Learning, Tiny and Huge: Heterogeneous Model Augmentation Towards Federated Tiny Learning.
International Conference on Machine Learning and Applications(2023)
摘要
With the popularity of tiny devices based on microcontroller units, there is an urgent need to develop federated tiny learning to privately obtain a well-performed tiny model serving tiny devices. However, due to the limited capacity of tiny models, the fundamental difference between training deep neural networks and tiny neural networks makes existing federated learning designed for deep models ineffective in learning tiny models. Although prior tiny machine learning research successfully augments tiny models with enlarged architecture for improved capacity, such augmentation relies on a pre-known centralized dataset and thus cannot be used in federated settings. To fill this void, in this work, we propose an innovative federated tiny learning framework, FedTinyAug, to enable distributed tiny model augmentation. By taking advantage of the extra capability at larger participating devices, the server first constructs augmented models and distributes them to larger devices, providing auxiliary supervision for training the tiny model. To provide strong supervision, a gradient-based augmented model selection algorithm is designed to efficiently determine favorable augmented models to fully explore distinct or even heterogeneous on-device knowledge. Extensive experiments are conducted on three popular tiny models to validate the effectiveness of FedTinyAug. Key augmentation factors are evaluated to guide the implementation of FedTinyAug in practice.
更多查看译文
关键词
Federated Learning,Tiny Machine Learning
AI 理解论文
溯源树
样例
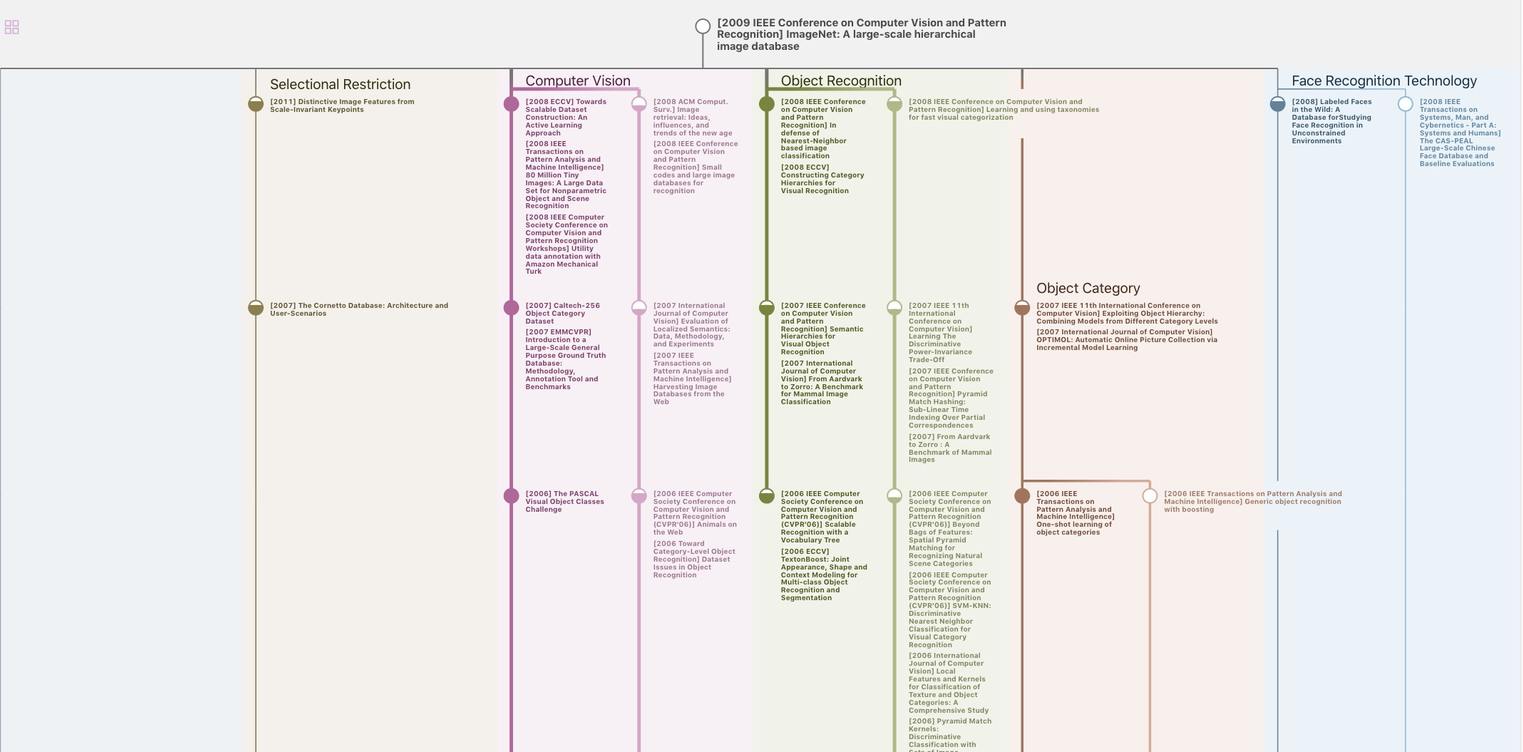
生成溯源树,研究论文发展脉络
Chat Paper
正在生成论文摘要