W3Detector: Detecting Fraudulent Online Sellers Based on Temporal and Spacial Information.
International Conference on Machine Learning and Applications(2023)
摘要
With the rapid development of electronic payment, risks in large-scale financial transaction networks are persistently arising. One important problem is how to use the transaction data to detect sellers who do fraudulent trades on the online platform. This paper analyzes three attributes of the transaction flow: money (what), time (when), region (where), and summarizes corresponding characteristics among fraudulent sellers. Based on this, we propose an unsupervised anomaly detection algorithm: W3Detector. It processes the raw data with a new sequence discretization method and uses statistical tools to convert data indicators into information value. With the minimum description length principle, we finally filter out the suspicious sellers. The algorithm is not affected by factors such as unit or range of the attributes, and is highly flexible and scalable. After running on three real-world transaction datasets collected from WeChat Pay, W3Detector has show significantly better performance than the baseline methods of anomaly detection, with weighted accuraccy (WACC) increased by 1.13X and F-score increased by 0.105 on average, and the recall value over 60%.
更多查看译文
关键词
anomaly detection,fraudulent trade,unsupervised algorithm,e-commerce business
AI 理解论文
溯源树
样例
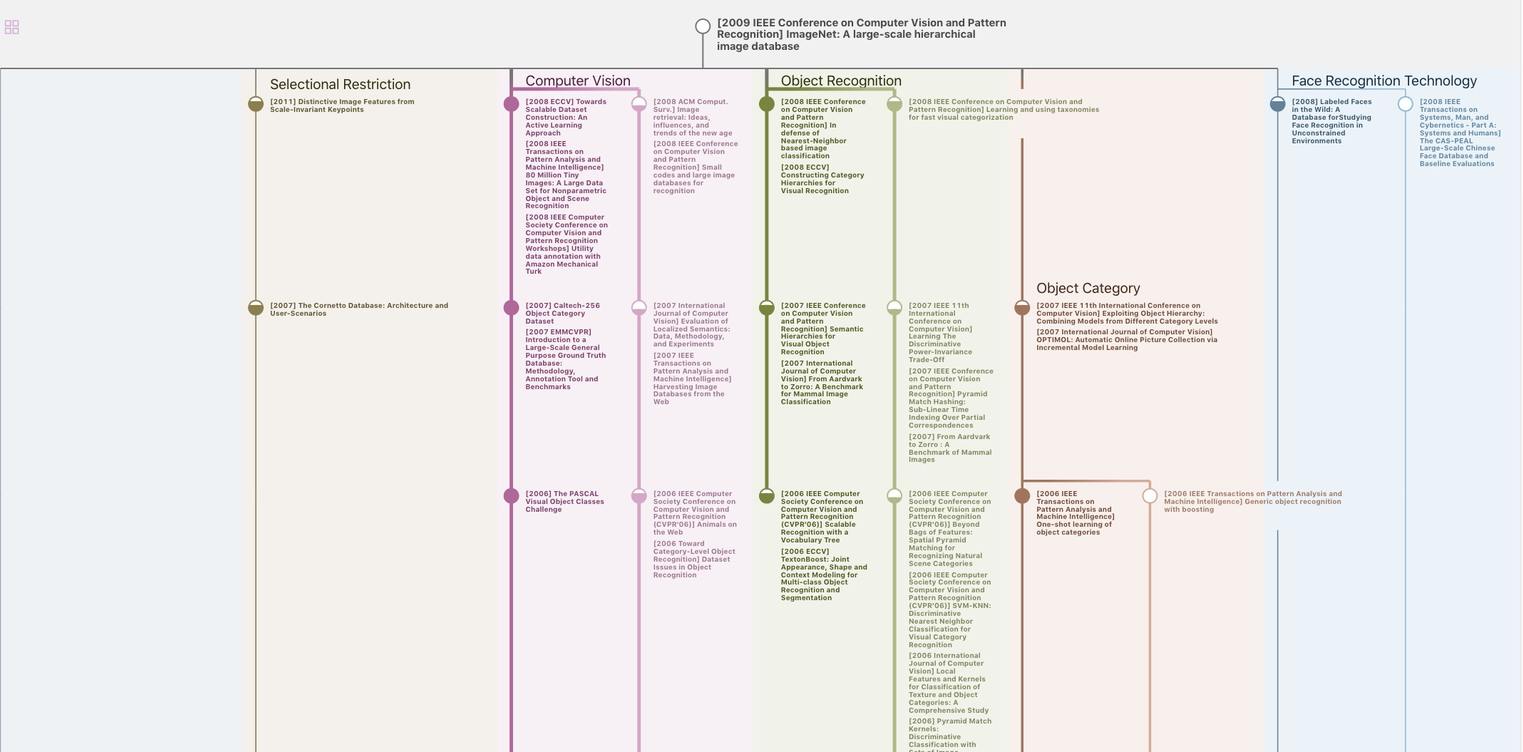
生成溯源树,研究论文发展脉络
Chat Paper
正在生成论文摘要