese character generation based on deep learning. At present, there are few studies on efficient font style transfer for glyph perturbation of Chinese characters. In this paper, a stylized glyph perturbation method based on style extractor and attention augmented convolution is proposed. It adopts a multi-head attention mechanism to enhance convolution in the font transfer, which concatenates the convolution feature maps and the self-attention activation maps to weaken the limitations of ordinary convolution in processing images. The extracted style features are sent into the decoder of the font transfer network so as to improve the stylized ability. Particularly, the impact of style extractor and attention augmented convolution on the glyph perturbation generation is addressed. The extraction accuracy and embedding capacity are tested in our experiments. The embedding capacity of secret message can achieve around 1.8 bit/character.
MoreTranslated text
Key words
Feature extraction,Perturbation methods,Convolution,Character generation,Task analysis,Skeleton,Optimization,Chinese character generation,glyph perturbation,information hiding,font style transfer,attention augmented convolution
AI Read Science
Must-Reading Tree
Example
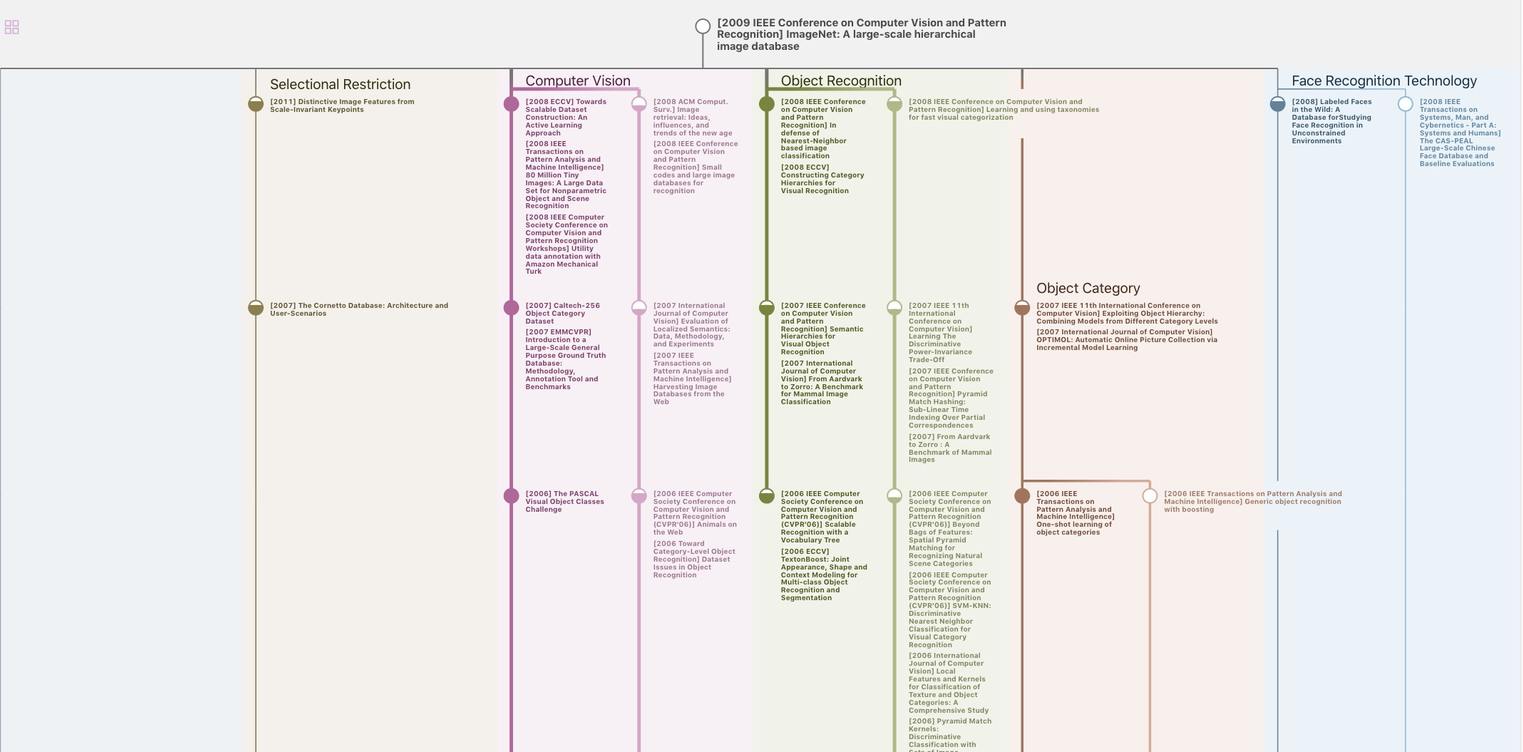
Generate MRT to find the research sequence of this paper
Chat Paper
Summary is being generated by the instructions you defined