Defect Detection in the Manufacturing Domain Using Product Design Data and Self-Knowledge Distillation.
International Conference on Electronics, Information and Communications(2024)
Abstract
The endeavor of defect detection in manufacturing is critical for ensuring product quality. Recent advancements have notably shifted towards the application of deep learning techniques to tackle this crucial task. However, this domain struggles with complexities due to the presence of noise and variability in the data collected. A significant obstacle arises from the imbalanced nature of datasets, where instances of defects are rare compared to abundant non-defective samples. Traditional data augmentation strategies, while commonplace, may unintentionally obscure defect characteristics, com-promising detection efficacy. In this paper, we propose a powerful strategy that utilizes invariant features and Self-Knowledge Distillation (Self-KD) as regularization method. Our method leverages existing image data alongside invariant features extracted from Computer Aided Design (CAD) to enhance the precision of defect classification. Additionally, we introduce the application of Self-KD as a potent regularization strategy in scenarios with limited defective instances. By enforcing consistency among points of the same class, our model effectively prevents overconfident predictions and reduces intra-class variations. Our experimental results demonstrate that our methodology substantially increases detection accuracy in datasets sourced from authentic industrial settings. Numerically, the performance of defect detection improved by 1.8%.
MoreTranslated text
Key words
Manufacturing industry,vision inspection system,defect detection,self-knowledge distillation
AI Read Science
Must-Reading Tree
Example
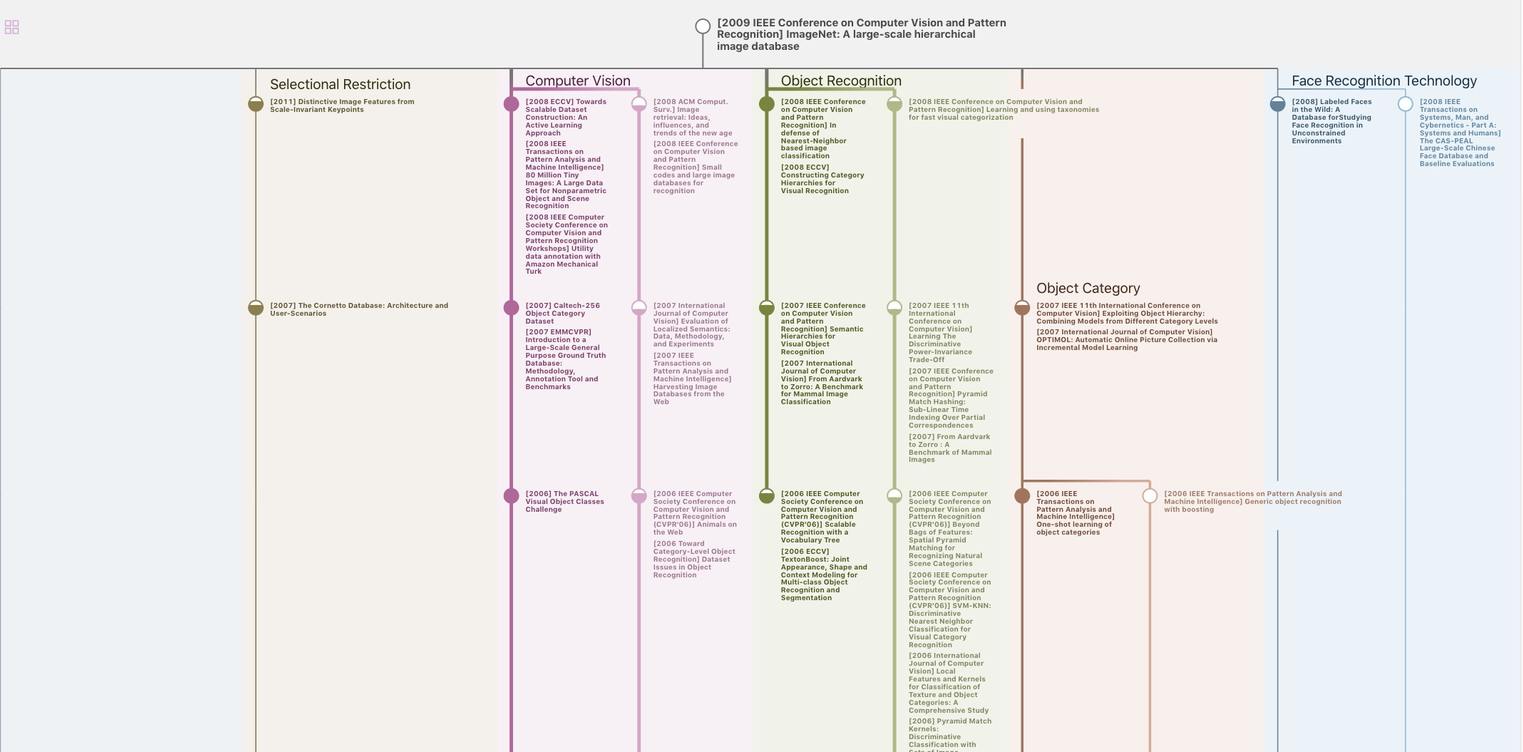
Generate MRT to find the research sequence of this paper
Chat Paper
Summary is being generated by the instructions you defined