Multimodal Co-Learning Meets Remote Sensing: Taxonomy, State of the Art, and Future Works
IEEE Journal of Selected Topics in Applied Earth Observations and Remote Sensing(2024)
摘要
In remote sensing (RS), multiple modalities of data are usually available, e.g., RGB, Multispectral, Hyperspectral, LiDAR, and SAR. Multimodal machine learning systems, which fuse these rich multimodal data modalities, have shown better performance compared to unimodal systems. Most multimodal research assumes that all modalities are present, aligned, and noiseless during training and testing time. However, in real-world scenarios, it is common to observe that one or more modalities are missing, noisy, and non-aligned, in either training or testing or both. In addition, acquiring large-scale, noise-free annotations is expensive, as a result, lacking sufficient annotated datasets or having to deal with inconsistent labels are open challenges. These challenges can be addressed under a learning paradigm called multimodal co-learning. This paper focuses on multimodal co-learning techniques for remote sensing data. We first review what data modalities are available in the remote sensing domain and the key benefits and challenges of combining multimodal data in the remote sensing context. We then review the remote sensing tasks that would benefit from multimodal processing including classification, segmentation, target detection, anomaly detection, and temporal change detection. We then dive deeper into technical details by reviewing more than 200 recent efforts in this area and provide a comprehensive taxonomy to systematically review state-of-the-art approaches in 4 key co-learning challenges including missing modalities, noisy modalities, limited modality annotations, and weakly-paired modalities. Based on these insights, we propose emerging research directions to inform potential future research in multimodal co-learning for remote sensing.
更多查看译文
关键词
Multimodal co-learning,multimodal learning,remote sensing,satellite imagery
AI 理解论文
溯源树
样例
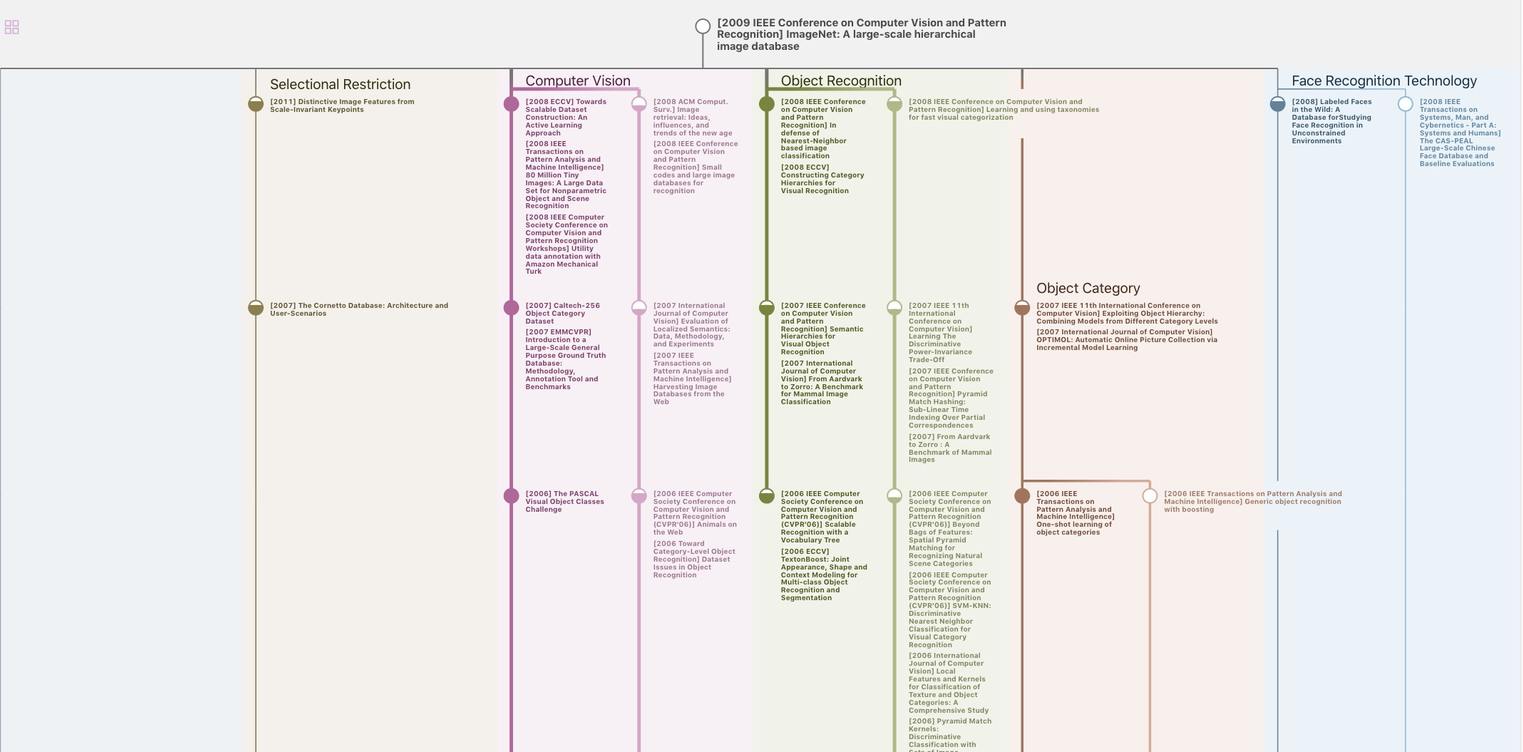
生成溯源树,研究论文发展脉络
Chat Paper
正在生成论文摘要