Learning Spatial-Temporal Decoupling Representations for Few-Shot Action Recognition
2023 China Automation Congress (CAC)(2023)
摘要
Few-shot action recognition is the task of predicting unlabeled actions based on a limited number of labeled actions. Recently most of few-shot action recognition methods adopt GCNs to encode the skeleton clips and predict the label by calculating the distance in spatial-temporal feature space. However, coarse encoded features will lead to confusion between ambiguous actions with similar spatial or temporal information, which results in misclassification among these actions. To solve this problem, we propose a effective method called Spatial-Temporal Decoupling Matching (STDM) consisting of a spatial-temporal decoupling module and a spatial-temporal matching strategy to model the spatial and temporal information effectively. The spatial-temporal decoupling module maps confusing encoded features to spatial and temporal feature spaces respectively. The spatial-temporal matching strategy simultaneously recognizes the actions from the spatial and temporal perspective. We have conducted numerous experiments on NTU RGB+D 120 and Kinetics datasets. Our method achieves comparable results and surpasses most of the SOTA methods.
更多查看译文
关键词
graph convolutional network,spatial-temporal decoupling,skeleton-based action recognition
AI 理解论文
溯源树
样例
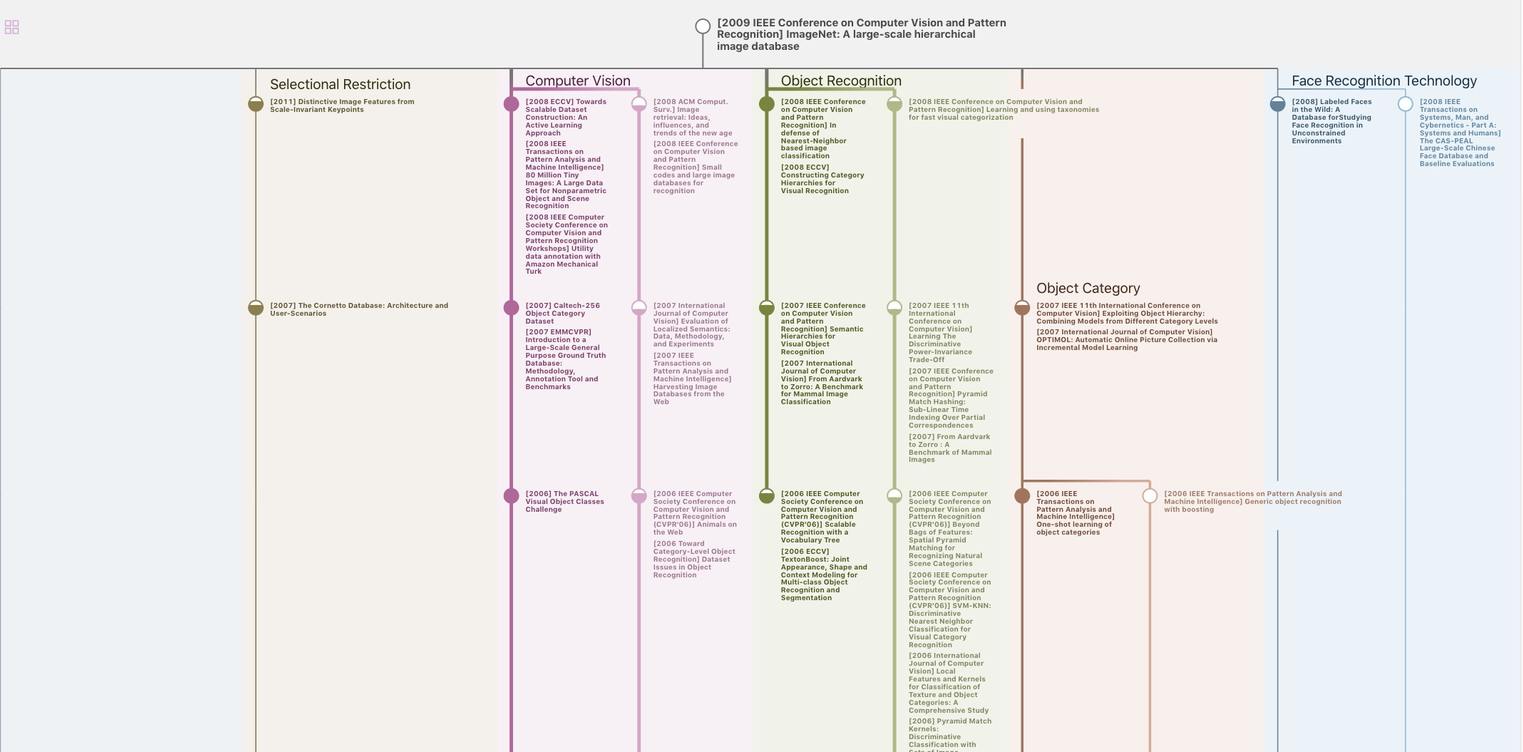
生成溯源树,研究论文发展脉络
Chat Paper
正在生成论文摘要