DOWN: Dynamic Order Weighted Network for Fine-grained Category Discovery
Knowledge-Based Systems(2024)
摘要
Discovering fine-grained categories from coarsely-labeled data is a practical and challenging task, which can adapt models to fine-grained sub-categories and reduce significant annotation costs. In the absence of fine-grained labels, previous works have encountered challenges in modeling fine-grained semantic correlation within data effectively and efficiently. In response to this challenge, we propose DOWN: Dynamic Order Weighted Network, which systematically explores and exploits semantic structures within data and reduces the effect of noisy samples. Specifically, a multi-task pre-training paradigm is initially employed to acquire both general and coarse-grained knowledge. Subsequently, we retrieve a candidate neighbor set for a given query and then sort the retrieved neighbors based on semantic similarity to capture relative orders between data, which enhances model capability to distinguish false-positive neighbors. To optimize the utilization of semantic structures of data and mitigate the impact of false neighbors, we introduce a weighting mechanism with exponential decay functions for the sorted neighbors based on order distances. Considering the incremental growth of the model’s capability during training, we dynamically adjust the base of the exponential decay function, which progressively augments the weights for tail samples, thus achieving equilibrium between exploitation of neighborhood information and avoidance of neighborhood noise. Moreover, we theoretically justify the effectiveness of our model from three perspectives. Extensive experiments on three benchmark datasets validate both effectiveness and efficiency of our model over SOTA models. Code and data will be released.
更多查看译文
关键词
Fine-grained Category Discovery,Weakly-supervised Learning,Neighborhood Contrastive Learning
AI 理解论文
溯源树
样例
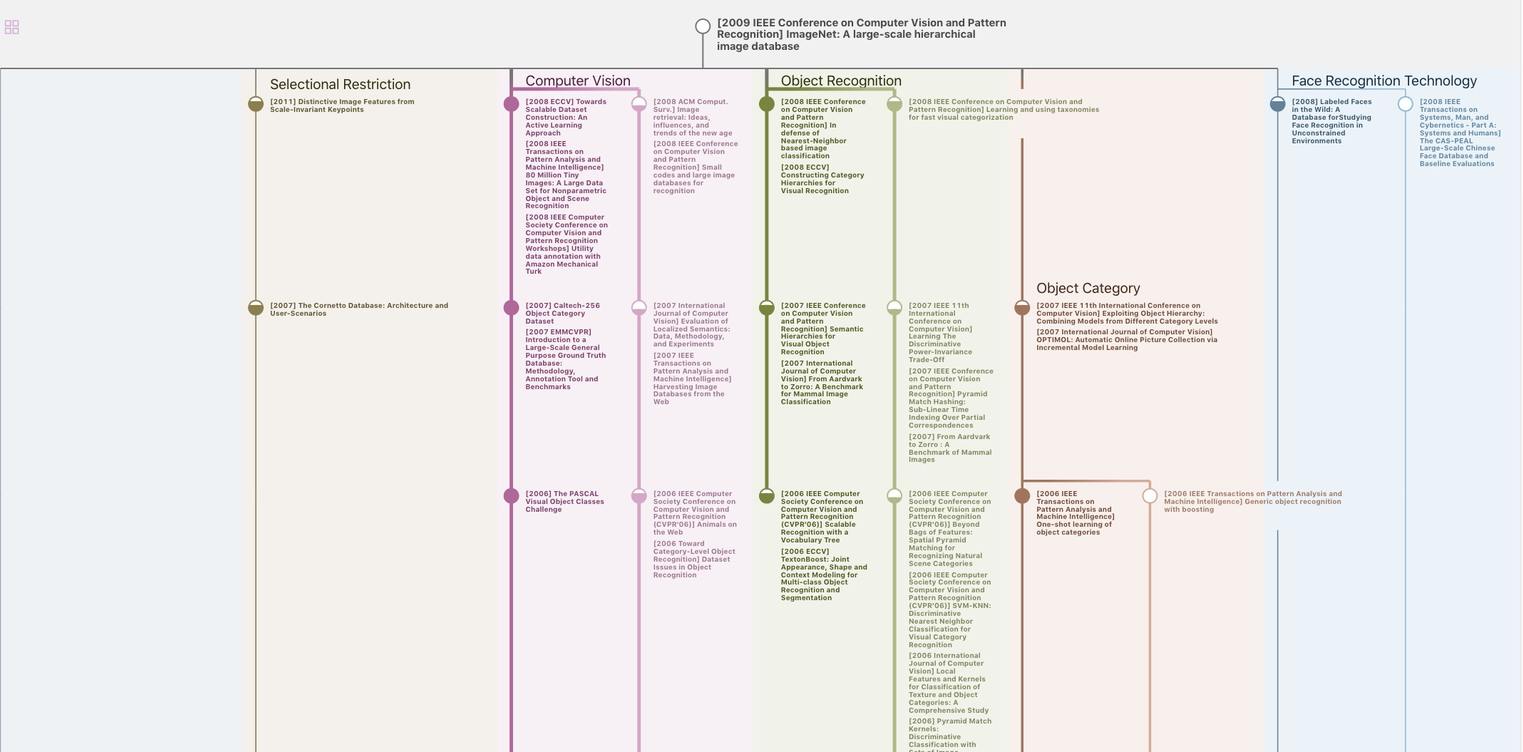
生成溯源树,研究论文发展脉络
Chat Paper
正在生成论文摘要