Optimizing Federated Learning in Statistical Heterogeneity: A Fire Detection Use Case
2024 IEEE APPLIED SENSING CONFERENCE, APSCON(2024)
Abstract
Sensors serve as the vigilant eyes and ears of the modern world, seamlessly collecting and translating environmental cues into invaluable data. The collected data enables us to make precise predictions for related events, which relies on data-trained models. These data contain critical and sensitive information, which requires to gathered on central server for model training in traditional ML approach, raising the concern of data privacy. Therefore, preserving the data privacy during model training is crucial. Federated Learning (FL) emerges as a solution that provides privacy-preserving model training without sharing the data. However, it has been observed that statistical heterogeneity in FL leads to drifts among the local models, causing unsatisfactory FL performance. To address this, the work proposes using the 'SARAH optimizer' with the FedAvg algorithm to minimize the drift by reducing the variance of the local updates. The results of this proposed implementation have shown significant performance improvements and a better convergence rate. Additionally, the work provides a fire detection use case for the proposed approach.
MoreTranslated text
Key words
Federated Learning,Statistical Heterogeneity,SARAH,Fire Detection
AI Read Science
Must-Reading Tree
Example
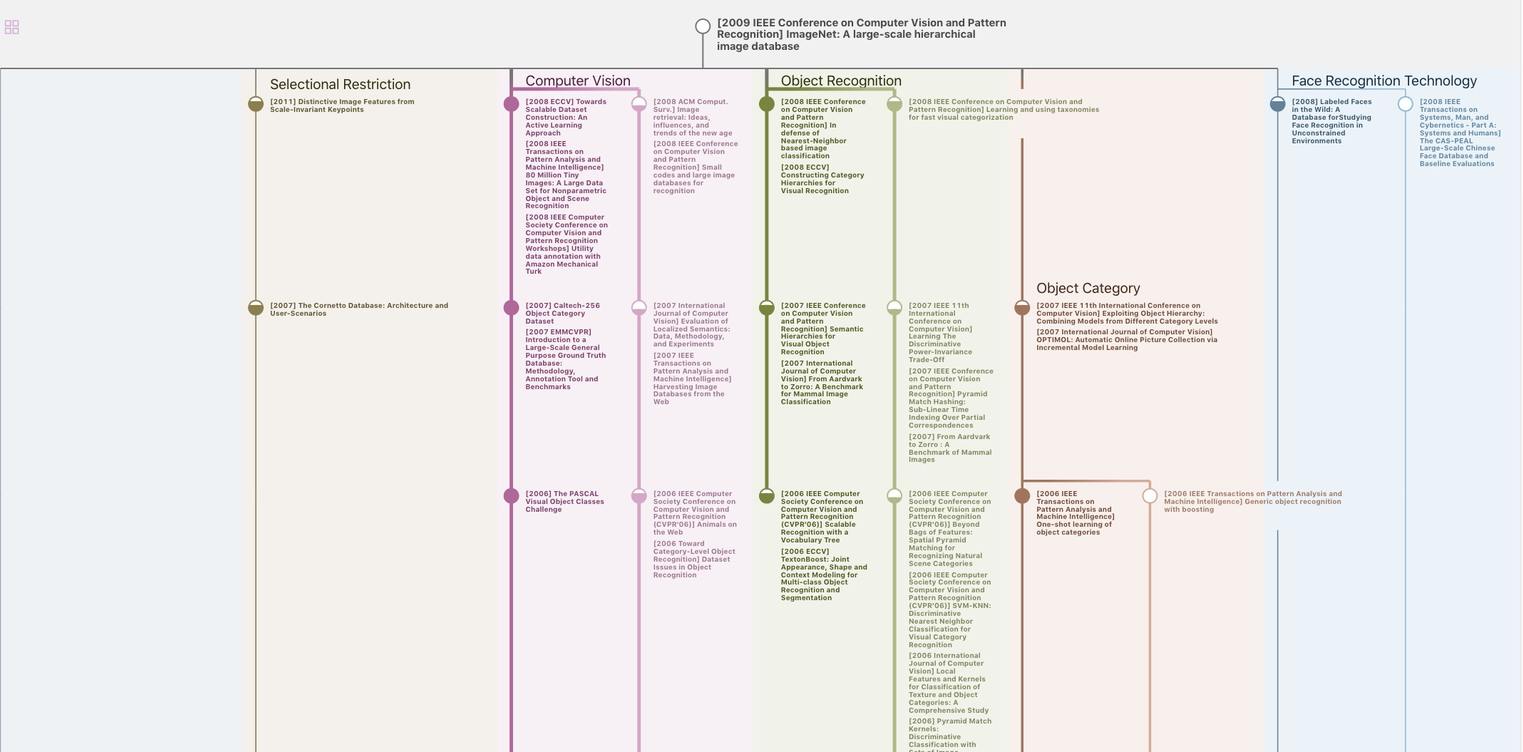
Generate MRT to find the research sequence of this paper
Chat Paper
Summary is being generated by the instructions you defined