Unsupervised End-to-End Training with a Self-Defined Bio-Inspired Target
arxiv(2024)
摘要
Current unsupervised learning methods depend on end-to-end training via deep
learning techniques such as self-supervised learning, with high computational
requirements, or employ layer-by-layer training using bio-inspired approaches
like Hebbian learning, using local learning rules incompatible with supervised
learning. Both approaches are problematic for edge AI hardware that relies on
sparse computational resources and would strongly benefit from alternating
between unsupervised and supervised learning phases - thus leveraging widely
available unlabeled data from the environment as well as labeled training
datasets. To solve this challenge, in this work, we introduce a 'self-defined
target' that uses Winner-Take-All (WTA) selectivity at the network's final
layer, complemented by regularization through biologically inspired homeostasis
mechanism. This approach, framework-agnostic and compatible with both global
(Backpropagation) and local (Equilibrium propagation) learning rules, achieves
a 97.6
incorporating a hidden layer enhances classification accuracy and the quality
of learned features across all training methods, showcasing the advantages of
end-to-end unsupervised training. Extending to semi-supervised learning, our
method dynamically adjusts the target according to data availability, reaching
a 96.6
our 'unsupervised target' strategy's efficacy and flexibility in scenarios
ranging from abundant to no labeled data availability.
更多查看译文
AI 理解论文
溯源树
样例
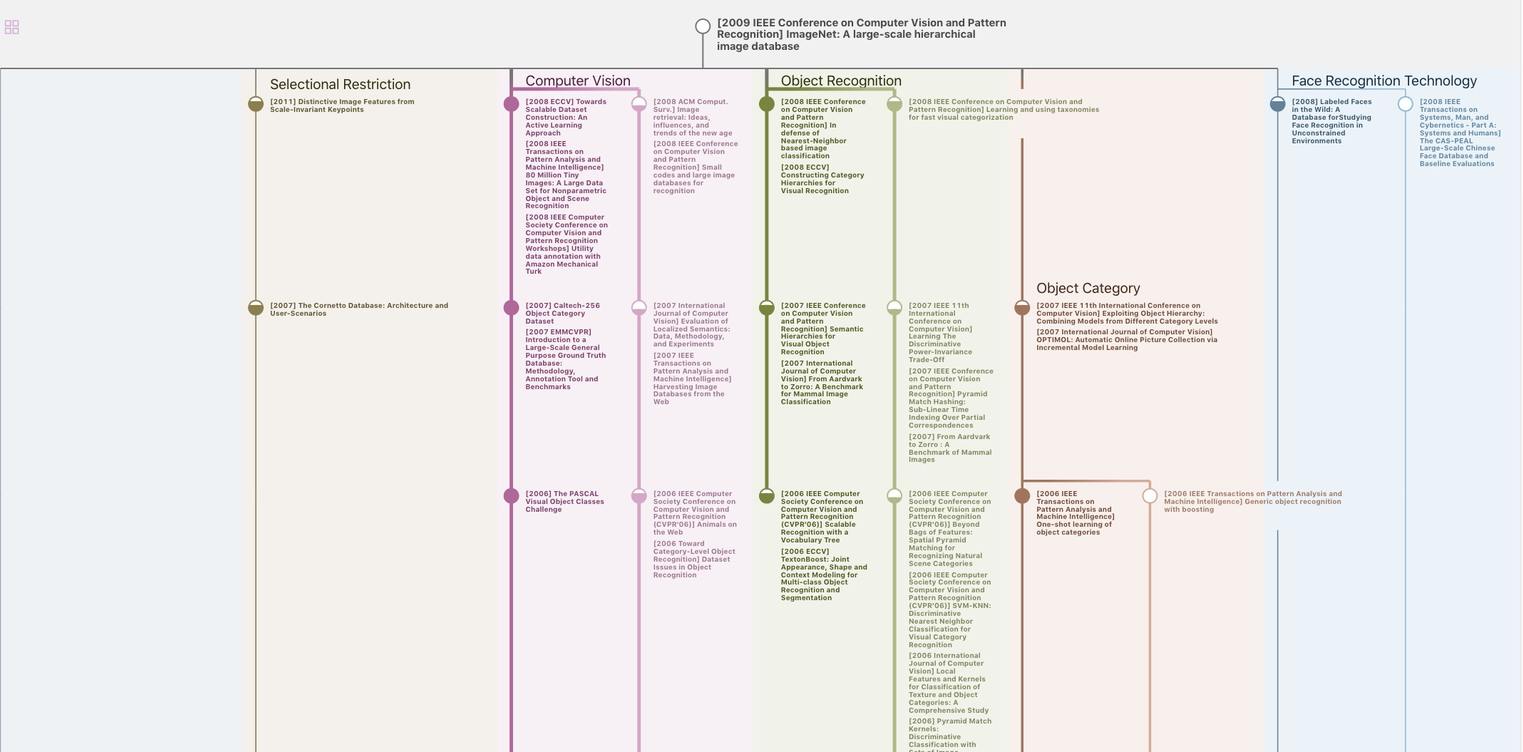
生成溯源树,研究论文发展脉络
Chat Paper
正在生成论文摘要