Revolutionizing flame detection: Novelization in flame detection through transferring distillation for knowledge to pruned model
EXPERT SYSTEMS WITH APPLICATIONS(2024)
摘要
Traditional flame sensors have demonstrated suboptimal detection performance in complex environments, prompting researchers to integrate deep neural network algorithms into these sensors to enhance detection accuracy. However, these algorithms usually rely on sizeable neural networks, resulting in excessive parameterization, which limits their adaptability to different devices. To effectively compress models, this study proposes an effective flame detection framework where the distillation for knowledge is transferred to pruned model (DK-TPM) for the purpose of reducing model parameters. This framework integrates CFasterNet (CFN) and RepConv as foundational components within the base model called YO-CR to accomplish comprehensive feature learning while ensuring better compatibility with devices. Subsequently, structured pruning is applied to the base model to construct a student model. Finally, a new technique called feature distillation with smoothing (FDS) is employed to transfer flame features from the teacher model into the student model. We conducted experiments using three V100 GPUs on five public datasets, demonstrating the generalization capability of the algorithm. Moreover, systematic experiments on flame datasets yielded significant results, achieving an average precision (AP0.5) of 81.5% and frames per second (FPSGPU) of 1048.6, surpassing the requirements of existing devices. Therefore, the proposed DK-TPM is able to provide reliable prediction accuracy and speed for flame detection tasks.
更多查看译文
关键词
Fire safety,Neural network,Pruning,Knowledge distillation
AI 理解论文
溯源树
样例
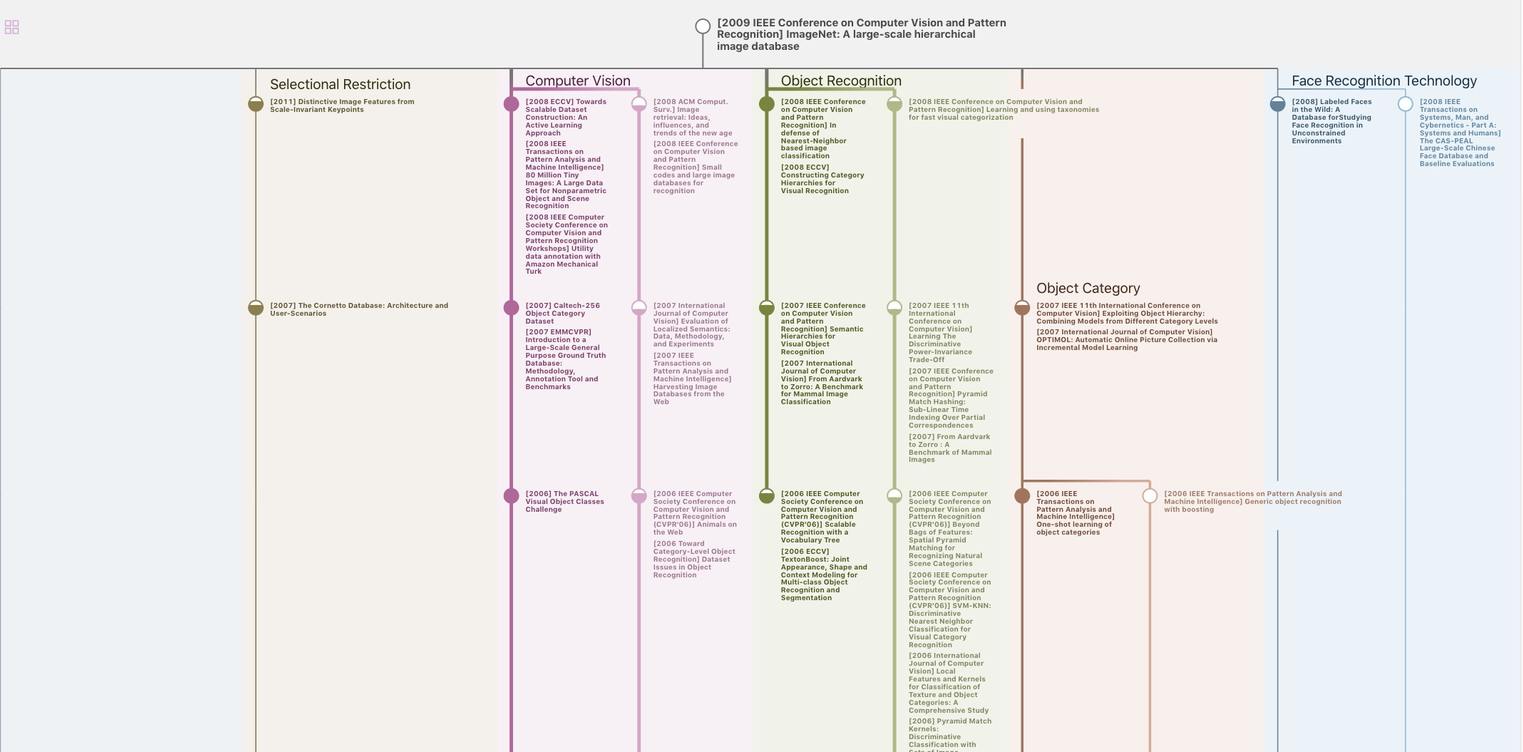
生成溯源树,研究论文发展脉络
Chat Paper
正在生成论文摘要