A Robust Recognition and Segmentation Algorithm for Retaining Walls in Mining Scenes
2023 China Automation Congress (CAC)(2023)
Abstract
The key for bulldozers to realize automatic operation in mine scenes is whether they can accurately identify and accurately segment the retaining walls, however, because the point cloud dataset of the mine site is too few, resulting in the deep learning-based identification and segmentation algorithm cannot be applied in this scene. At the same time, because of the rugged road surface of the mine scene, the existence of a lot of dust, numerous disturbances, retaining wall features are not obvious and other problems, the algorithms proposed by previous scholars are not perfect enough to solve the problem. We propose a point cloud recognition and segmentation algorithm based on clustering and evaluation function of integrated features. Ours firstly compensates the skew of the point cloud map with RANSAC and down samples the data by gridding the point cloud. Then reduces the influence of dust and truck materials in the scene by normal vector and variance information. Finally, screens out the candidate target class by density clustering, and identifies and segments the retaining wall by integrated feature. Our proposed algorithm is validated in several different real mine scenarios and the results show that ours has high accuracy and strong robustness.
MoreTranslated text
Key words
point cloud recognition,density clustering,evaluation function,RANSAC plane fitting
AI Read Science
Must-Reading Tree
Example
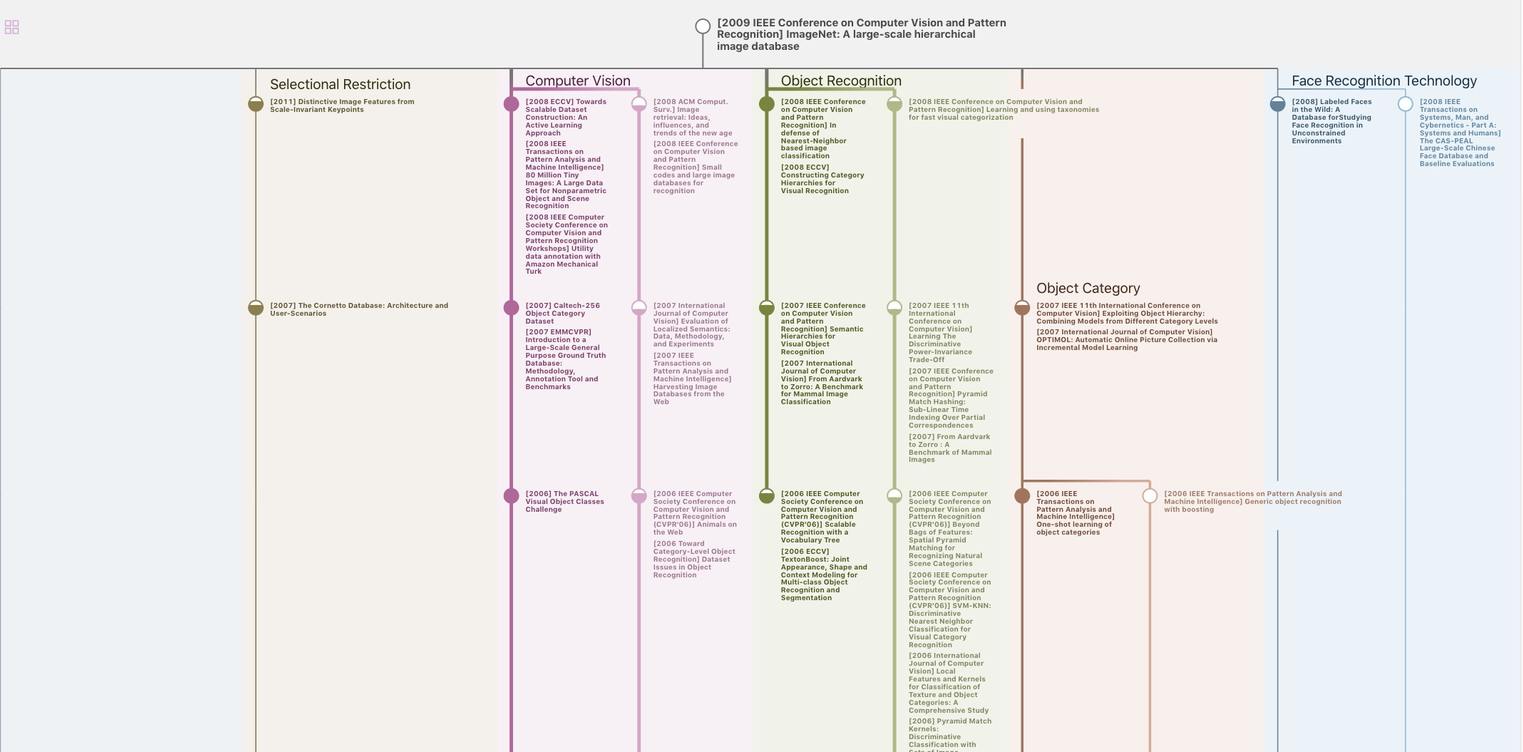
Generate MRT to find the research sequence of this paper
Chat Paper
Summary is being generated by the instructions you defined